Dependency, reciprocity, and informal mentorship in predicting long-term research collaboration: A co-authorship matrix-based multivariate time series analysis
JOURNAL OF INFORMETRICS(2024)
摘要
In this study, we examine the roles of dependency, reciprocity, and informal mentorship in the prediction of long-term research collaboration in five disciplines. We use co-authorship matrixbased multivariate time series features and interpretable machine learning to train long-term collaboration prediction models and interpret the feature importance of trained models. Overall, long-term research collaboration that is defined using various standards was rare across the examined disciplines, and the prediction results were moderate to good. We found dependency, reciprocity, and informal mentorship to have different roles in different disciplines. Among the three, informal mentorship was important in predicting long-term research collaboration in Agriculture, Geology, and Library and Information Science. Reciprocity, which measures the interdependence between two researchers was important to prediction in the fields of Agriculture and Geology. Finally, dependency was important in all the disciplines with varying degrees of importance.
更多查看译文
关键词
Long-term research collaboration,Co-authorship prediction,dependency,Reciprocity,Informal mentorship,Interpretable machine learning
AI 理解论文
溯源树
样例
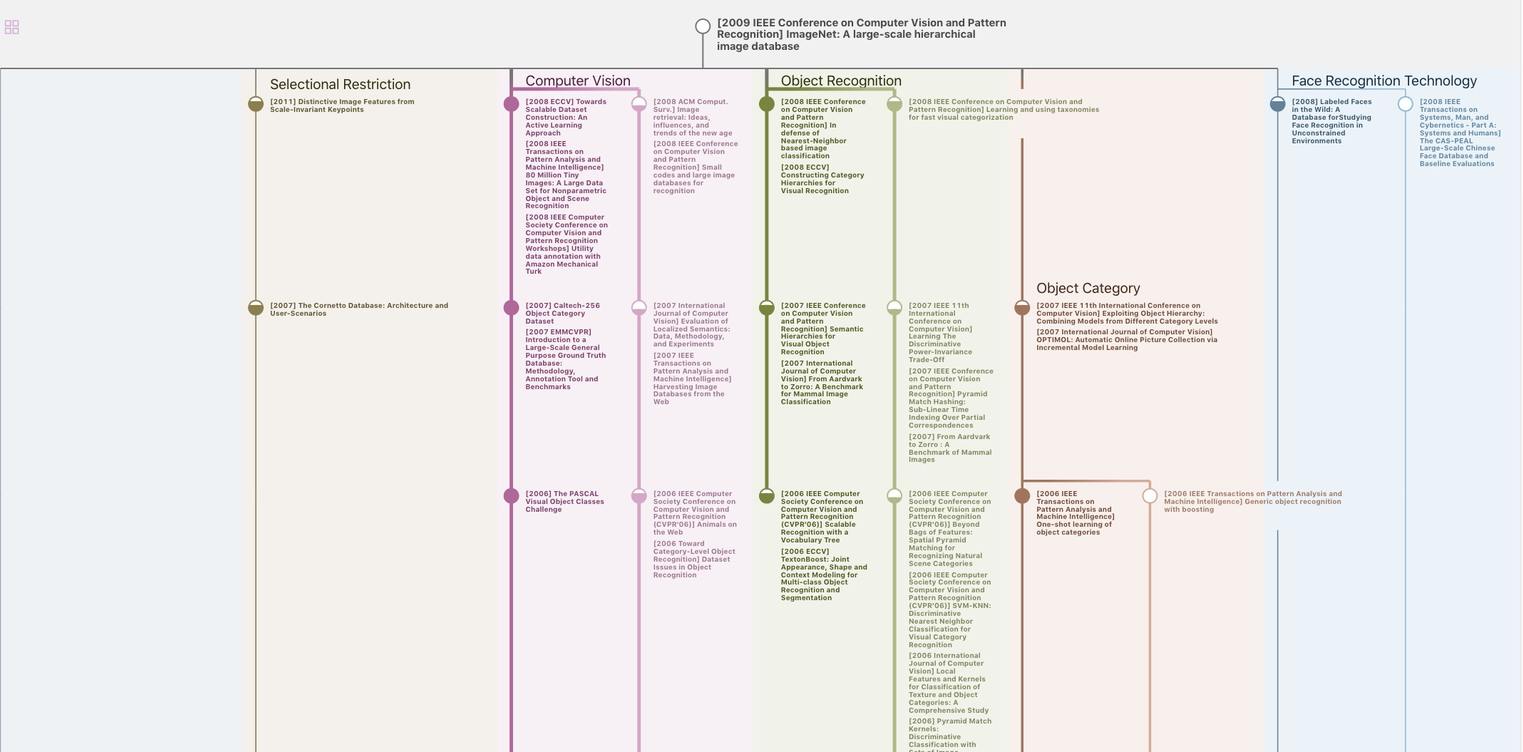
生成溯源树,研究论文发展脉络
Chat Paper
正在生成论文摘要