Temporal Double Graph Convolutional Network for CO and CO Prediction in Blast Furnace Gas.
IEEE Transactions on Instrumentation and Measurement(2024)
Abstract
Accurately predicting CO and CO2 content in blast furnace gas (BFG) holds immense significance, ensuring stable furnace operation and improving energy utilization. However, due to the variable operating conditions of blast furnace (BF) ironmaking and complex chemical reactions in the BF, it is difficult to accurately predict the changing trend of CO and CO2 content in BFG. To solve this problem, this study proposes a temporal double graph convolutional network (TDGCN) model for CO and CO2 content prediction. It consists of three parts: graph convolution, hypergraph convolution, and TimesNet. Specifically, we constructed a BF ironmaking feature graph in the face of the complex coupling relationship between BF ironmaking features. The graph convolutional network (GCN) is used to extract the topology on the feature graph and to update the feature variables. To further extract feature correlations and relevant information, we employ a hypergraph convolutional network to explore high-order correlations within the hypergraph. Subsequently, we utilize this information to update the feature graph, endowing the TDGCN model with dynamic adaptive capabilities under varying operating conditions. Finally, we introduce TimesNet to model long-term dependencies in BF ironmaking data. Through a series of experiments, the results show that the prediction effect of the TDGCN model is better than that of the traditional methods.
MoreTranslated text
Key words
Blast furnace gas (BFG),CO and CO₂ content,graph convolutional network (GCN),hypergraph convolutional network,prediction
AI Read Science
Must-Reading Tree
Example
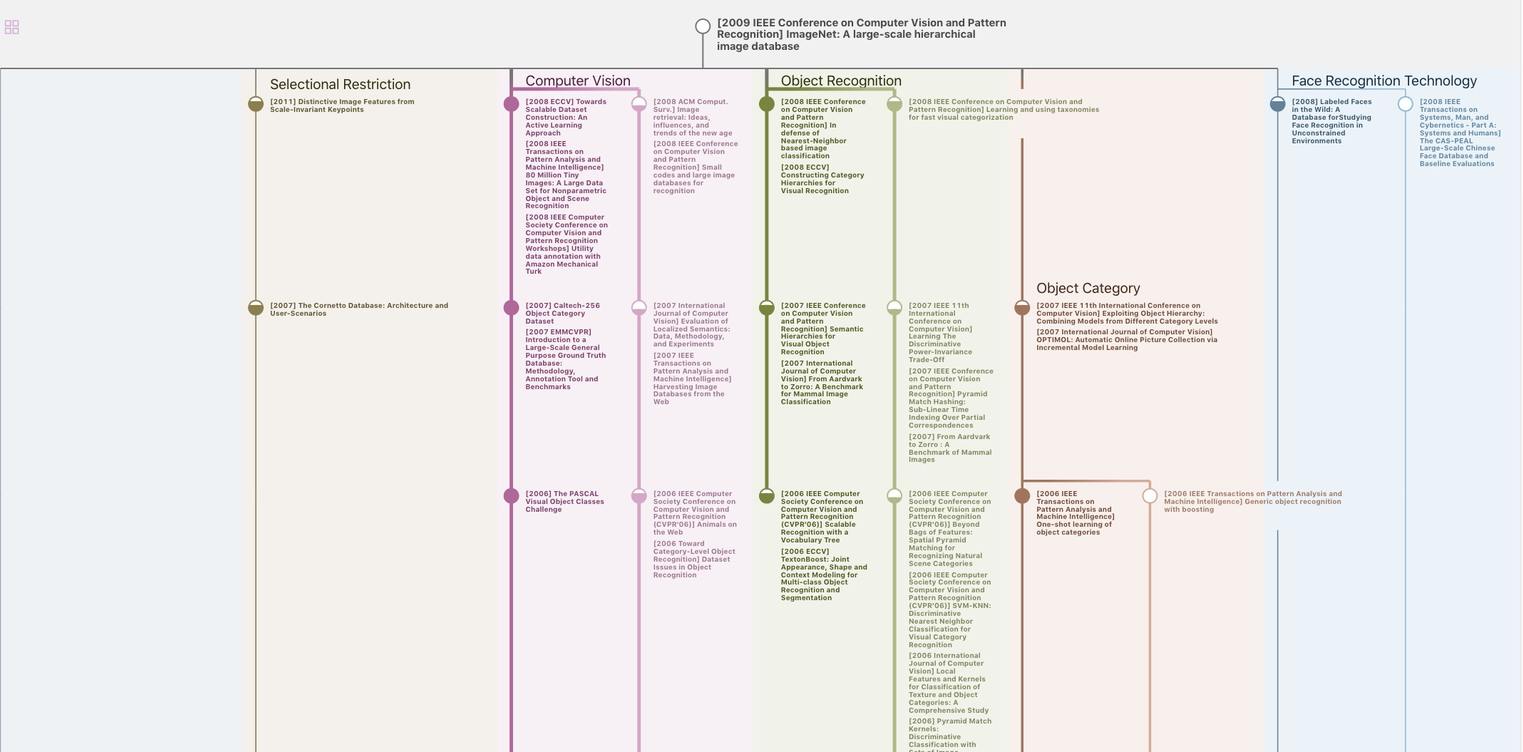
Generate MRT to find the research sequence of this paper
Chat Paper
Summary is being generated by the instructions you defined