Improving Online POMDP Planning Algorithms with Decaying Q Value
2023 IEEE 35TH INTERNATIONAL CONFERENCE ON TOOLS WITH ARTIFICIAL INTELLIGENCE, ICTAI(2023)
Abstract
Online POMDP solvers search for the optimal policy based on multiple simulations. When scaling to large problems, more simulations typically lead to better results, but also more search time, thus it is necessary to make the best of finite simulations. Note that multiple simulations are not equivalent or independent, among which the earlier ones tend to sample randomly, while the later ones can take advantage of the previous results to better balance the exploration and exploitation. Moreover, there may be some possible environmental changes during the planning procedure. For these considerations, we allocate different weights to multiple simulations according to their order and propose a general Decaying Q Value (DQV) method to improve the existing online POMDP planning algorithms. We choose to improve POMCPOW, one of the state-of-the-art algorithms, to verify the effectiveness of the proposed method. Several experiments show that DQV can achieve competitive results on large-scale problems.
MoreTranslated text
Key words
POMDP,POMCP-DQV,sampling order
AI Read Science
Must-Reading Tree
Example
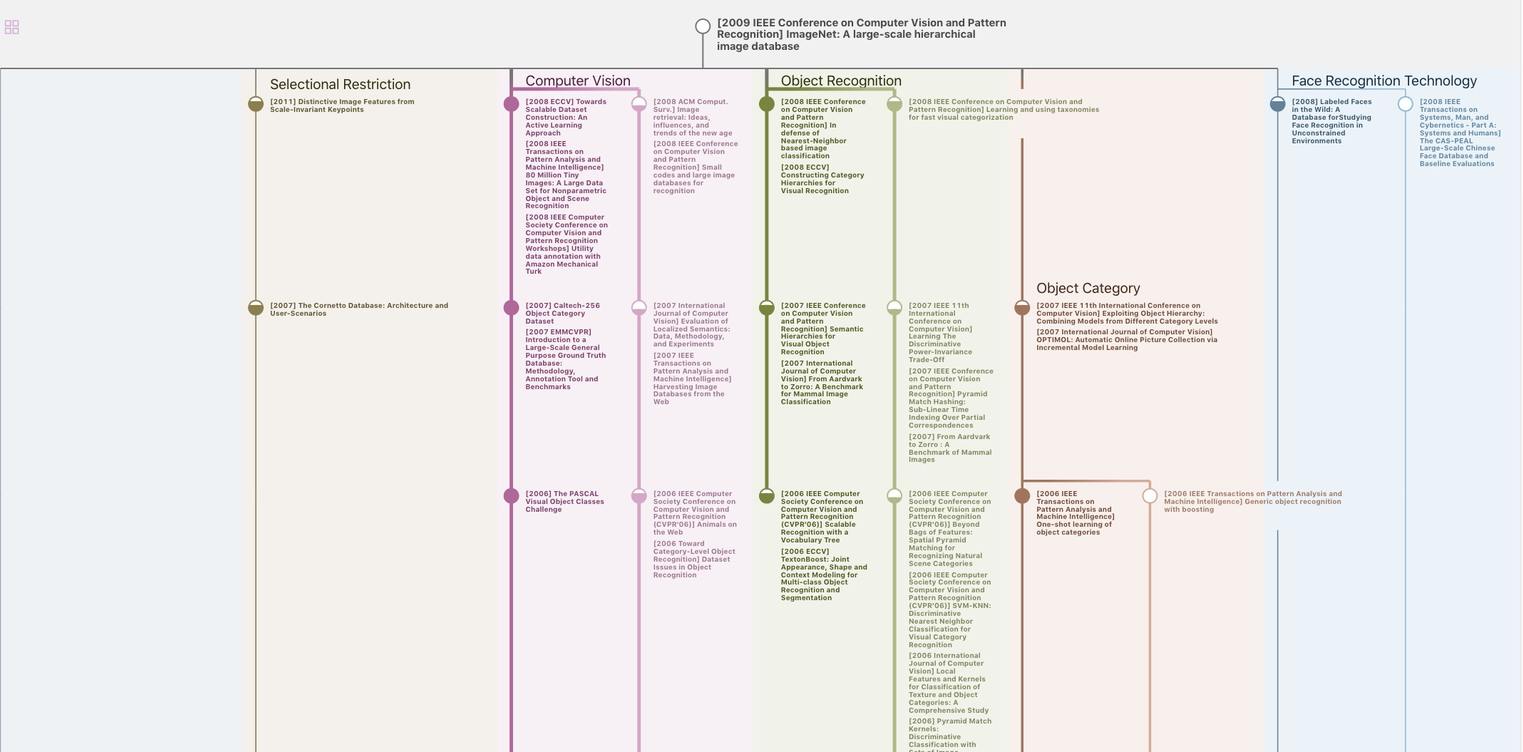
Generate MRT to find the research sequence of this paper
Chat Paper
Summary is being generated by the instructions you defined