Two-phase early prediction method for remaining useful life of lithium-ion batteries based on a neural network and Gaussian process regression
Frontiers in Energy(2023)
摘要
Lithium-ion batteries (LIBs) are widely used in transportation, energy storage, and other fields. The prediction of the remaining useful life (RUL) of lithium batteries not only provides a reference for health management but also serves as a basis for assessing the residual value of the battery. In order to improve the prediction accuracy of the RUL of LIBs, a two-phase RUL early prediction method combining neural network and Gaussian process regression (GPR) is proposed. In the initial phase, the features related to the capacity degradation of LIBs are utilized to train the neural network model, which is used to predict the initial cycle lifetime of 124 LIBs. The Pearson coefficient’s two most significant characteristic factors and the predicted normalized lifetime form a 3D space. The Euclidean distance between the test dataset and each cell in the training dataset and validation dataset is calculated, and the shortest distance is considered to have a similar degradation pattern, which is used to determine the initial Dual Exponential Model (DEM). In the second phase, GPR uses the DEM as the initial parameter to predict each test set’s early RUL (ERUL). By testing four batteries under different working conditions, the RMSE of all capacity estimation is less than 1.2
更多查看译文
关键词
lithium-ion batteries,RUL prediction,double exponential model,neural network,Gaussian process regression (GPR)
AI 理解论文
溯源树
样例
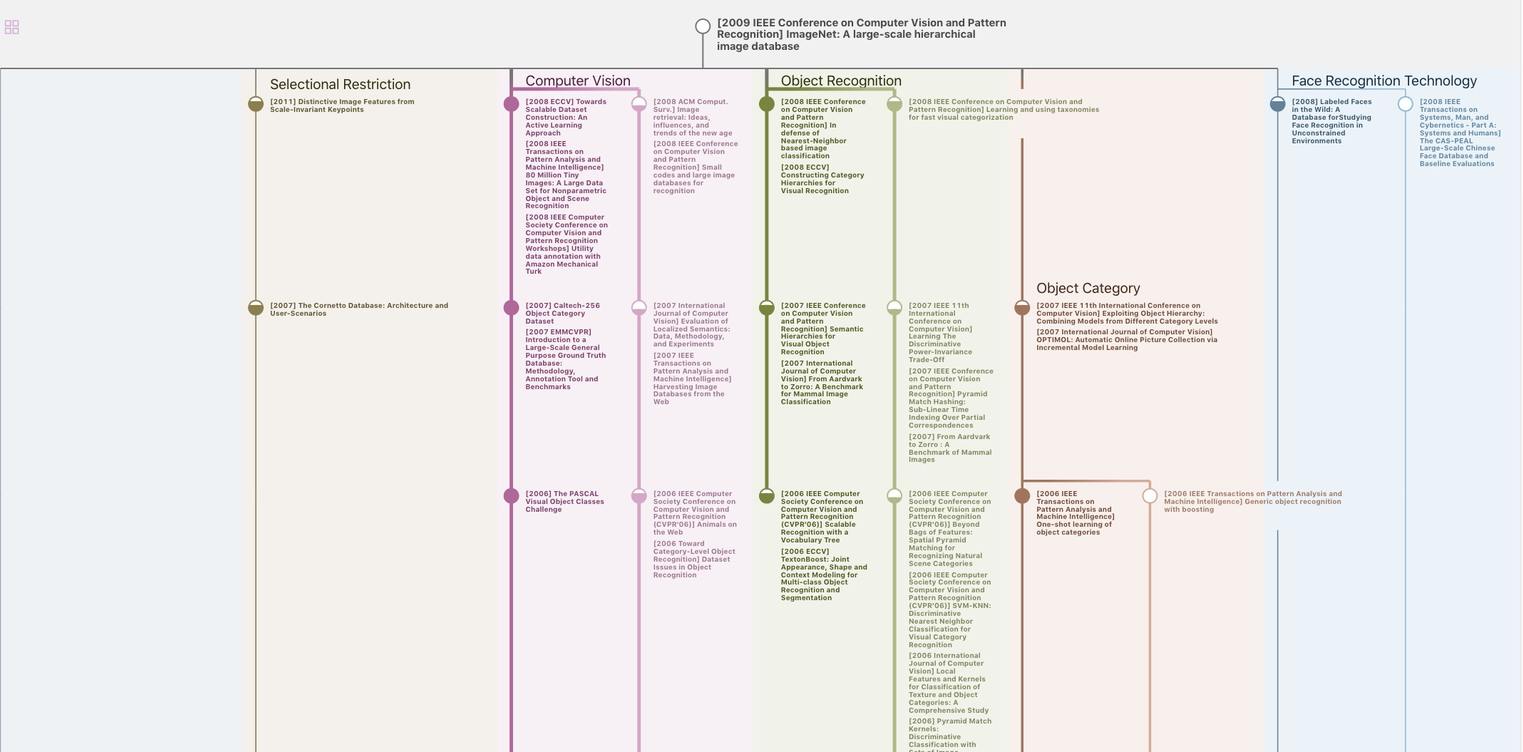
生成溯源树,研究论文发展脉络
Chat Paper
正在生成论文摘要