PSNN-TADA: Prototype and Stochastic Neural Network-Based Twice Adversarial Domain Adaptation for Fault Diagnosis Under Varying Working Conditions
IEEE TRANSACTIONS ON INSTRUMENTATION AND MEASUREMENT(2024)
摘要
The varying working conditions of rolling bearings lead to data distribution shifts, which presents an obstacle to intelligent fault diagnosis. To alleviate the performance degradation, unsupervised domain adaptation (DA) is used to address the issue where the test set has a different distribution from the training set. Most existing intelligent transfer fault diagnosis methods align features by using either a fixed metric or a domain discriminator to tackle data distribution divergence. However, the alignment of decision boundaries in fault classifiers has not been thoroughly studied, causing ambiguous predictions on unlabeled target domain samples and catastrophic performance degradation in fault diagnosis. To overcome the deficiencies, a novel diagnosis framework named prototype and stochastic neural network-based twice adversarial DA (PSNN-TADA) is proposed to extract domain-invariant fault features under varying working conditions. In particular, PSNN-TADA has two adversarial training processes at different levels. First, PSNN-TADA has a prototype-based discriminator using multimodal information to achieve conditional domain alignment. Second, PSNN-TADA learns reliable decision boundaries from an adversarial process between the extractor and the stochastic classifier, which further improves the accuracy of the classifier on target fault samples. The proposed method is comprehensively tested on both an open-access dataset and a practical fault diagnosis platform, and extensive experimental results illustrate that PSNN-TADA achieves superior adaptation performance compared with the state-of-the-art.
更多查看译文
关键词
Conditional domain adversarial,intelligent fault diagnosis,stochastic classifier,unsupervised domain adaptation (DA),varying working conditions
AI 理解论文
溯源树
样例
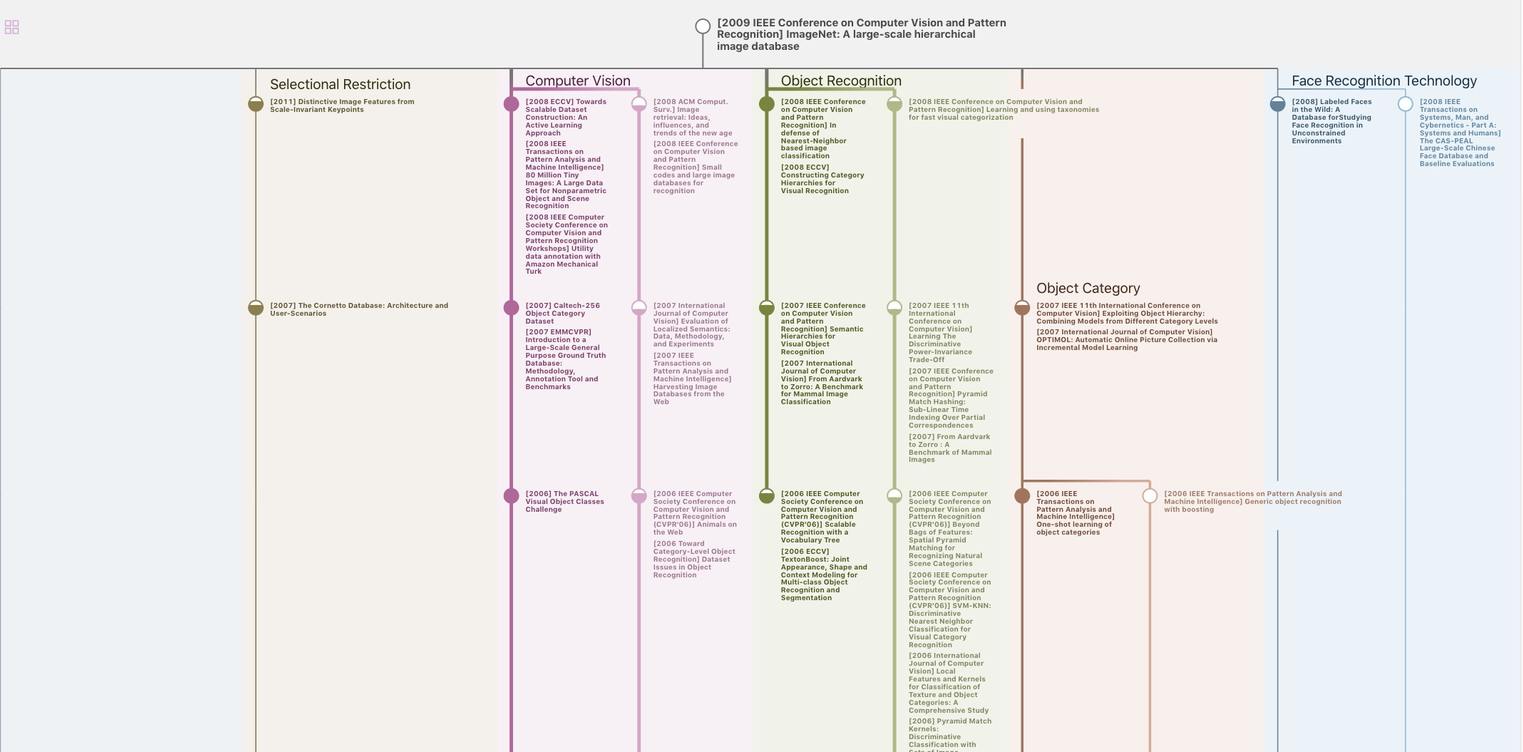
生成溯源树,研究论文发展脉络
Chat Paper
正在生成论文摘要