Machine learning informed tetragonal ratio c/a of martensite
Computational Materials Science(2024)
Abstract
Tetragonal ratio (c/a) is a critical structural parameter that heavily decides plenty of performances of tetragonal martensitic materials. Nevertheless, the knowledge about c/a remains limited and there is still no definitive strategy to tailor it. In this work, the machine learning method, combined with high-throughput ab-initio calculation, was introduced as an attempt to investigate c/a. After training, a high-precision random forest model of predicting c/a was established. Combining a five-step descriptor screening and visualization analyses, the most pertinent parameters deciding c/a, i.e., mean melting point (TM) and mean volume (V) of constituent elements, were identified. Furthermore, a simple relation between c/a and these two parameters, i.e., c/a = m TM/V + b where the m and b are constants, was proposed. Surprisingly, this relation exhibits an exceptional generalization performance in a wide range of untrained materials. This work is expected to provide an explicit, efficient and general route to tailor c/a and hence promote the design of advanced martensitic materials.
MoreTranslated text
Key words
Martensitic transformation,Tetragonal ratio c / a,Shape memory alloy,Machine learning,First-principles calculations
AI Read Science
Must-Reading Tree
Example
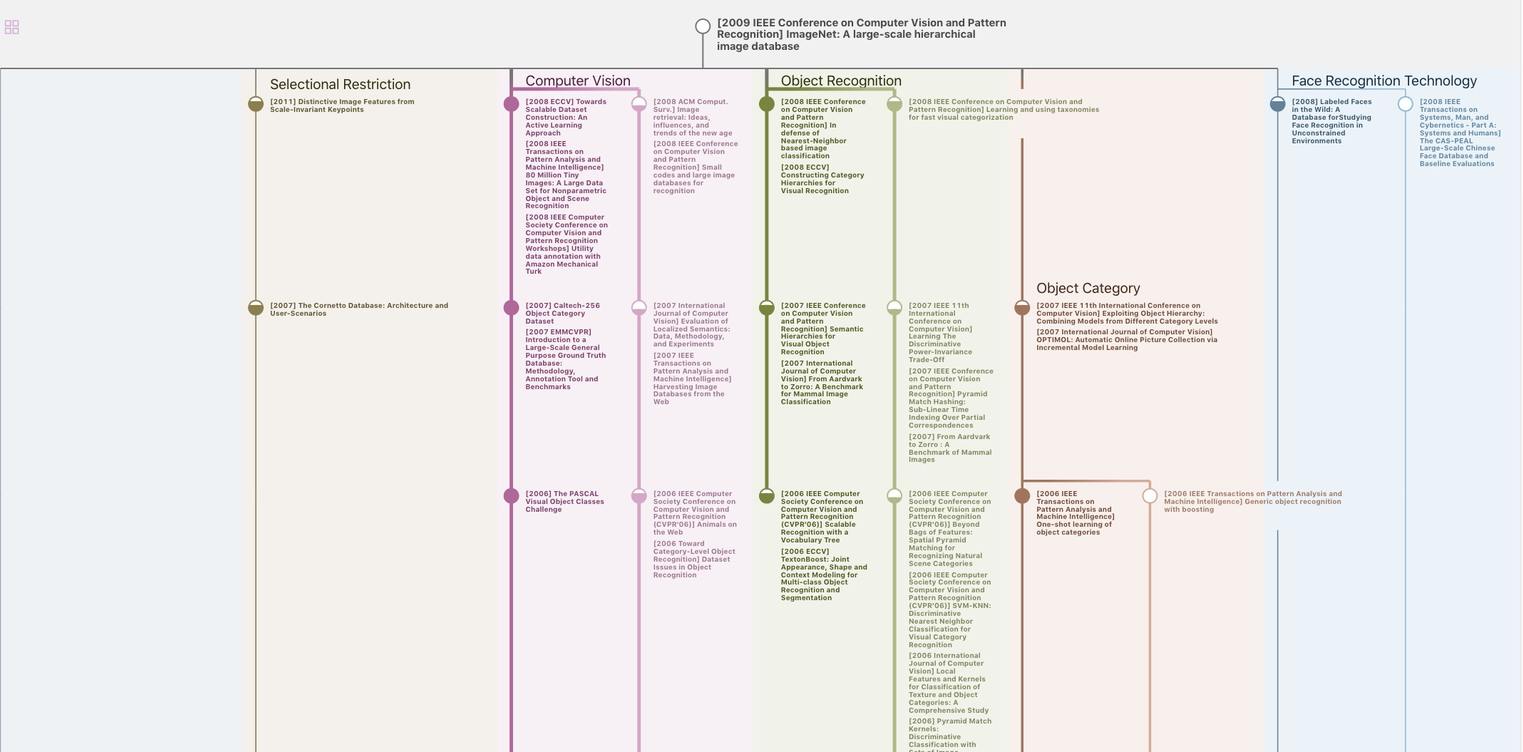
Generate MRT to find the research sequence of this paper
Chat Paper
Summary is being generated by the instructions you defined