Wearable Smart Solution for Accurate Machine Learning-Based AF Detection Using ECG Signals
crossref(2023)
摘要
Atrial Fibrillation (AF) is known as one of the dominant cardiac disease that is associated with severe consequences such as risk of strokes. This cardiac arrhythmia can be efficiently diagnosed using EEG signals. However, AF episodes in EEG records are not always permanent for all subjects. In addition, lengthy subject preparation and ECG recording sessions makes this practice time consuming and not attractive for efficient diagnosis. One attractive solution for this problem will be the use of wearable Internet of Medical Things IoMT devices for EEG recording and AF detection. From another side the use machine learning for efficient diagnosis could provide high accurate diagnosis. However, the deployment of these wireless wearable devices faces the problems of limited processing capabilities and of energy scarcity. In this context, the design of energy efficient accurate on-sensor AF detection scheme using machine learning algorithms still requires a focused research effort. This paper proposes a wearable solution for ECG-based processing scheme for embedded AF detection. The proposed method achieved 100% sensitivity and 94.5% of specificity and accuracy of around 97.4 % for wide range of subjects while showing low energy-consumption. The obtained results show the efficiency of using Logistic Regression algorithm for accurate AF detection and attest about practical feasibility of the proposed approach in wearable IoMT devices.
更多查看译文
AI 理解论文
溯源树
样例
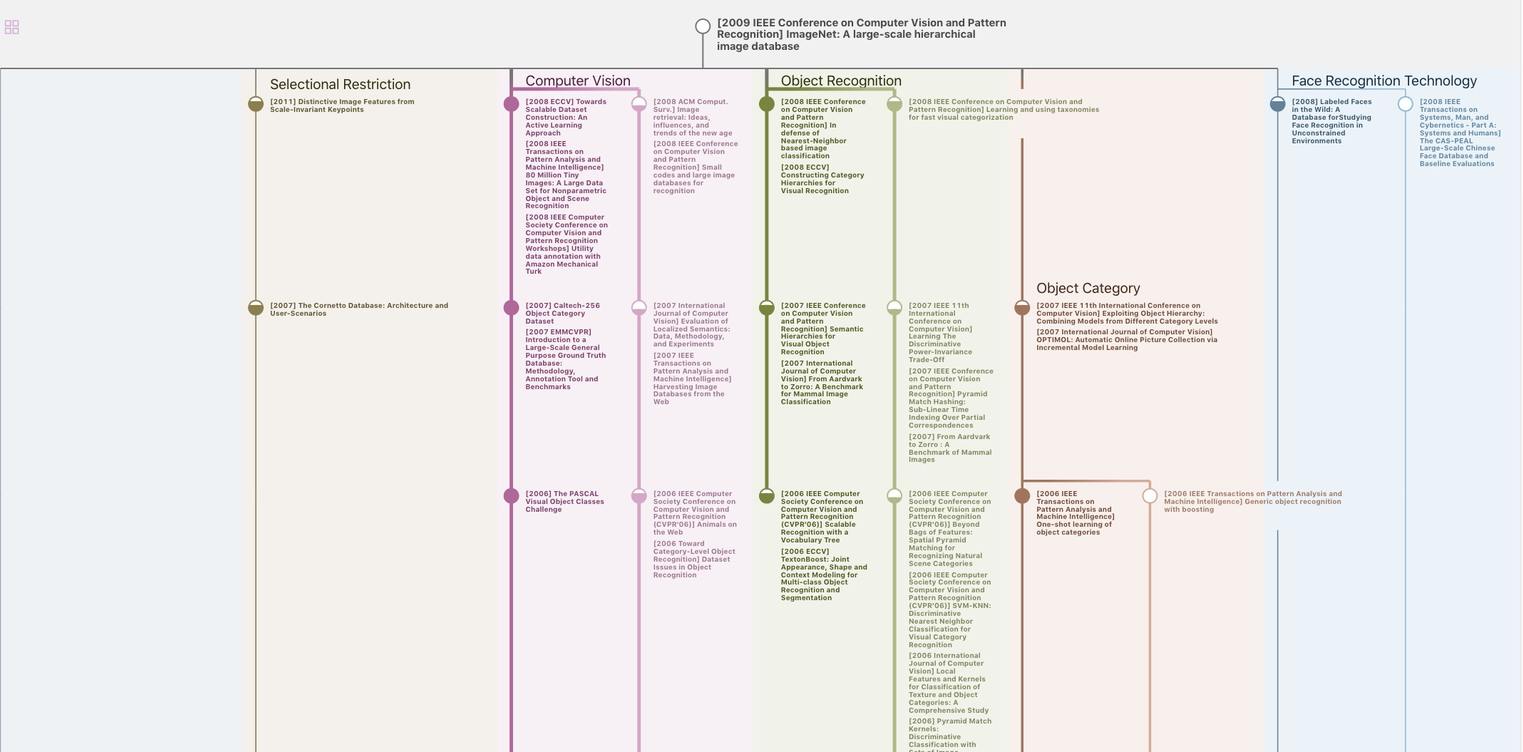
生成溯源树,研究论文发展脉络
Chat Paper
正在生成论文摘要