Distortion Magnitude Control: A Dynamic Augmentation Optimization Approach for Few-Shot Learning in Remote Sensing Scene Classification
IEEE GEOSCIENCE AND REMOTE SENSING LETTERS(2024)
摘要
As a critical component of few-shot classification learning, feature augmentation methods have a direct bearing on classification performance. In the realm of few-shot classification tasks concerning remote sensing (RS) imagery, the instances of samples exhibiting relatively feeble features are commonplace. Employing conventional data augmentation approaches often falls short of achieving the desired classification outcomes. In response to such challenges, this letter introduces an optimization method for data augmentation in the context of few-shot RS image scene classification. We commence by analyzing the impact of distortion levels in data augmentation on classification performance. Then, we construct distortion-level spaces using different types of features and incorporate the distortion-level spaces into the model learning process using the support set, facilitating both model parameter optimization and dynamic optimization of distortion levels. The resulting optimized support data offer feature distortions of diverse types and magnitudes, thereby fortifying the feature robustness within the samples. We evaluate the proposed approach on widely used RS datasets, and the results demonstrate significant improvement in classification accuracy compared to the baseline. The introduction of this method offers a fresh perspective for data augmentation-based few-shot RS scene classification learning.
更多查看译文
关键词
Data augmentation,distortion magnitude,feature distortion,remote sensing (RS) scene classification
AI 理解论文
溯源树
样例
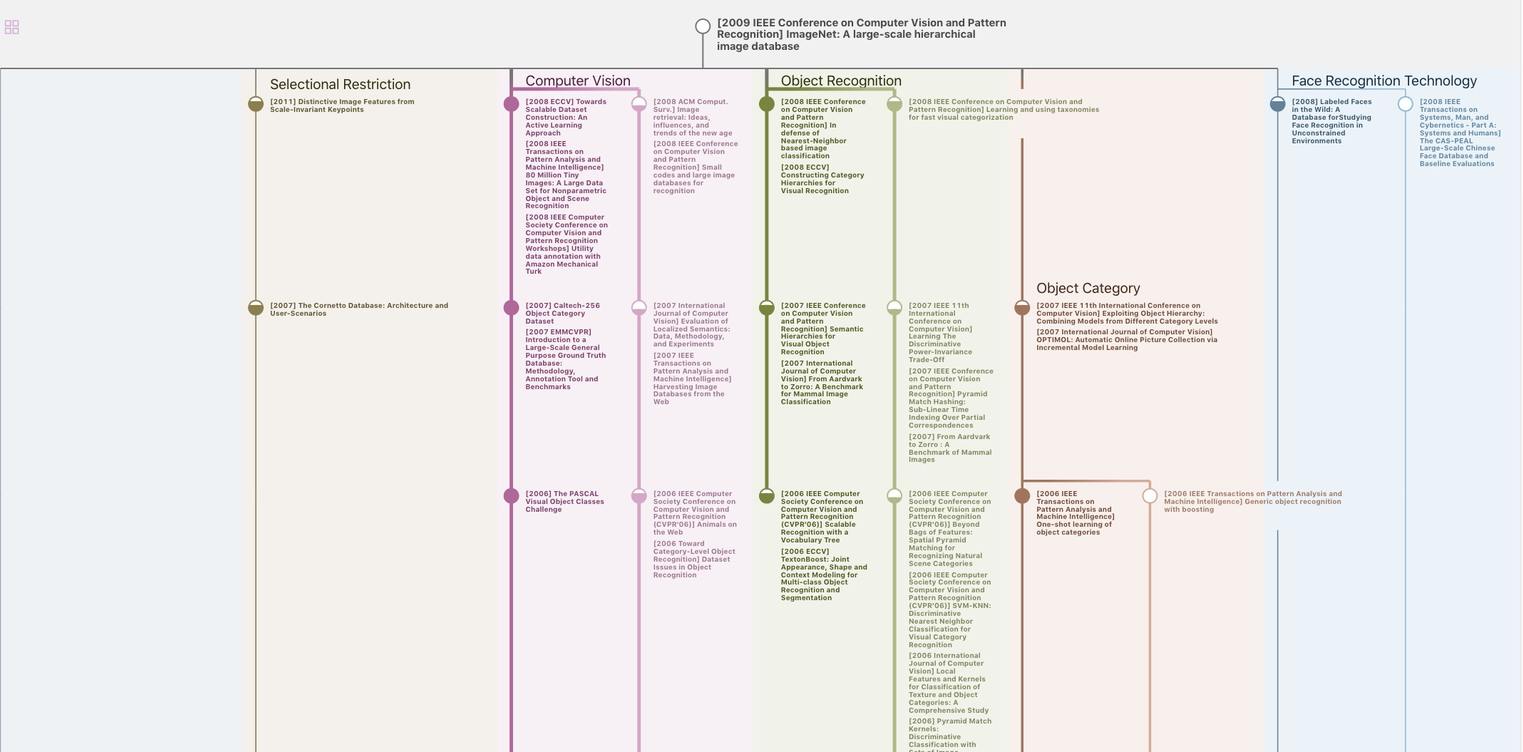
生成溯源树,研究论文发展脉络
Chat Paper
正在生成论文摘要