AHMN: A multi-modal network for long MOOC videos chapter segmentation
Multimedia Tools and Applications(2023)
Abstract
This paper proposes a task named MOOC Videos Chapter Segmentation (MVCS) which is a significant problem in the field of video understanding. To solve this problem, we first introduce a dataset called MOOC Videos Understanding (MVU) which consists of approximately 10k annotated chapters organized by 120k snippets from 400 MOOC videos where chapters and snippets are two levels of video unit proposed in this paper for hierarchical level expression of videos. Then, we design the Attention-based Hierarchical bi-LSTM Multi-modal Network (AHMN) based on three core ideas: (1) we take advantage of the features of multi-modal semantic elements, including video, audio, and text, along with an attention-based multi-modal fusion module to extract video information in a comprehensive way. (2) we focus on chapters boundaries rather than the content recognition of chapters themselves, so we develop Boundary Predict Network (BPN) to label boundaries between chapters. (3) we exploit the semantic consistency between snippets and develop Consistency Modeling as an auxiliary task to improve the performance of BPN. Our experiments demonstrate that the proposed AHMN can solve the MVCS precisely, outperforming previous methods on all evaluation metrics.
MoreTranslated text
Key words
Video understanding,Low-quality video,Multimodal embedding,Attention mechanism
AI Read Science
Must-Reading Tree
Example
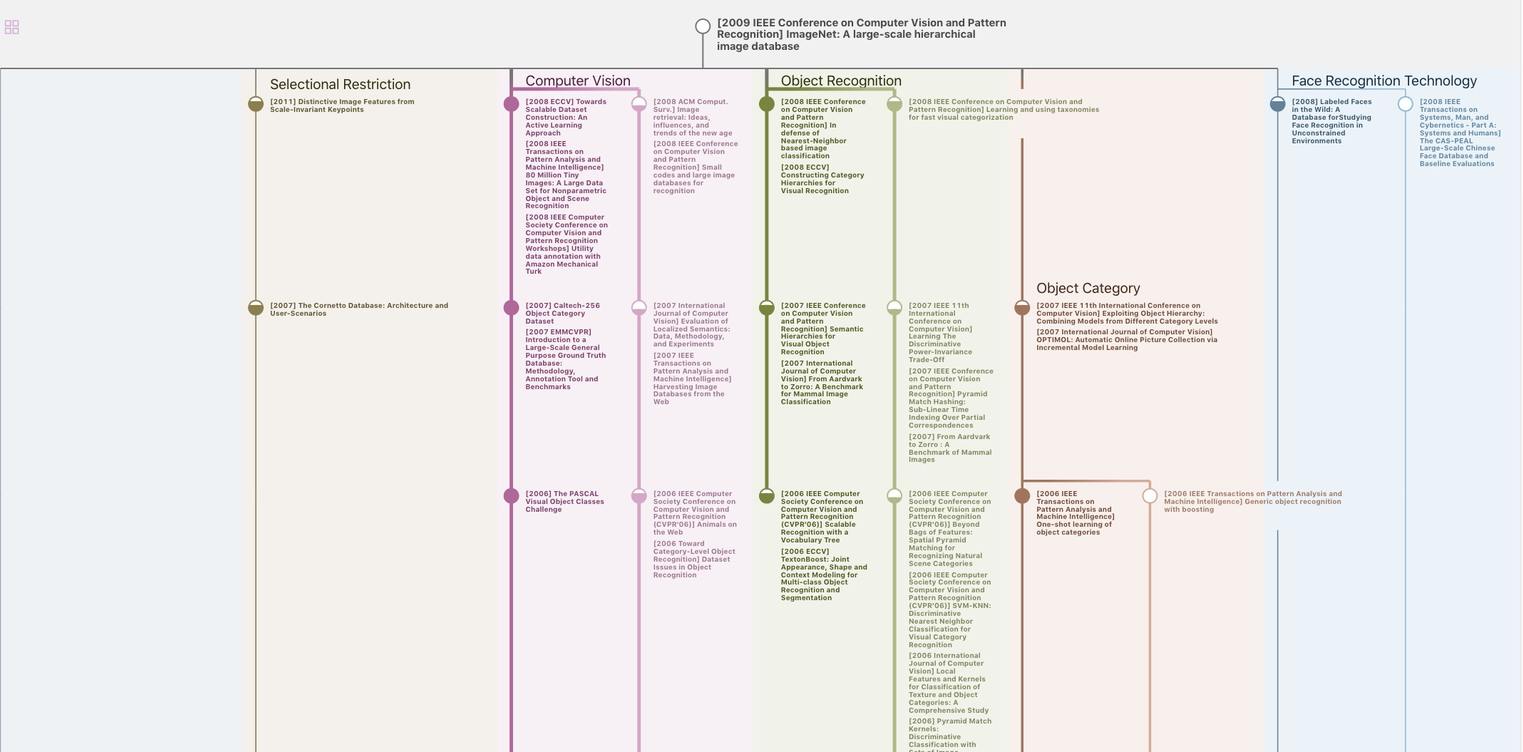
Generate MRT to find the research sequence of this paper
Chat Paper
Summary is being generated by the instructions you defined