USING NETWORK ANALYSIS METHODS TO STUDY MULTIMORBIDITY PATTERNS
INNOVATION IN AGING(2023)
Key words
Multimorbidity,Patient Complexity,Network Analysis,Symptom Networks
AI Read Science
Must-Reading Tree
Example
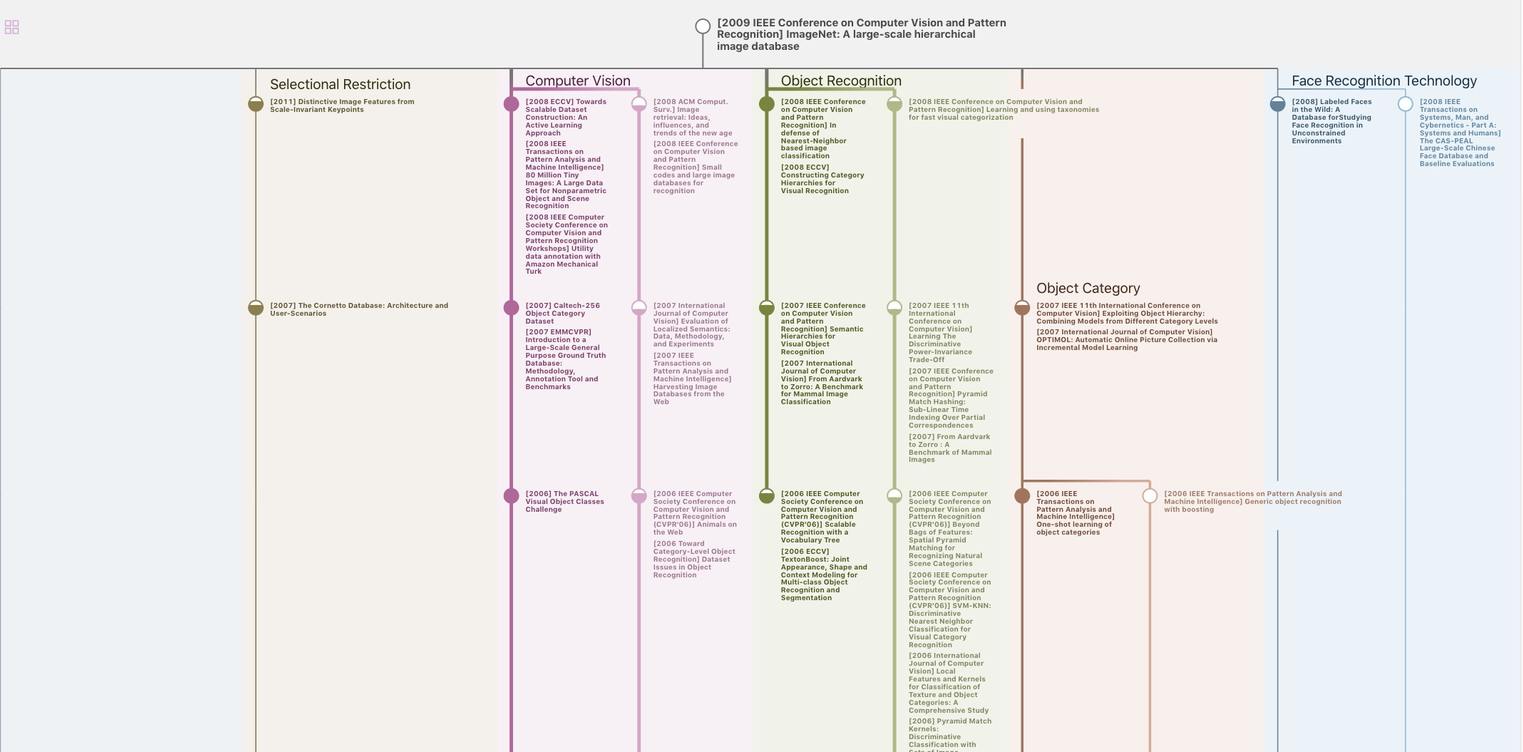
Generate MRT to find the research sequence of this paper
Chat Paper
Summary is being generated by the instructions you defined