Lightweight CNN Architecture Design Based on SpatialTemporal Tensor and Its Application in Bearing Fault Diagnosis
IEEE Transactions on Instrumentation and Measurement(2024)
摘要
Bearing is a failure-prone component in rotating machinery equipment, and various task conditions make bearing fault diagnosis (BFD) a challenging task. Despite the great success of convolutional neural network (CNN) in feature extraction, most of them are based on manual tuning, and it is not easy to determine a unified CNN architecture with an effective balance between accuracy and computational resources [parameters and floating point operations (FLOPs)]. In this article, an automatic CNN architecture design method called AUTO-CNN is proposed to address the above issues. First, a novel representation learning method (RLM) based on spatial-temporal tensors (STTs) is proposed, in which AUTO-CNN can use 2-D multiscale convolutions to capture more feature hierarchy, i.e., temporal variabilities and spatial characteristics. Second, unlike most of the current nontask-specific studies that establish deeper and more complex networks for better performance, this article investigates lightweight CNNs with limited resources under task-specific applications. Finally, to speed up the design process, an efficient hierarchical encoding strategy and space exploration strategy are proposed to avoid inefficient architecture generation and reduce CNNs training time. Experiments on four real-world BFD tasks demonstrate that the proposed method outperforms the peer competitors, including machine learning, deep learning, and other CNN-based methods.
更多查看译文
关键词
Automatic architecture design,bearing fault diagnosis (BFD),convolutional neural network (CNN),deep learning,genetic algorithm
AI 理解论文
溯源树
样例
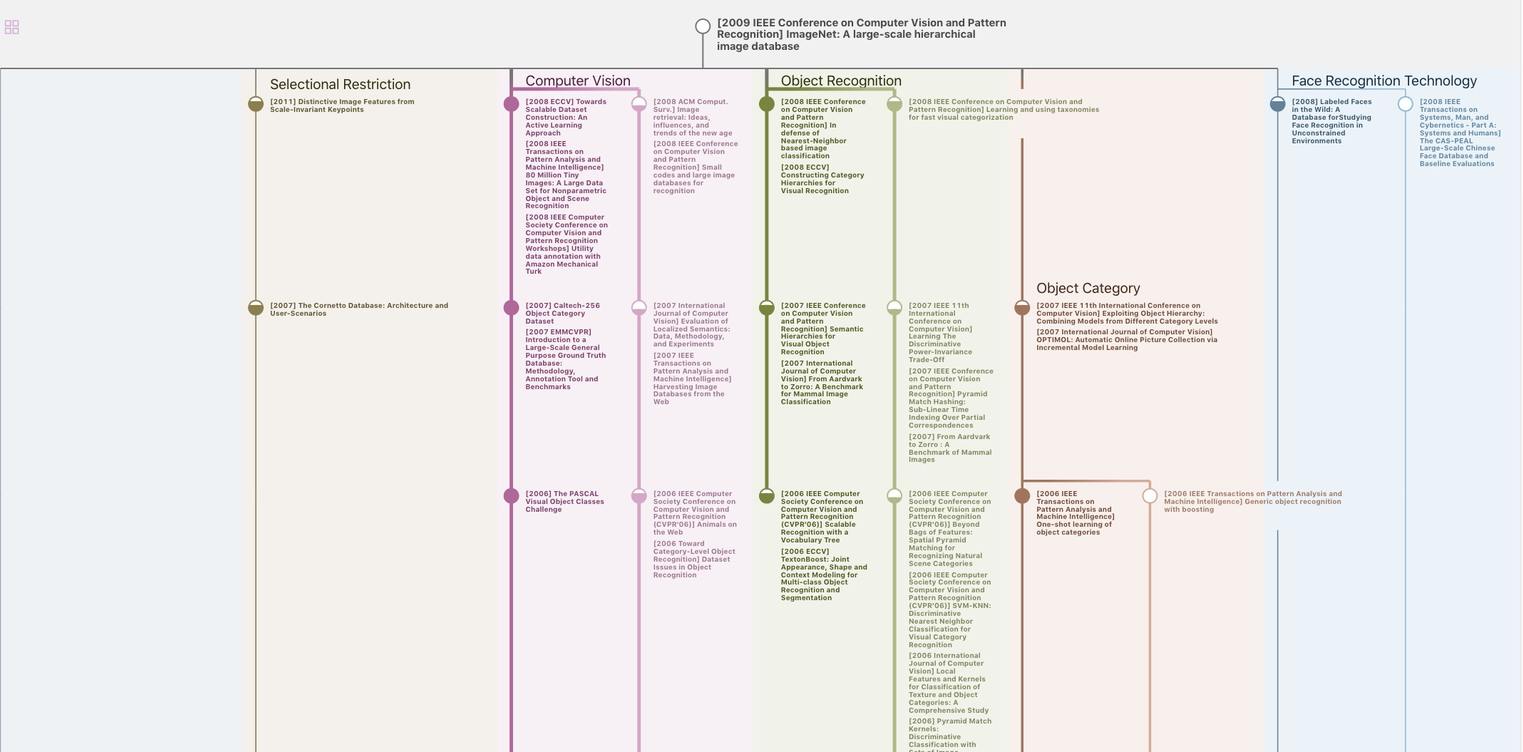
生成溯源树,研究论文发展脉络
Chat Paper
正在生成论文摘要