Fault Diagnosis of Highway Machinery Hydraulic System Based on LS-TF
IEEE TRANSACTIONS ON INSTRUMENTATION AND MEASUREMENT(2024)
Abstract
Fault diagnosis of hydraulic system is of great significance to reduce the risk of damage to road machinery and improve construction safety. The hydraulic system of highway machinery has the characteristics of uncertainty, nonlinearity, and time-varying, so it is difficult to carry out efficient fault diagnosis under complex working conditions. In this article, a new approach to classification based on a hybrid model of transformer and long short-term memory neural network (LSTF) is proposed. At first, the feature information of 1-D time series is extracted by the convolution layer and long short-term memory (LSTM) network, which reduces the problems, such as gradient disappearance. Second, the location feature information is obtained by location encoding in transformer, and the multihead attention mechanism (MHAM) as well as the residual network realize the capture of associated features in time series to facilitate the transfer of feature information in the network. Finally, the anti-noise experiments are conducted on the model in the dataset of the hydraulic system of road machinery, and the results of fault diagnosis are visualized. The analysis has shown that the proposed model has high diagnostic accuracy and noise resolution compared with other diagnostic methods.
MoreTranslated text
Key words
Anti-noise,hydraulic system,intelligent fault diagnosis,road machinery
AI Read Science
Must-Reading Tree
Example
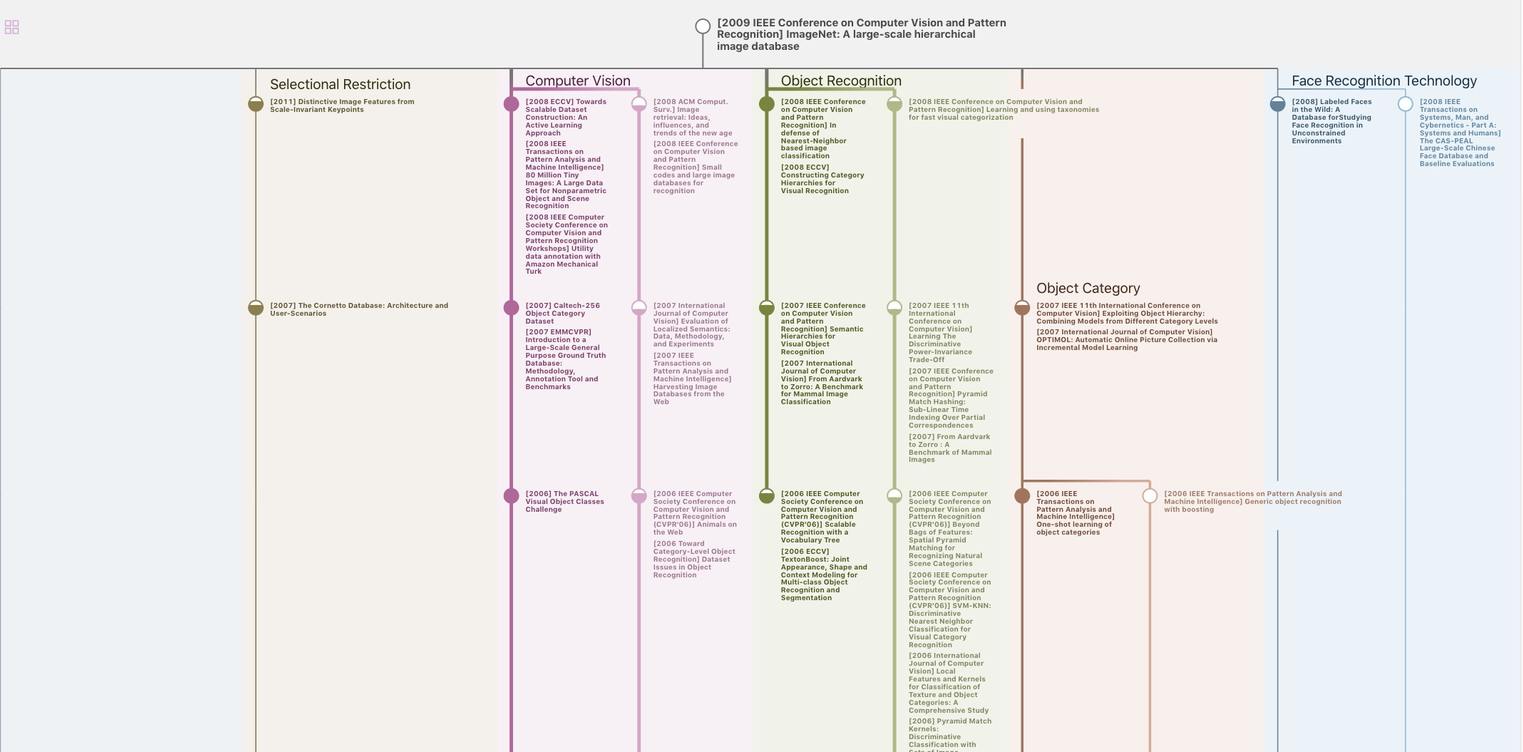
Generate MRT to find the research sequence of this paper
Chat Paper
Summary is being generated by the instructions you defined