Automated Identification and Quantification of Metastatic Brain Tumors and Perilesional Edema Based on a Deep Learning Neural Network.
JOURNAL OF NEURO-ONCOLOGY(2024)
Key words
Metastatic brain tumors,Perilesional edema,Deep learning neural network,Identification,Quantification
AI Read Science
Must-Reading Tree
Example
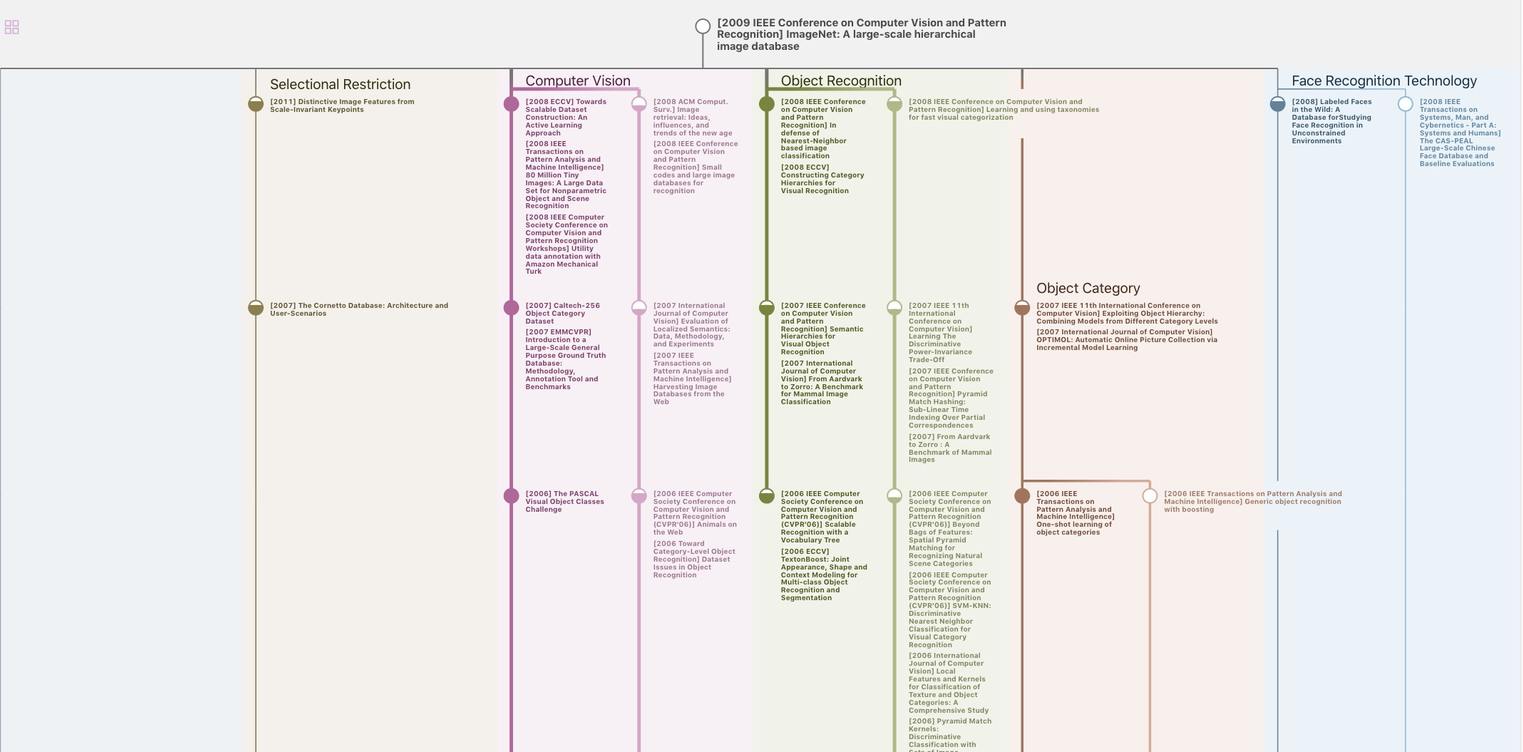
Generate MRT to find the research sequence of this paper
Chat Paper
Summary is being generated by the instructions you defined