Metalearning with Very Few Samples Per Task
Annual Conference Computational Learning Theory(2023)
Abstract
Metalearning and multitask learning are two frameworks for solving a group of
related learning tasks more efficiently than we could hope to solve each of the
individual tasks on their own. In multitask learning, we are given a fixed set
of related learning tasks and need to output one accurate model per task,
whereas in metalearning we are given tasks that are drawn i.i.d. from a
metadistribution and need to output some common information that can be easily
specialized to new tasks from the metadistribution.
We consider a binary classification setting where tasks are related by a
shared representation, that is, every task P can be solved by a classifier of
the form f_P∘ h where h ∈ H is a map from features to a
representation space that is shared across tasks, and f_P∈ F is a
task-specific classifier from the representation space to labels. The main
question we ask is how much data do we need to metalearn a good representation?
Here, the amount of data is measured in terms of the number of tasks t that
we need to see and the number of samples n per task. We focus on the regime
where n is extremely small. Our main result shows that, in a
distribution-free setting where the feature vectors are in ℝ^d, the
representation is a linear map from ℝ^d →ℝ^k, and the
task-specific classifiers are halfspaces in ℝ^k, we can metalearn a
representation with error ε using n = k+2 samples per task, and
d · (1/ε)^O(k) tasks. Learning with so few samples per task
is remarkable because metalearning would be impossible with k+1 samples per
task, and because we cannot even hope to learn an accurate task-specific
classifier with k+2 samples per task. Our work also yields a characterization
of distribution-free multitask learning and reductions between meta and
multitask learning.
MoreTranslated text
AI Read Science
Must-Reading Tree
Example
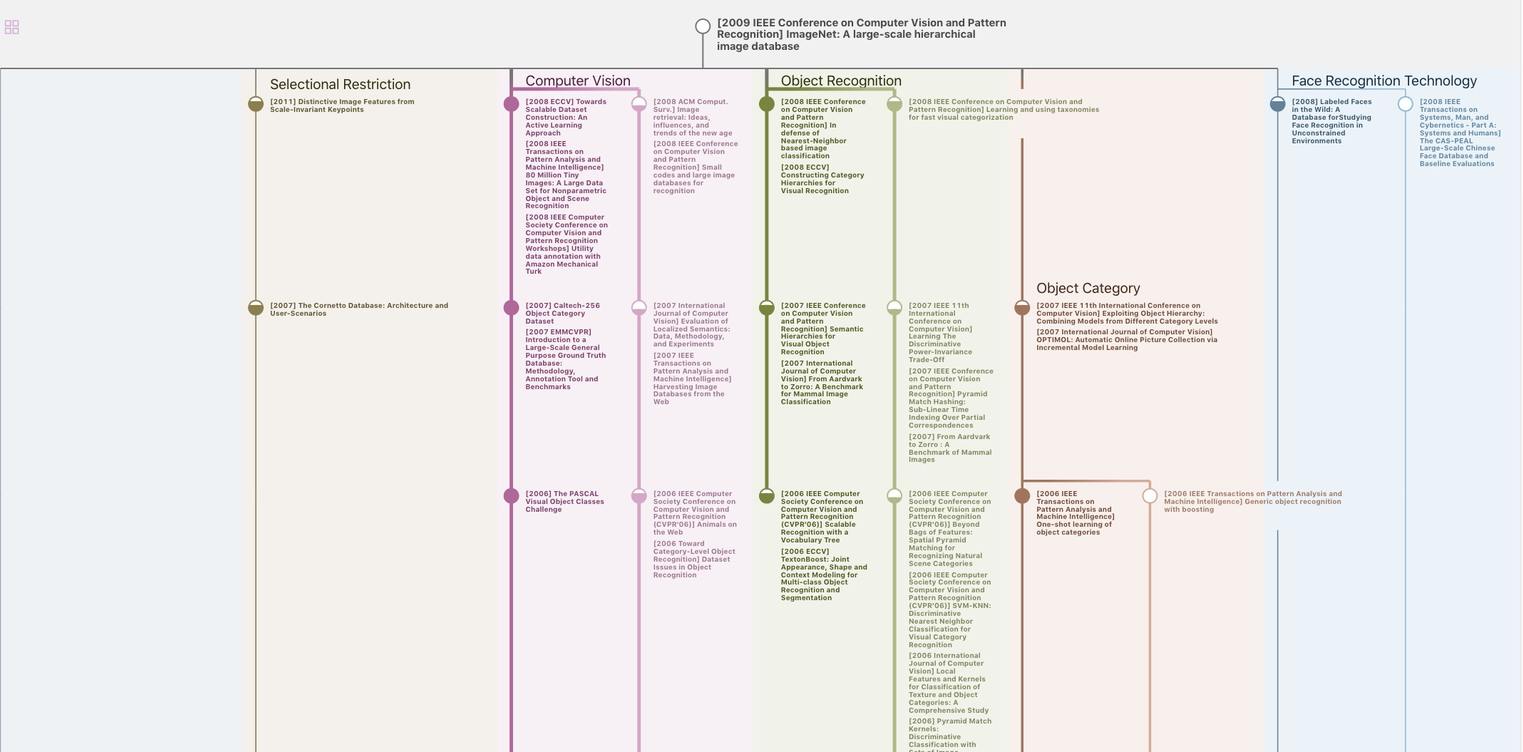
Generate MRT to find the research sequence of this paper
Chat Paper
Summary is being generated by the instructions you defined