An Empirical Analysis of Rebalancing Methods for Security Issue Report Identification.
2023 IEEE 28th Pacific Rim International Symposium on Dependable Computing (PRDC)(2023)
摘要
Identifying security vulnerabilities in issue reports is a complex and time-sensitive task that when carried out effectively and in a timely manner can prevent attackers from exploiting software systems. While it is possible to address this using machine learning, the heavy imbalance of the datasets involved requires a meticulous use of rebalancing methods to achieve reasonably effective models. In this paper we analyze the effectiveness of different data rebalancing methods (e.g., oversampling and undersampling) applied to the classification of security issue reports using machine learning techniques. Our results using the Ubuntu dataset show that oversampling is an overall better strategy for rebalancing, SVMSMOTE and Random Undersampling are the individual methods that show the best performance. We also found that variations in the proportion of the minority class have little effect on the difference in the effectiveness of the best methods. Overall, our results are useful for creating more effective machine learning models for the automatic identification of security bug reports.
更多查看译文
关键词
Issue report,security issue report,vulnerability,security,software development,machine learning
AI 理解论文
溯源树
样例
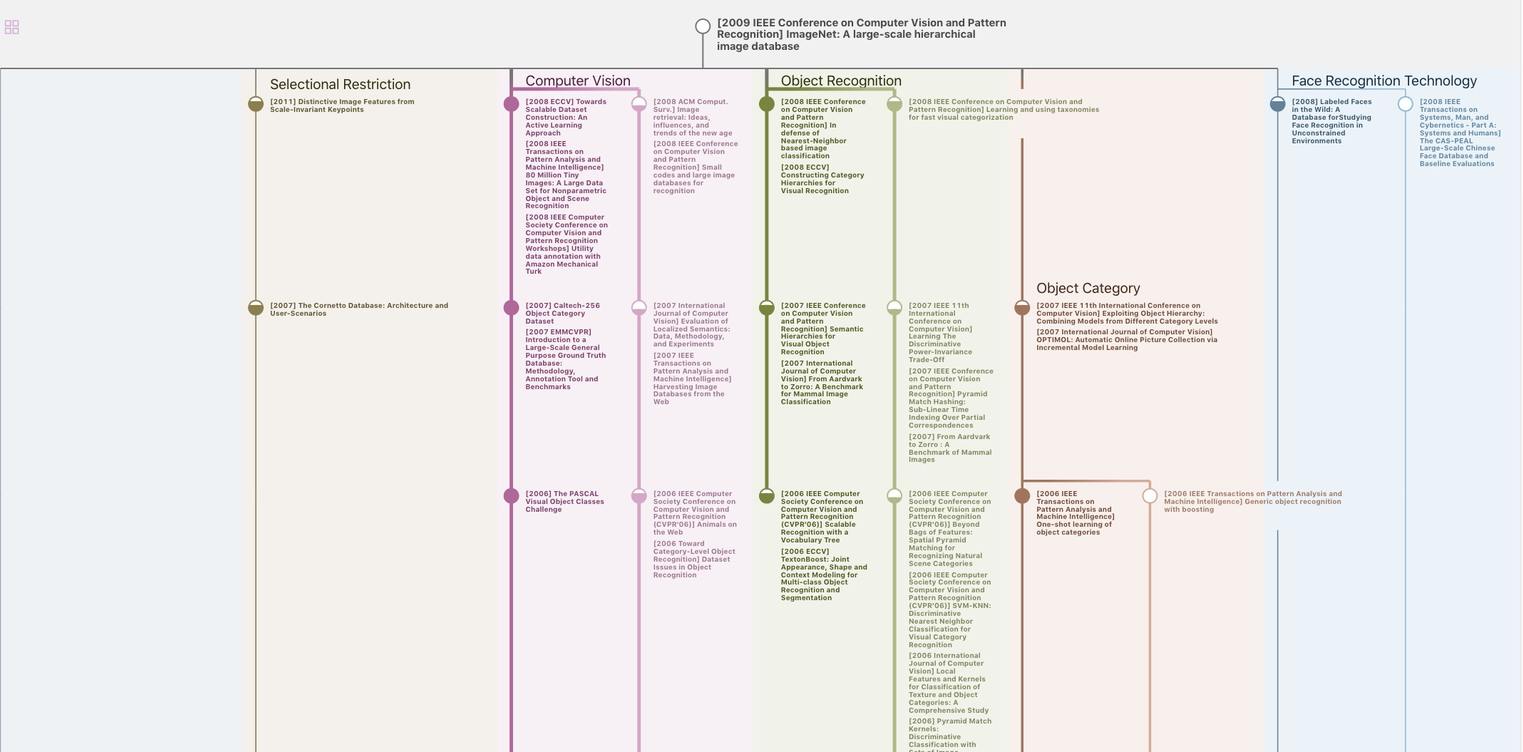
生成溯源树,研究论文发展脉络
Chat Paper
正在生成论文摘要