A Microwave Sensing System Enhanced by a Machine Learning Algorithm for Alzheimer’s Disease Early Detection
2023 IEEE Conference on Antenna Measurements and Applications (CAMA)(2023)
Abstract
Diagnostic techniques able to detect Alzheimer’s disease in its early stage are costly, invasive, and lack portability. Early detection is fundamental to give patients the best treatment and the possibility to plan their future life accordingly, and benefits caregivers and medical professionals. To address the drawbacks of current detection technologies, microwave imaging and sensing are emerging as a non-intrusive, relatively low-cost alternative methods to differentiate between normal and pathological states. The physiological basis behind this difference is the change in permittivity within the cerebrospinal fluid of Alzheimer’s patients. In this study, we investigate machine learning applied to microwave sensing data using a multilayer perceptron classifier. Data collection is made through experiments involving a realistic multi-tissue head phantom filled with liquids mimicking different disease severities. We explore various architectural approaches for the classification algorithm, evaluating the performances of binary classification for different combinations of hyperparameters and using different subsets of the total data pool as training data. Our preliminary findings affirm the potential of the proposed technique, reaching high correct classification scores on test data not used during training.
MoreTranslated text
Key words
Alzheimer’s disease,microwave antennas,machine learning,realistic phantom
AI Read Science
Must-Reading Tree
Example
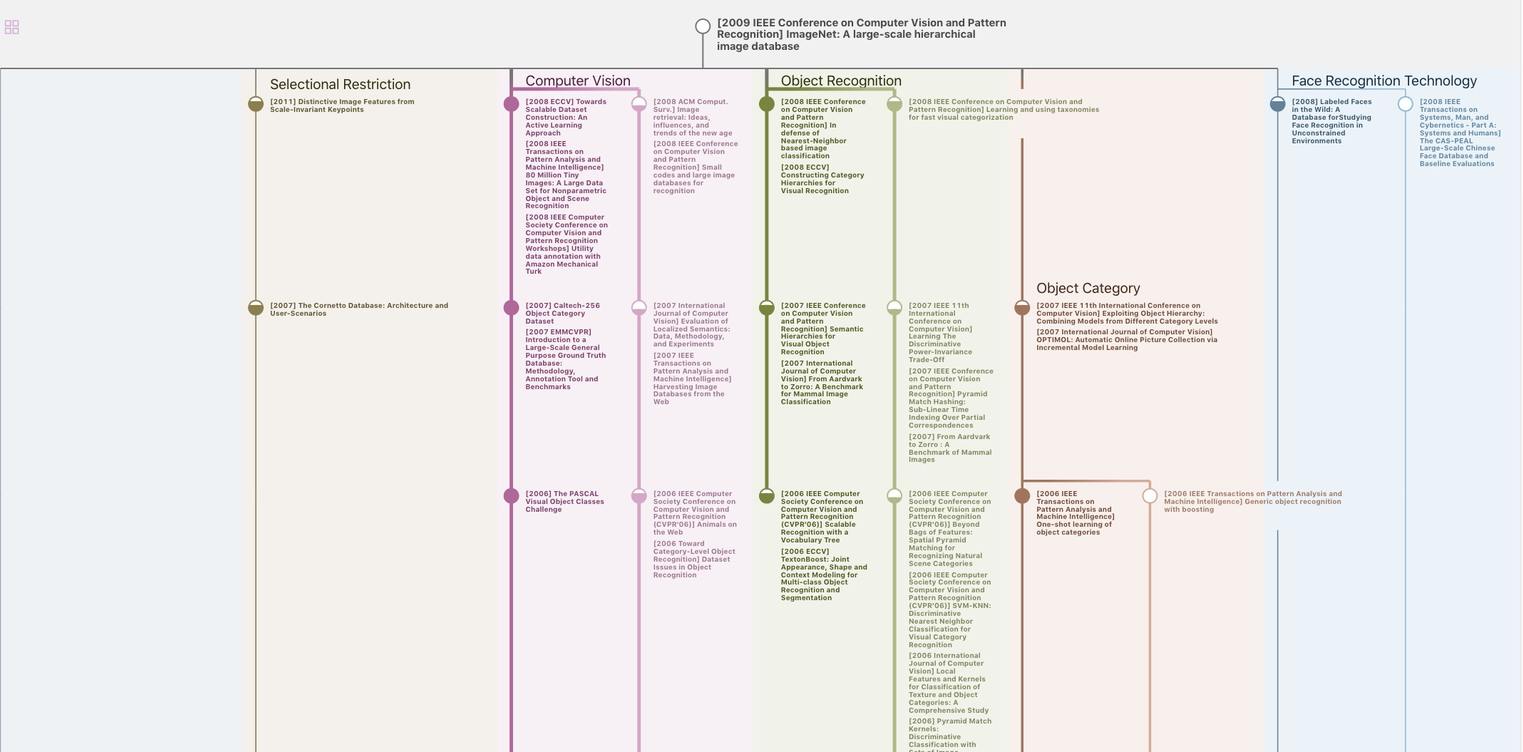
Generate MRT to find the research sequence of this paper
Chat Paper
Summary is being generated by the instructions you defined