Multimodal Federated Learning with Missing Modality via Prototype Mask and Contrast
CoRR(2023)
摘要
In real-world scenarios, multimodal federated learning often faces the
practical challenge of intricate modality missing, which poses constraints on
building federated frameworks and significantly degrades model inference
accuracy. Existing solutions for addressing missing modalities generally
involve developing modality-specific encoders on clients and training modality
fusion modules on servers. However, these methods are primarily constrained to
specific scenarios with either unimodal clients or complete multimodal clients,
struggling to generalize effectively in the intricate modality missing
scenarios. In this paper, we introduce a prototype library into the
FedAvg-based Federated Learning framework, thereby empowering the framework
with the capability to alleviate the global model performance degradation
resulting from modality missing during both training and testing. The proposed
method utilizes prototypes as masks representing missing modalities to
formulate a task-calibrated training loss and a model-agnostic uni-modality
inference strategy. In addition, a proximal term based on prototypes is
constructed to enhance local training. Experimental results demonstrate the
state-of-the-art performance of our approach. Compared to the baselines, our
method improved inference accuracy by 3.7% with 50% modality missing during
training and by 23.8% during uni-modality inference. Code is available at
https://github.com/BaoGuangYin/PmcmFL.
更多查看译文
AI 理解论文
溯源树
样例
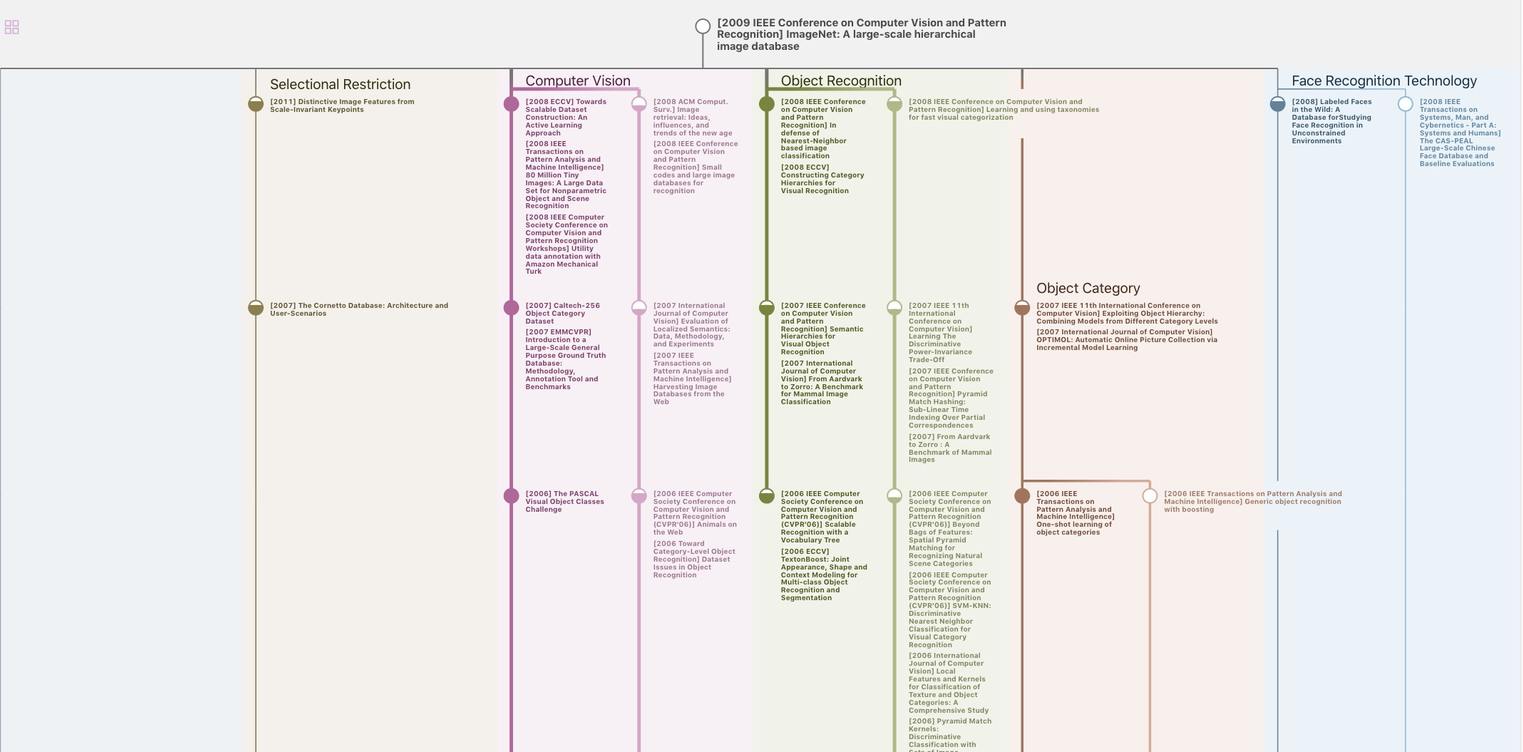
生成溯源树,研究论文发展脉络
Chat Paper
正在生成论文摘要