SleepLess : personalized sleep monitoring using smartphones and semi-supervised learning
CSI Transactions on ICT(2023)
摘要
Sleep affects our bodily functions and is critical in promoting every individual’s well-being. To that end, sleep health monitoring research has gained interest recently, including coupling data-driven AI techniques with mHealth adaptations of wearable, smartphone, and contactless-sensing modalities. Regardless, prior works, by and large, require gathering sufficient ground truth data to develop personalized and highly accurate sleep prediction models. This requirement inherently presents a challenge of such models underperforming when inferring sleep on new users without labeled data. In this paper, we propose SleepLess , which uses a semi-supervised learning pipeline over unlabeled data sensed from the user’s smartphone network activity to develop personalized models and detect their sleep duration for the night. Specifically, it uses a pre-trained model on an existing set of users to produce pseudo labels for unlabeled data of a new user and achieves personalization by fine-tuning over selectively picking the pseudo labels. Our IRB-approved user study found SleepLess model yielding around 96% accuracy, between 12–27 min of sleep time error and 18–25 min of wake time error. Comparison against other approaches that sought to predict with fewer labeled data found SleepLess , similarly yielding best performance. Our study demonstrates the feasibility of achieving personalized sleep prediction models by utilizing unlabeled data extracted from network activity of users’ smartphones, using a semi-supervised approach.
更多查看译文
关键词
Semi-supervised learning,Time-series analysis,Sleep,Passive sensing
AI 理解论文
溯源树
样例
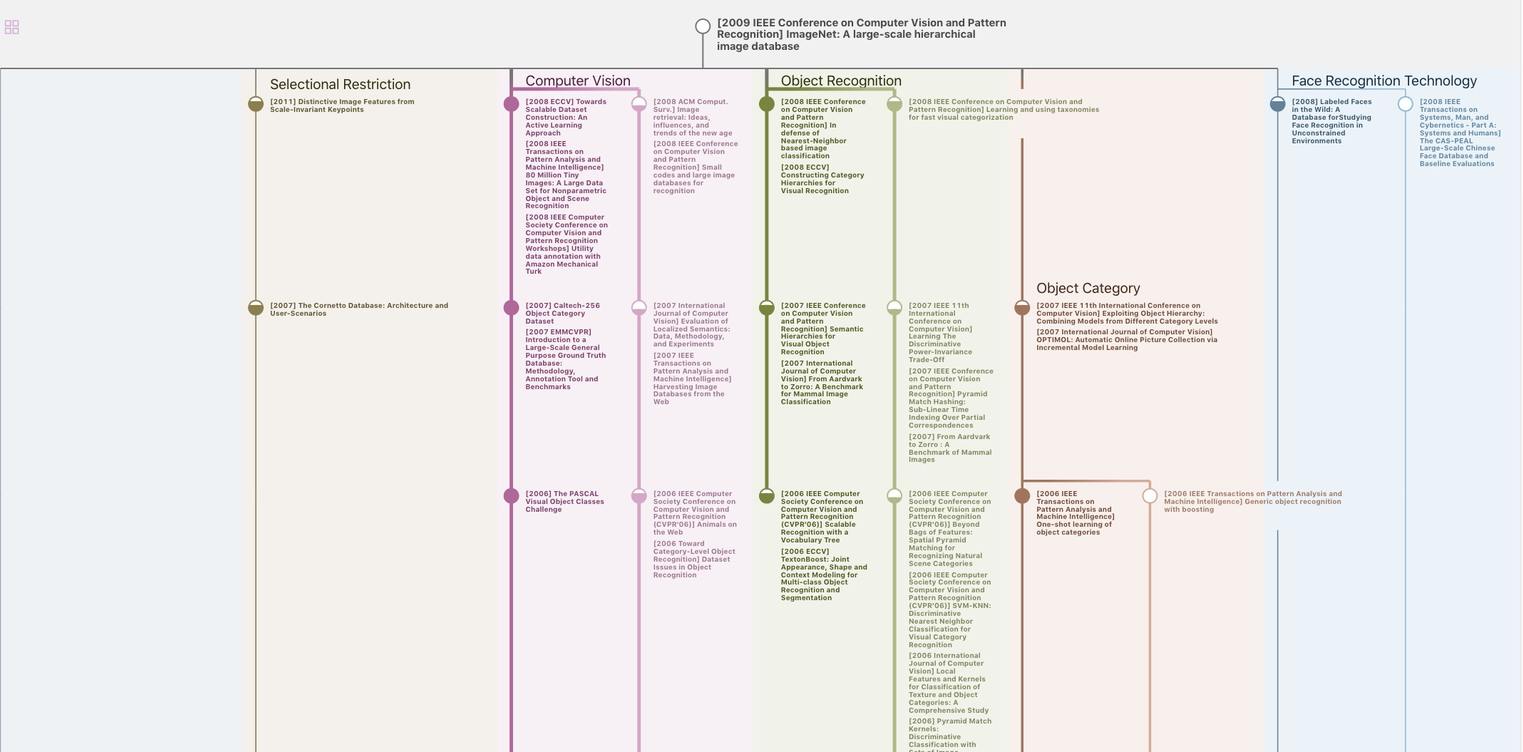
生成溯源树,研究论文发展脉络
Chat Paper
正在生成论文摘要