Physics-informed machine learning method with space-time Karhunen-Love expansions for forward and inverse partial differential equations
JOURNAL OF COMPUTATIONAL PHYSICS(2024)
摘要
We propose a physics -informed machine -learning method based on space -time -dependent Karhunen-Loeve expansions (KLEs) of the state variables and the residual least -square formulation of the solution of partial differential equations. This method, which we name dPICKLE, results in a reduced -order model for solving forward and inverse time -dependent partial differential equations. By conditioning KLEs on data, dPICKLE seamlessly assimilates data in forward and inverse solutions. KLEs are linear in unknown parameters. Because of this, and unlike physicsinformed deep -learning methods based on the residual least -square formulation, for well -posed partial differential equation (PDE) problems, dPICKLE leads to linear least -square problems (directly for linear PDEs and after linearization for nonlinear PDEs), which guarantees a unique solution. The efficiency and accuracy of dPICKLE are demonstrated for linear and nonlinear forward and inverse problems via comparison with analytical, finite difference, and physicsinformed neural network (PINN) solutions.
更多查看译文
关键词
Reduced-order models,Machine learning,Inverse methods,Space-time-dependent conditional,Karhunen-Loeve expansions
AI 理解论文
溯源树
样例
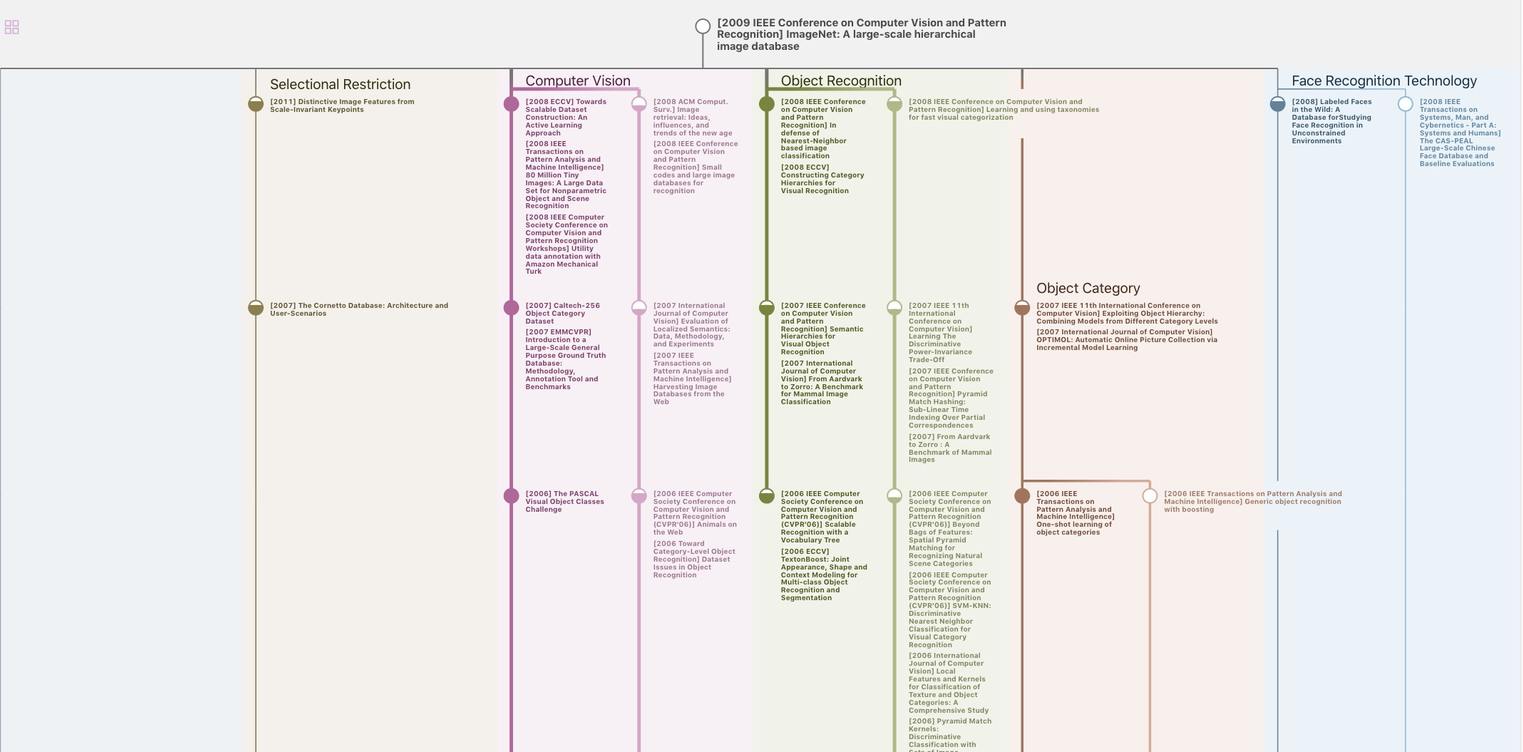
生成溯源树,研究论文发展脉络
Chat Paper
正在生成论文摘要