Neural network informed day-ahead scheduling of pumped hydro energy storage
ENERGY(2024)
摘要
This paper presents a neural network-constrained optimization model for the optimal scheduling of pumped hydro energy storage. Neural networks are trained offline to capture the complex head-dependent performance curves in both pump and turbine modes using actual operation data. The trained models are then embedded into the optimization framework that yields the optimal and physics-compliant day-ahead scheduling in energy and reserve markets for the pumped hydro energy storage. To identify the trade-off between modeling accuracy and computation burden, different neural network architectures are investigated, along with the impact of neural network sparsity, i.e., weights pruning to reduce dimensionality. The proposed approach is then compared with state-of-the-art solutions, such as piecewise linear approximations. To that end, a detailed simulator of the pumped hydro energy storage, mimicking its minute-wise behavior, is developed to accurately assess the feasibility and economic performance of the resulting schedules. Results demonstrate the ability of neural networks to better guide the optimization model, thus leading to higher profits while keeping acceptable solving times, especially when weight pruning is leveraged. In particular, we show that accurately capturing the non-linear characteristics of pumped hydro energy storage is critical to offer reliable reserve commitments to power systems.
更多查看译文
关键词
Machine learning,Neural network informed optimization,Pumped hydro energy storage,Data-driven optimization,Day-ahead scheduling
AI 理解论文
溯源树
样例
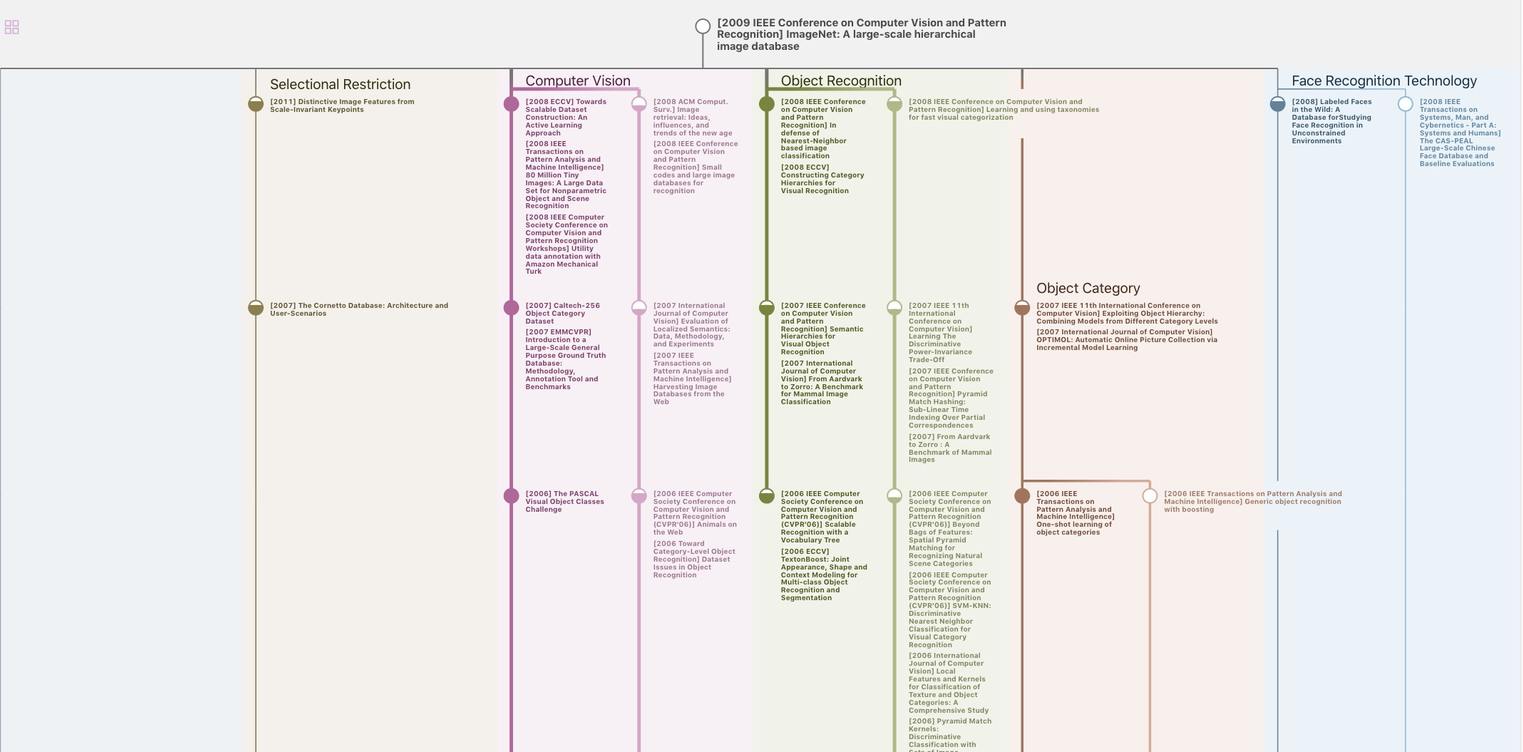
生成溯源树,研究论文发展脉络
Chat Paper
正在生成论文摘要