PnP-Based Ground Moving Target Imaging Network for Squint SAR and Sparse Sampling
IEEE TRANSACTIONS ON GEOSCIENCE AND REMOTE SENSING(2024)
摘要
As a result of residual phase error caused by motion parameters, ground moving targets (GMTs) are defocused and displaced in conventional synthetic aperture radar (SAR) imaging. Although some refocusing algorithms for GMT have been proposed, these methods are difficult to handle geometric correction and sparse recovery in squint mode simultaneously. Deep learning (DL) technology has been successfully used to solve radar imaging problems in microwave vision, where deep unfolding networks (DUNs) and convolutional neural networks (CNNs) are the most widely applied. In this article, a novel GMT imaging network (GMTIm-Net) is proposed for squint SAR and sparse sampling, whose framework combines DUN and CNN advantages. Specifically, we first incorporate a matched filter-based approximated observation model and a minimum entropy-based motion parameter estimation method within a sparse reconstruction framework. An iterative shrinkage threshold algorithm is adopted to solve this framework, and the solution procedure is unfolded as a GMT refocusing network. Then, we introduce plug-and-play (PnP) technology to replace the & ell;(1) norm-based regularizer for improving its noise immunity. Finally, a CNN-based image transformation network is proposed to perform geometric correction of imaging results in squint mode. By inputting the 2-D sparse complex-valued GMT echo, the trained GMTIm-Net can efficiently output focused and corrected GMT images. The experiments demonstrate that our proposed GMTIm-Net outperforms conventional GMT focusing methods in terms of focusing performance and computing efficiency.
更多查看译文
关键词
Deep learning (DL),ground moving target imaging (GMTIm),plug-and-play (PnP),sparse reconstruction,synthetic aperture radar (SAR)
AI 理解论文
溯源树
样例
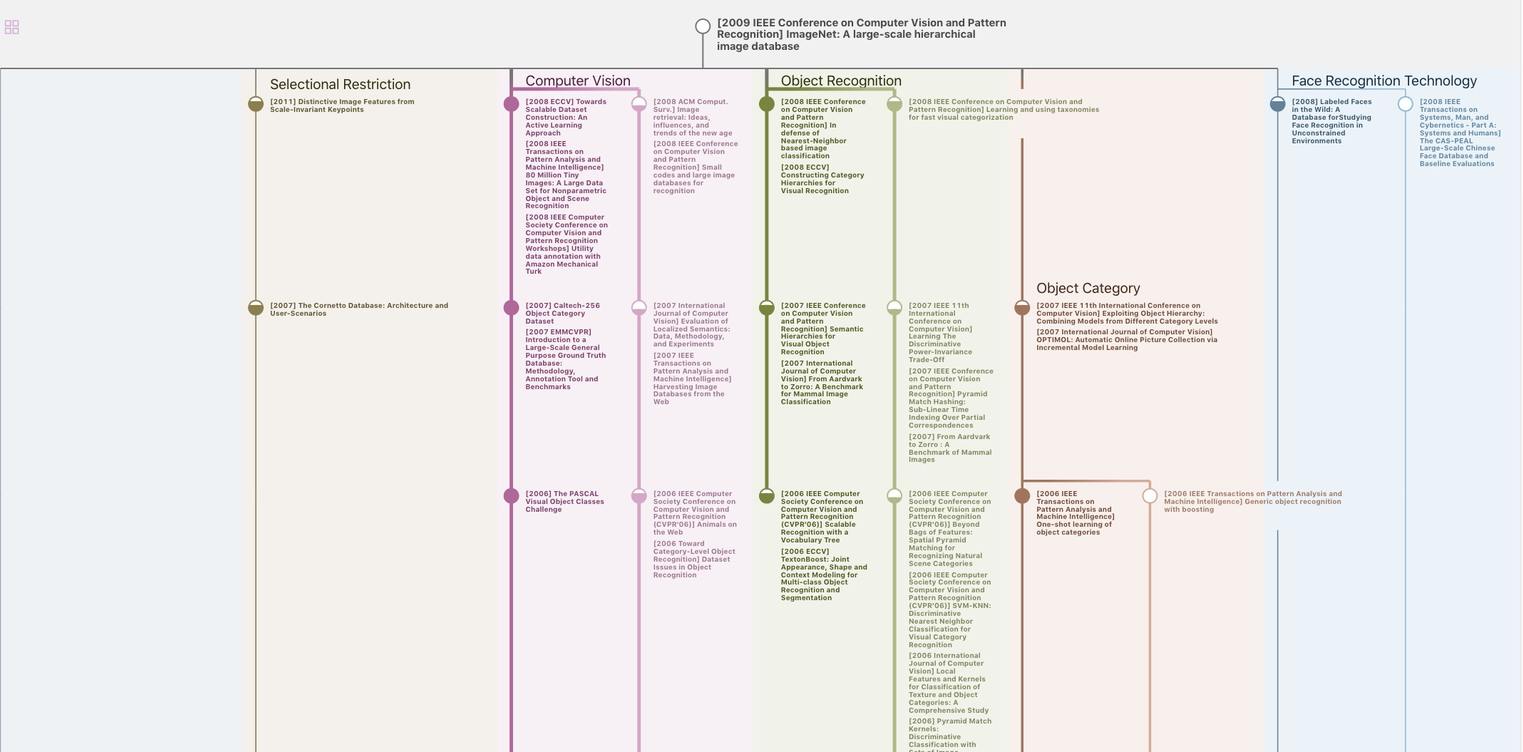
生成溯源树,研究论文发展脉络
Chat Paper
正在生成论文摘要