Fourier approach to goodness-of-fit tests for Gaussian random processes
Statistical Papers(2023)
Abstract
A new goodness-of-fit (GoF) test is proposed and investigated for the Gaussianity of the observed functional data. The test statistic is the Cramér-von Mises distance between the observed empirical characteristic functional (CF) and the theoretical CF corresponding to the null hypothesis stating that the functional observations (process paths) were generated from a specific parametric family of Gaussian processes, possibly with unknown parameters. The asymptotic null distribution of the proposed test statistic is derived also in the presence of these nuisance parameters, the consistency of the classical parametric bootstrap is established, and some particular choices of the necessary tuning parameters are discussed. The empirical level and power are investigated in a simulation study involving GoF tests of an Ornstein–Uhlenbeck process, Vašíček model, or a (fractional) Brownian motion, both with and without nuisance parameters, with suitable Gaussian and non-Gaussian alternatives.
MoreTranslated text
Key words
Functional data,Goodness-of-fit test,Characteristic functional,Gaussian processes
AI Read Science
Must-Reading Tree
Example
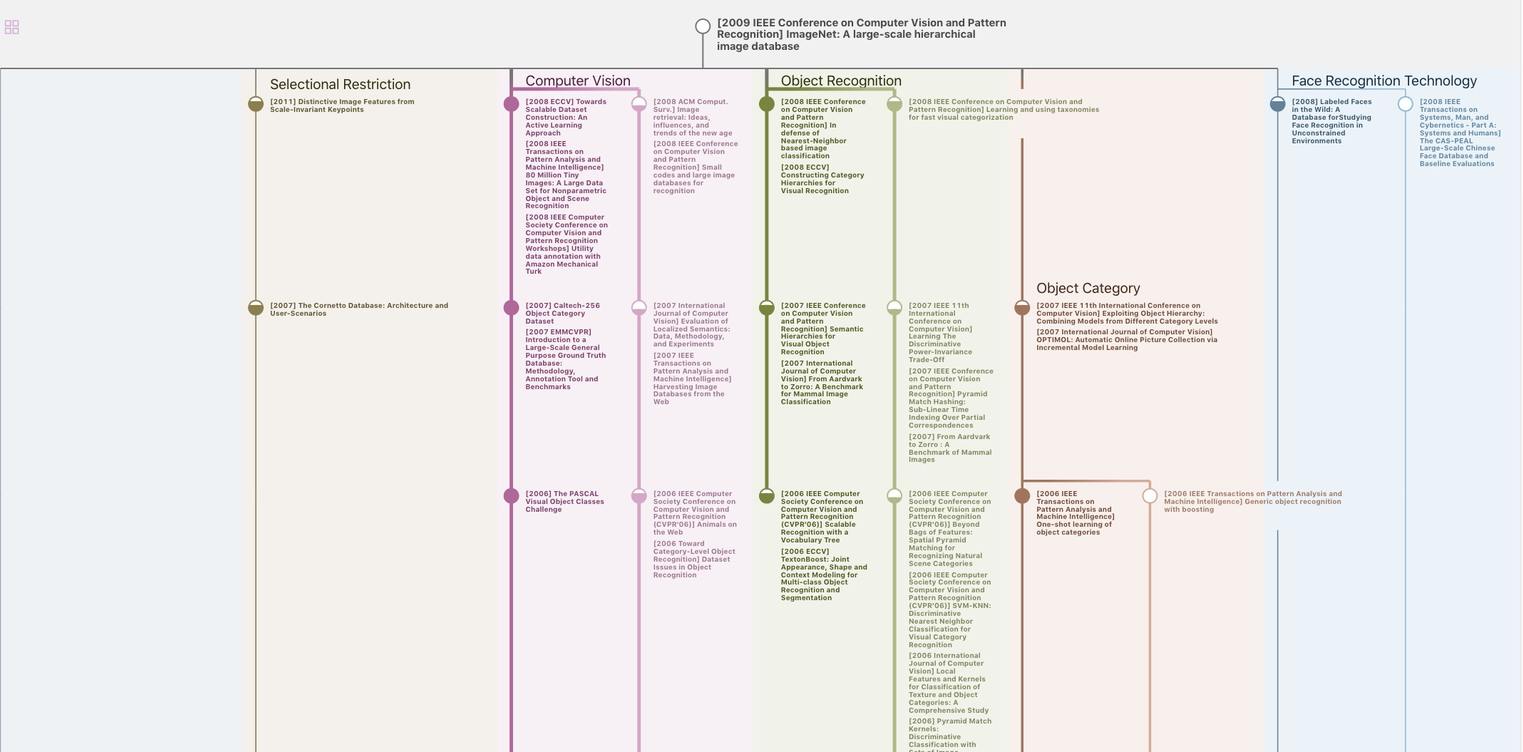
Generate MRT to find the research sequence of this paper
Chat Paper
Summary is being generated by the instructions you defined