Advancing Mental Health Diagnostics: GPT-Based Method for Depression Detection
2023 62ND ANNUAL CONFERENCE OF THE SOCIETY OF INSTRUMENT AND CONTROL ENGINEERS, SICE(2023)
摘要
In this paper, we present a novel artificial intelligence ( AI) application for depression detection, using advanced transformer networks to analyse clinical interviews. By incorporating simulated data to enhance traditional datasets, we overcome limitations in data protection and privacy, consequently improving the model's performance. Our methodology employs BERT-based models, GPT-3.5, and ChatGPT-4, demonstrating state-of-the-art results in detecting depression from linguistic patterns and contextual information that significantly outperform previous approaches. Utilising the DAIC-WOZ and Extended-DAIC datasets, our study showcases the potential of the proposed application in revolutionising mental health care through early depression detection and intervention. Empirical results from various experiments highlight the efficacy of our approach and its suitability for real-world implementation. Furthermore, we acknowledge the ethical, legal, and social implications of AI in mental health diagnostics. Ultimately, our study underscores the transformative potential of AI in mental health diagnostics, paving the way for innovative solutions that can facilitate early intervention and improve patient outcomes.
更多查看译文
关键词
Mental Health,Depression Detection,Deep Learning,NLP Transformer LLM,GPT-3.5,ChatGPT-4
AI 理解论文
溯源树
样例
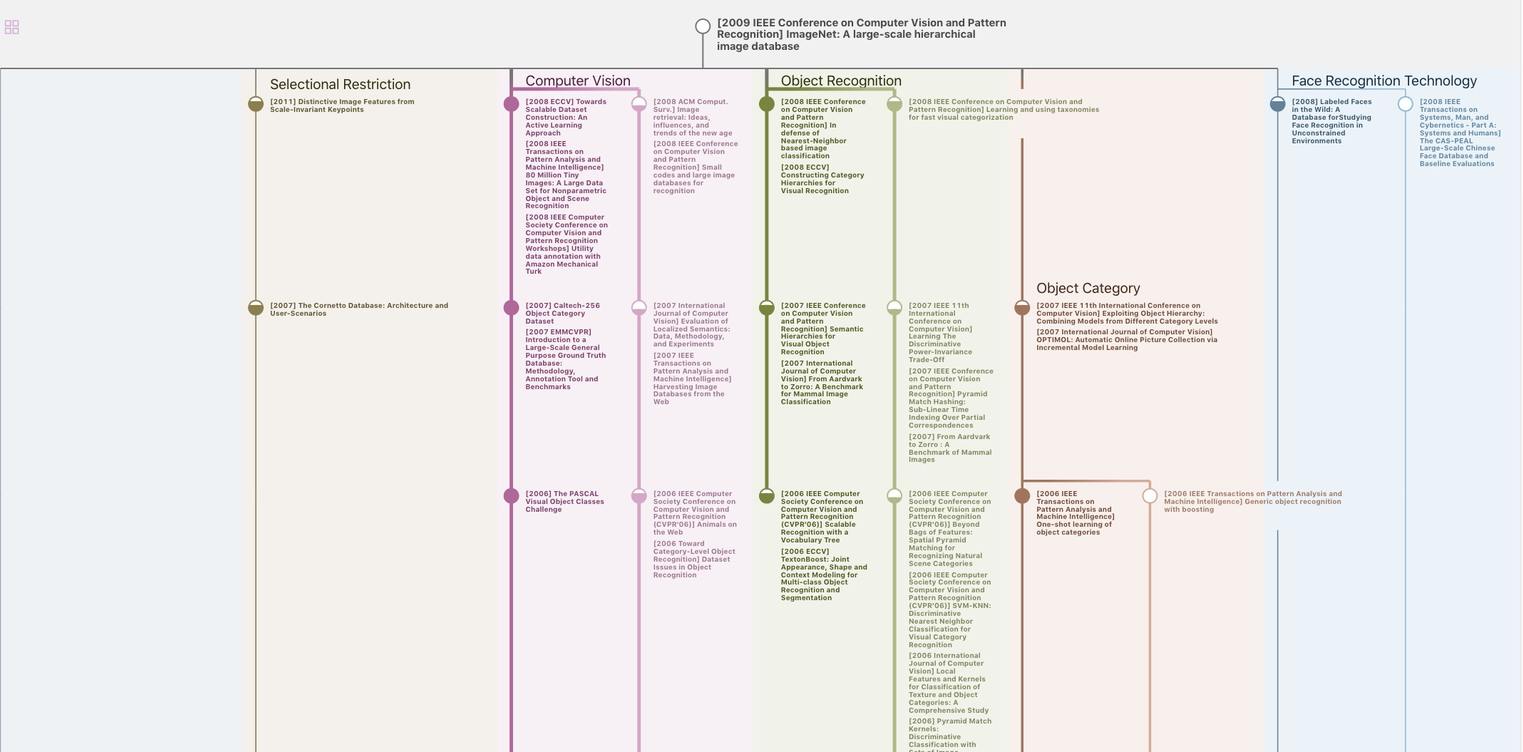
生成溯源树,研究论文发展脉络
Chat Paper
正在生成论文摘要