Efficient Inverse Fractional Neural Network-Based Simultaneous Schemes for Nonlinear Engineering Applications
FRACTAL AND FRACTIONAL(2023)
Abstract
Finding all the roots of a nonlinear equation is an important and difficult task that arises naturally in numerous scientific and engineering applications. Sequential iterative algorithms frequently use a deflating strategy to compute all the roots of the nonlinear equation, as rounding errors have the potential to produce inaccurate results. On the other hand, simultaneous iterative parallel techniques require an accurate initial estimation of the roots to converge effectively. In this paper, we propose a new class of global neural network-based root-finding algorithms for locating real and complex polynomial roots, which exploits the ability of machine learning techniques to learn from data and make accurate predictions. The approximations computed by the neural network are used to initialize two efficient fractional Caputo-inverse simultaneous algorithms of convergence orders sigma+2 and 2 sigma+4, respectively. The results of our numerical experiments on selected engineering applications show that the new inverse parallel fractional schemes have the potential to outperform other state-of-the-art nonlinear root-finding methods in terms of both accuracy and elapsed solution time.
MoreTranslated text
Key words
fractional derivative,inverse fractional scheme,regression analyses,computational efficiency,neural network
AI Read Science
Must-Reading Tree
Example
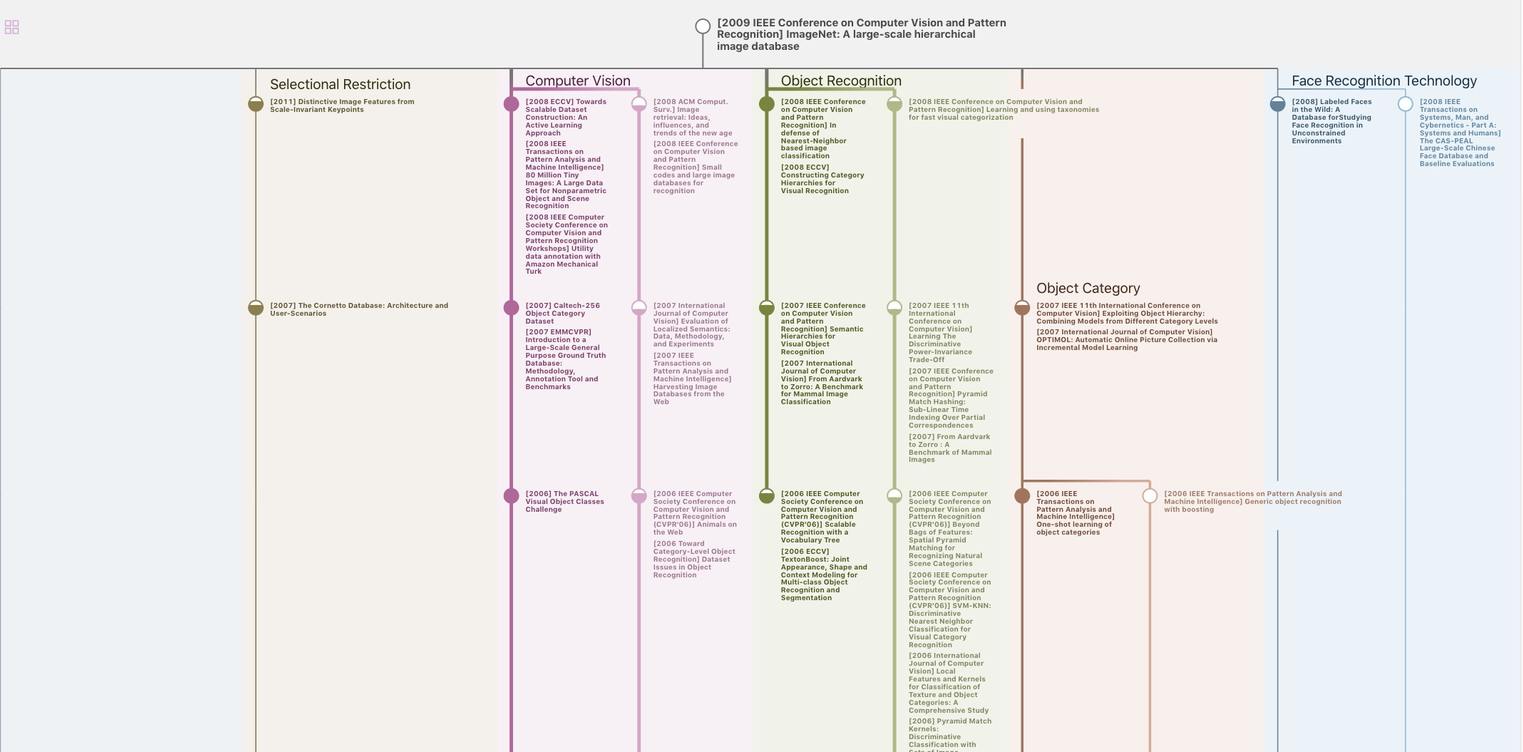
Generate MRT to find the research sequence of this paper
Chat Paper
Summary is being generated by the instructions you defined