Cross-Architecture Relational Consistency for Point Cloud Self-Supervised Learning
2023 IEEE 35TH INTERNATIONAL CONFERENCE ON TOOLS WITH ARTIFICIAL INTELLIGENCE, ICTAI(2023)
摘要
In this paper, we present a novel Cross-architecture Relational Consistency (CRC) framework for point cloud self-supervised learning. Since the features produced by encoders of different architectures contain different information in point cloud processing, we design a cross-architecture framework to obtain complementary feature representations, which utilizes two parallel encoders, i.e., PointNet++ (point-based) and SparseConv (voxel-based). To better preserve the uniqueness of feature spaces of different encoders, the CRC framework learns the cross-architecture consistency of inter-instance relations in the feature space of two encoders through distribution alignment, which aligns the similarity distributions calculated from the two spaces using the KL divergence objective. The CRC framework not only learns complementary information from different encoders but also obtains two pre-trained models that can be used separately for downstream tasks. The distribution alignment objective enables the CRC framework to capture more inter-sample correlation information, thereby better preserving meaningful semantic structures. We validate the effectiveness of the CRC framework on the KITTI 3D object detection task, where it outperforms the previous self-supervised learning methods. Further, the ablation studies validate the potency of our approach for a better point cloud understanding. Code: https://github.com/Physu/CRCSSL
更多查看译文
关键词
Self-supervised learning,Point cloud,Relational consistency
AI 理解论文
溯源树
样例
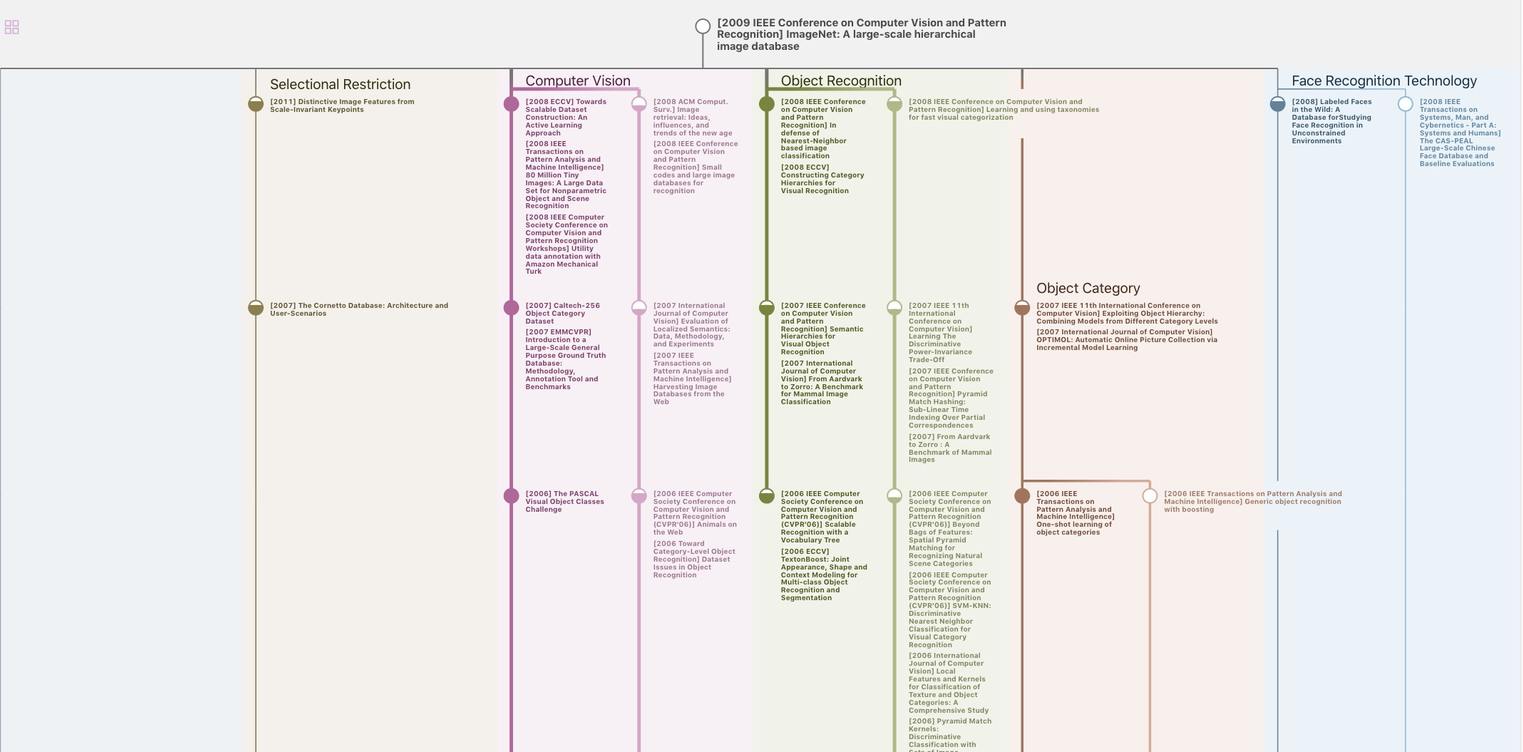
生成溯源树,研究论文发展脉络
Chat Paper
正在生成论文摘要