Self-Attention Mechanism-Based Head Pose Estimation Network with Fusion of Point Cloud and Image Features
SENSORS(2023)
摘要
Head pose estimation serves various applications, such as gaze estimation, fatigue-driven detection, and virtual reality. Nonetheless, achieving precise and efficient predictions remains challenging owing to the reliance on singular data sources. Therefore, this study introduces a technique involving multimodal feature fusion to elevate head pose estimation accuracy. The proposed method amalgamates data derived from diverse sources, including RGB and depth images, to construct a comprehensive three-dimensional representation of the head, commonly referred to as a point cloud. The noteworthy innovations of this method encompass a residual multilayer perceptron structure within PointNet, designed to tackle gradient-related challenges, along with spatial self-attention mechanisms aimed at noise reduction. The enhanced PointNet and ResNet networks are utilized to extract features from both point clouds and images. These extracted features undergo fusion. Furthermore, the incorporation of a scoring module strengthens robustness, particularly in scenarios involving facial occlusion. This is achieved by preserving features from the highest-scoring point cloud. Additionally, a prediction module is employed, combining classification and regression methodologies to accurately estimate head poses. The proposed method improves the accuracy and robustness of head pose estimation, especially in cases involving facial obstructions. These advancements are substantiated by experiments conducted using the BIWI dataset, demonstrating the superiority of this method over existing techniques.
更多查看译文
关键词
point cloud data,head pose estimation,PointNet,ResNet,multi-source feature fusion
AI 理解论文
溯源树
样例
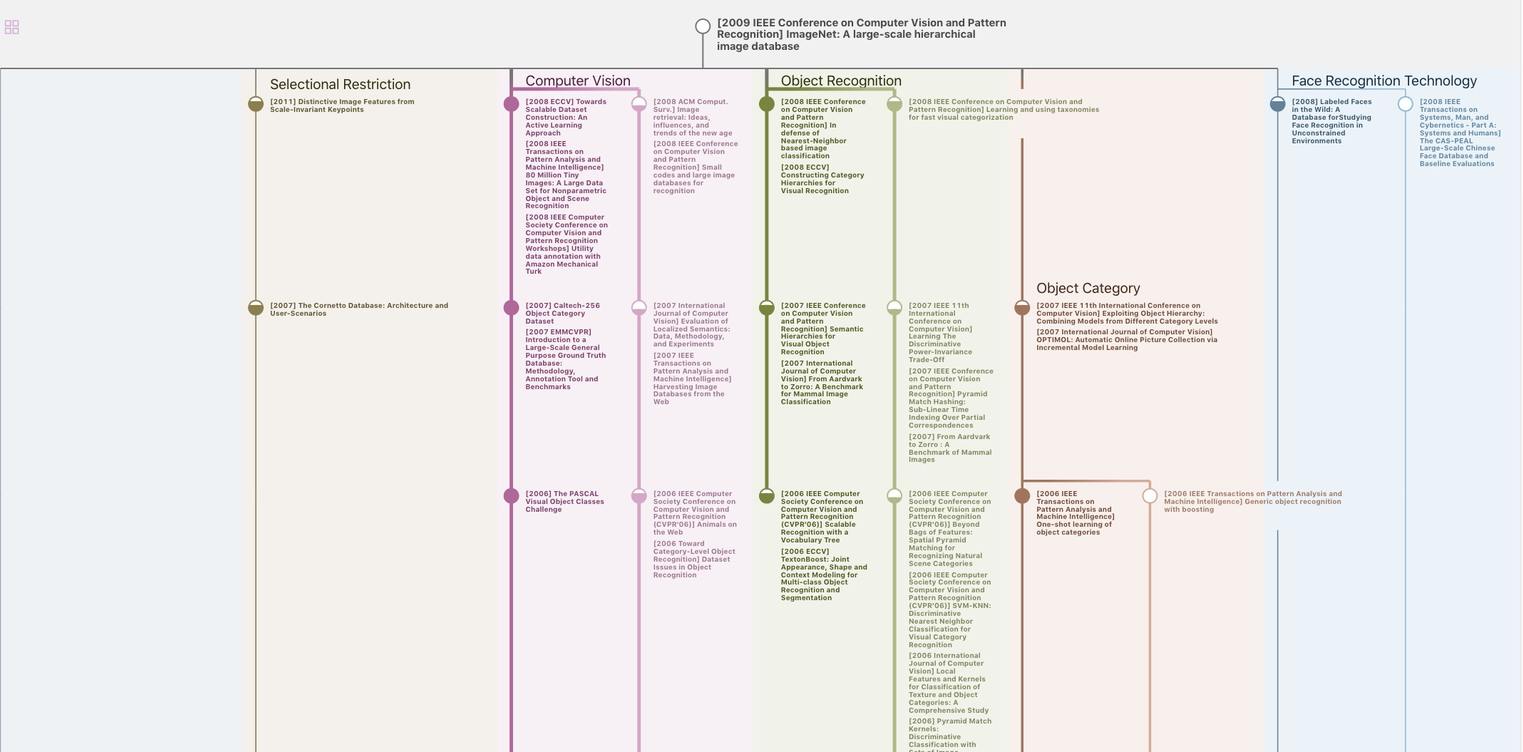
生成溯源树,研究论文发展脉络
Chat Paper
正在生成论文摘要