Selecting essential factors for predicting reference crop evapotranspiration through tree-based machine learning and Bayesian optimization
Theoretical and Applied Climatology(2023)
摘要
Reference crop evapotranspiration (ETO) is a basic component of the hydrological cycle and its estimation is critical for agricultural water resource management and scheduling. In this study, three tree-based machine learning algorithms (random forest [RF], gradient boosting decision tree [GBDT], and extreme gradient boosting [XGBoost]) were adopted to determine the essential factors for ETO prediction. The tree-based models were optimized using the Bayesian optimization (BO) algorithm and were compared with three standalone models in terms of daily ETO and monthly mean ETO estimations in North China, with different input combinations of essential variables. The results indicated that solar radiation (Rs) and air temperature (Ts), including the maximum, minimum, and average temperatures, in daily ETO were the key variables affecting model prediction accuracy. Rs was the most influential factor in the monthly average ETO model followed by Ts. Both relative humidity (RH) and wind speed at 2 m (U2) had little impact on ETO prediction at different scales, although their importance differed. Compared with the GBDT and RF models, the XGBoost model exhibited the best performance for daily ETO and monthly mean ETO estimations. The hybrid tree-based models with the BO algorithm outperformed standalone tree-based models. Overall, compared with the other inputs, the model with three inputs (Rs, Ts, and RH/U2) had the highest accuracy. The BO-XGBoost model exhibited superior performance in terms of the global performance index (GPI) for daily ETO and monthly mean ETO predictions and is recommended as a more accurate model for predicting daily ETO and monthly mean ETO in North China or areas with a similar climate.
更多查看译文
关键词
Reference crop evapotranspiration,Essential factors,Tree-based algorithms,Machine learning,Bayesian optimization
AI 理解论文
溯源树
样例
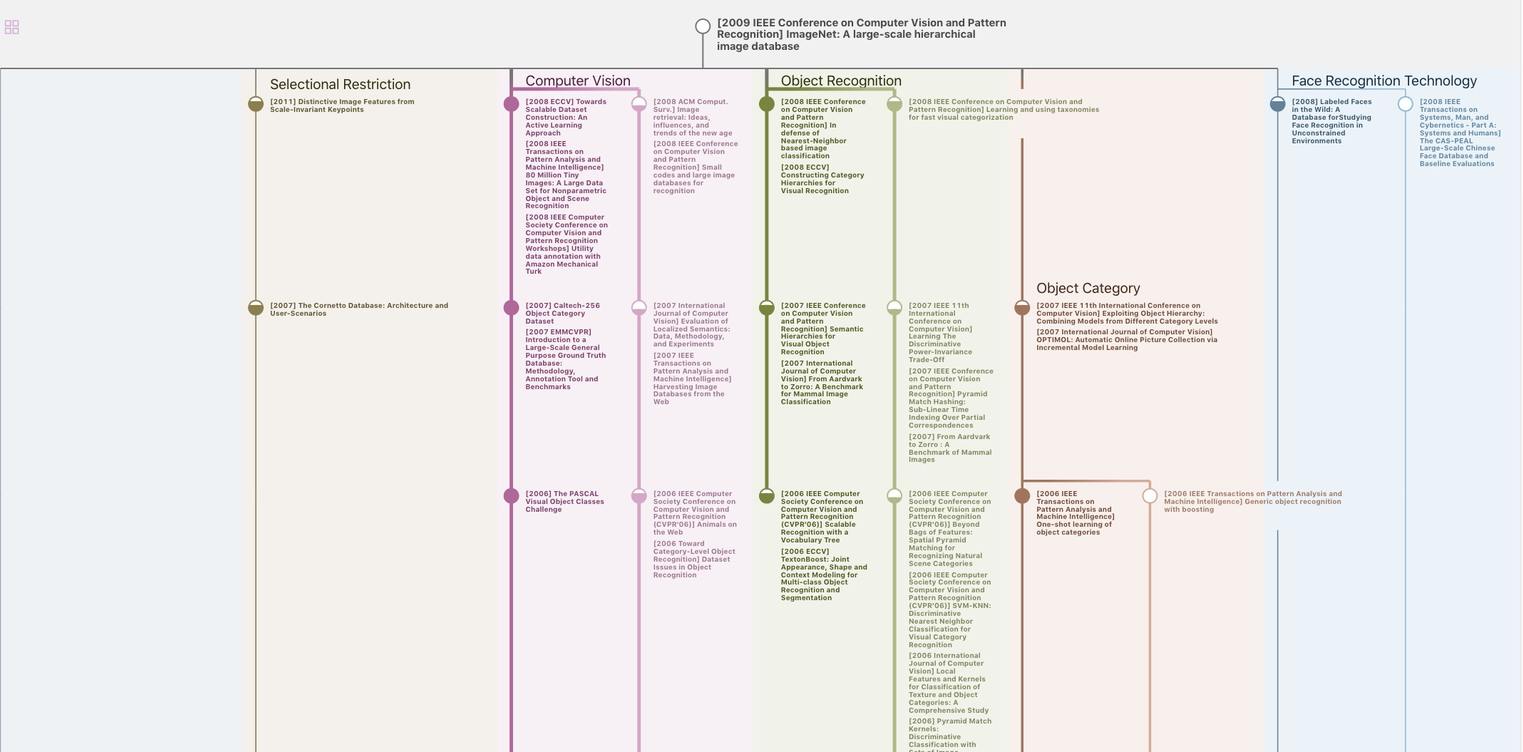
生成溯源树,研究论文发展脉络
Chat Paper
正在生成论文摘要