Learning consumer preferences through textual and visual data: a multi-modal approach
Electronic Commerce Research(2023)
摘要
This paper proposes a novel multi-modal probabilistic topic model (LSTIT) to infer consumer preferences by jointly leveraging textual and visual data. Specifically, we use the title and image of the items purchased by consumers. Considering that the titles of items are relatively short text, we thus restrict the topic assignment for these titles. Meanwhile, we employ the same topic distribution to model the relationship between the title and the image of the item. To learn consumer preferences, the proposed model extracts several important dimensions based on textual words in titles and visual features in images. Experiments on the Amazon dataset show that the proposed model outperforms other baseline models for the task of learning consumer preferences. Our findings provide significant implications for managers to understand users’ personalized interests behind purchase behavior from a fine-grained level and a multi-modal perspective.
更多查看译文
关键词
User preferences,Multi-modal data,Topic model,Explainable learning
AI 理解论文
溯源树
样例
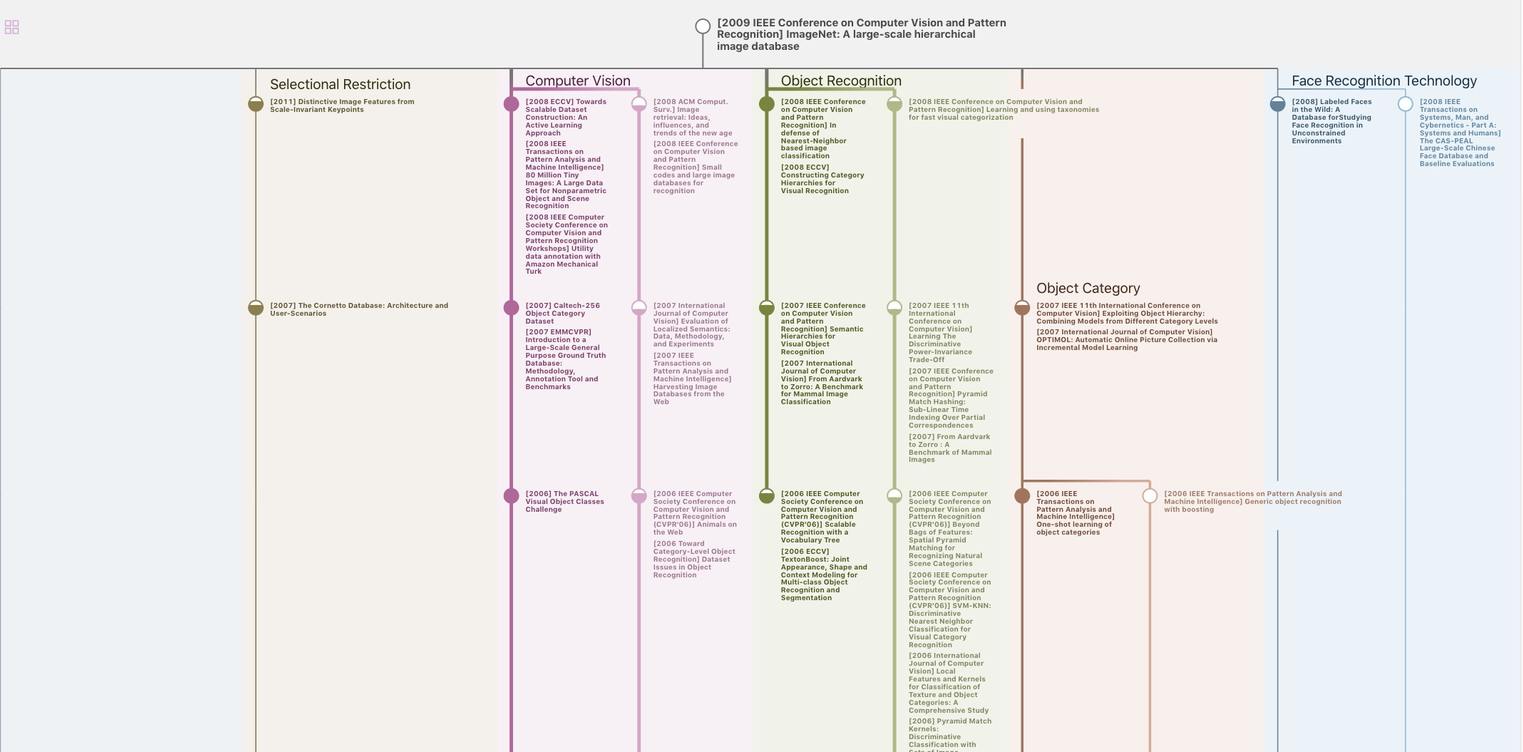
生成溯源树,研究论文发展脉络
Chat Paper
正在生成论文摘要