Spatial-temporal graph neural network based on gated convolution and topological attention for traffic flow prediction
Applied Intelligence(2023)
Abstract
Accurate traffic flow prediction is essential for developing intelligent transportation systems (ITS) and providing real-time traffic applications. This study proposes a novel Spatial-Temporal Graph Neural Network based on Gated Convolution and Topological Attention (STGNN-GCTA) to accurately model complex spatiotemporal traffic flow correlations. In the temporal dimension, we design a novel Gated-Memory Convolutional Neural Network (GMCNN) to capture the non-linear temporal dependencies by controlling the output based on the timing information position. In the spatial dimension, we develop a Multilayer Graph Topological Attention Network (MGTAN) to capture the dynamic spatial dependencies by identifying high-impact neighborhood segments in each time step. In particular, we improve the model’s prediction robustness in a noisy environment using the Network Smoothing Training (NST) method. Experimental results on two public traffic datasets demonstrate that STGNN-GCTA has higher prediction accuracy and execution efficiency than baseline methods and exhibits excellent robustness.
MoreTranslated text
Key words
Traffic flow prediction,ITS,Attention mechanism,Causal convolution,Missing data recovery,Spatiotemporal correlations
AI Read Science
Must-Reading Tree
Example
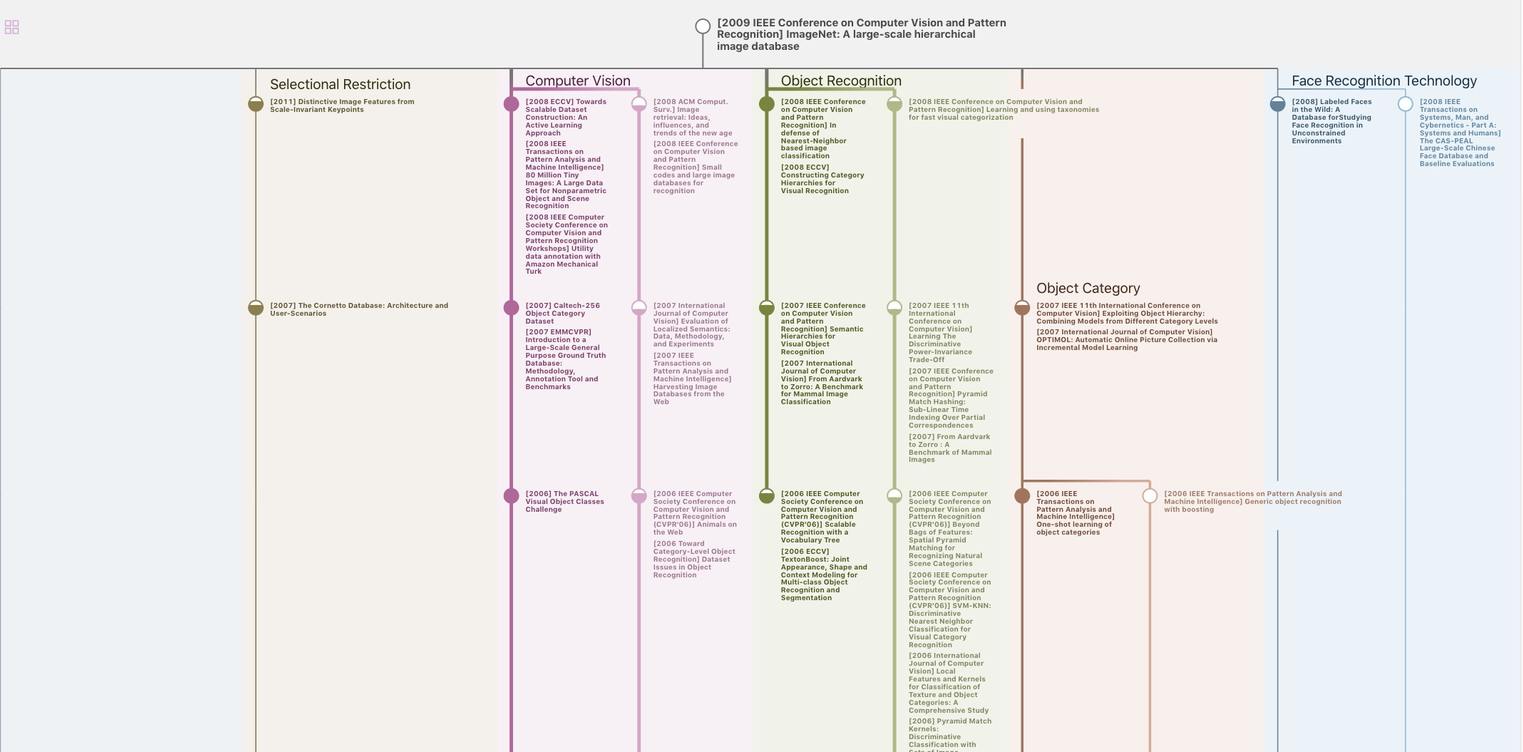
Generate MRT to find the research sequence of this paper
Chat Paper
Summary is being generated by the instructions you defined