Imaging of regional lung function by capacitively coupled electrical impedance tomography and image reconstruction using deep neural network.
2023 IEEE International Conference on Imaging Systems and Techniques (IST)(2023)
Abstract
Electrical tomography is a low-cost and noninvasive technique for visualizing the internal structure of an object. It relies on the components' distinct electrical or electromagnetic properties in a measurement volume. A capacitively coupled impedance electrical tomography (CCEIT) is a new contactless EIT technique that uses voltage excitation without direct contact with the skin. The introduction of CCEIT allows us to overcome one of the most challenging issues of conventional EIT, such as variable electrode-skin impedance, which negatively affects measurement accuracy. This work is devoted to using artificial neural networks for image reconstruction in capacitively coupled impedance electrical tomography. The work focuses on the robustness comparison of two different architectures of artificial networks: linear network and conditional generative adversarial network (cGAN). Evaluation of networks was done on numerical simulation of chest and lungs phantom, having healthy and illness-affected lungs simulated. The thorax phantom, which includes the heart, aorta, spine, and lungs, was elaborated. The sensor with 32 area electrodes was used. The ECTsim custom-designed toolbox for Matlab was used to generate the training data sets. Two kinds of illnesses: pneumothorax and pleural effusion were assessed. The results showed that it is possible to obtain information about regional lung function using ANN-based image reconstruction.
MoreTranslated text
Key words
Electrical impedance tomography,neural networks,image reconstruction,inverse problem,cGAN,numerical simulation
AI Read Science
Must-Reading Tree
Example
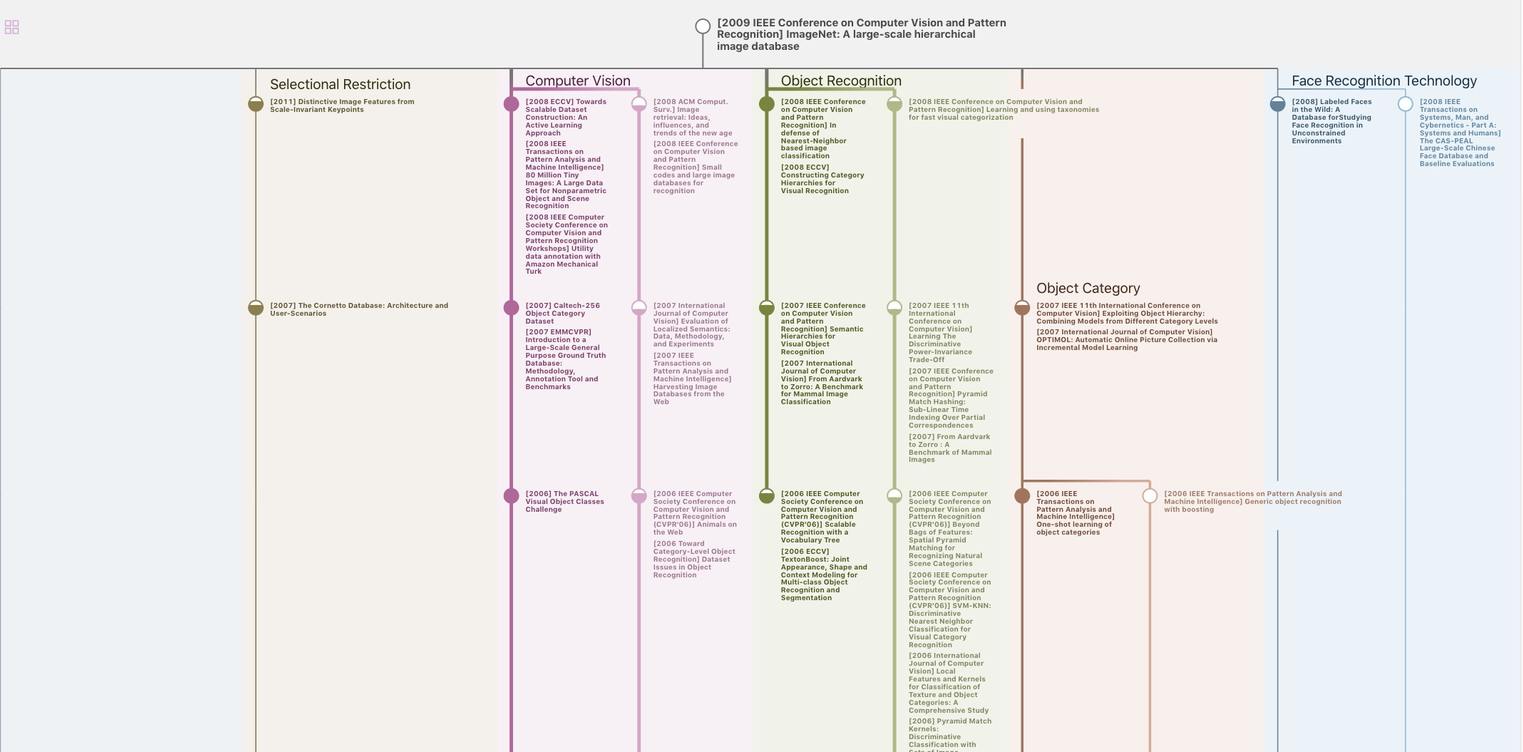
Generate MRT to find the research sequence of this paper
Chat Paper
Summary is being generated by the instructions you defined