Deep Reinforcement Learning with Inverse Jacobian based Model-Free Path Planning for Deburring in Complex Industrial Environment
Journal of Intelligent & Robotic Systems(2023)
摘要
In this study, we present an innovative approach to robotic deburring path planning by combining deep reinforcement learning (DRL) with an inverse Jacobian strategy. Existing model-based path planning methods, including sampling-based approaches, often suffer from computational complexity and challenges in capturing the dynamics of deburring systems. To overcome these limitations, our novel DRL-based framework for path planning leverages experiential learning to identify optimal deburring trajectories without relying on predefined models. This model-free approach is particularly suited for complex deburring scenarios with unknown system dynamics. Additionally, we employ an inverse Jacobian technique with a time-varying gain module (η(t) = e^2t) during training, which yields remarkable benefits in terms of exploration–exploitation balance and collision avoidance, enhancing the overall performance of the DRL agent. Through a series of experiments conducted in a simulated environment, we evaluate the efficacy of our proposed algorithm for deburring path planning. Our modified DRL-based approach, utilizing inverse kinematics with a time-varying gain module, demonstrates superior performance in terms of convergence speed, optimality, and robustness when compared to conventional path planning methods. Notably, in comparison to algorithms like sampling-based strategies, our model-free DRL-based approach outperforms these methods, achieving an exceptional average success rate of 97
更多查看译文
关键词
Path planning,Deburring,TD3,Deep reinforcement learning,Collision avoidance
AI 理解论文
溯源树
样例
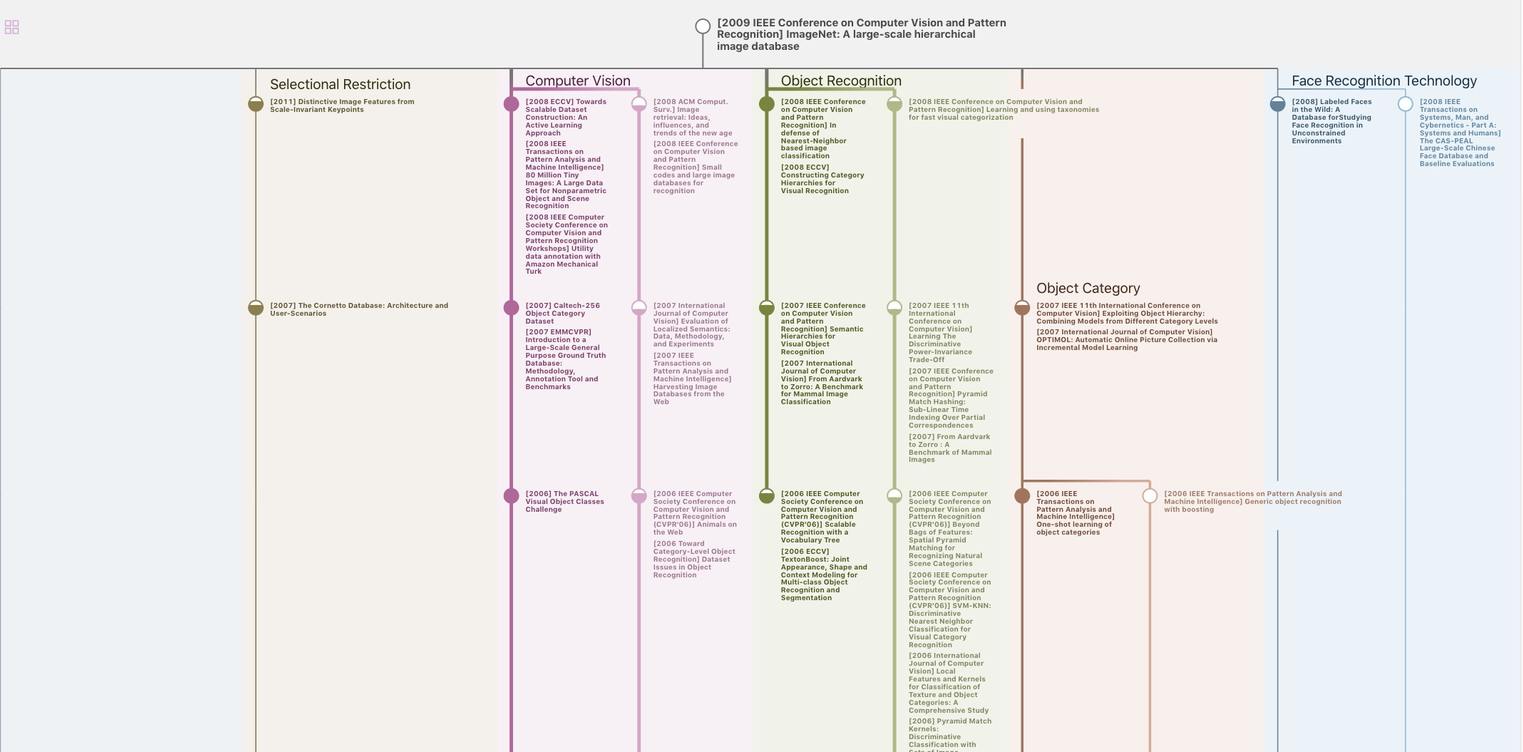
生成溯源树,研究论文发展脉络
Chat Paper
正在生成论文摘要