DCGAN-based image enhancement in electrical capacitance tomography.
2023 IEEE International Conference on Imaging Systems and Techniques (IST)(2023)
Abstract
Electrical capacitance tomography (ECT) is a technique to visualize the cross-sectional permittivity distribution in the sensing domain from inter-electrode capacitance measurements on the domain boundary. Conventional image reconstruction methods suffer from the low spatial resolution, as ECT inverse problem is inherently nonlinear, ill-conditioned and ill-posed. Deep learning methods has made considerable achievements and has great potential in ameliorating the reconstruction quality of ECT. Generative adversarial network (GAN) is a typical framework developed in the field of deep learning in recent years to solve image processing problems with ill-posed nature. This paper proposed a novel method based on deep convolutional generation adversarial networks (DCGAN) to enhance image quality of reconstructed images, especially for accurate shape reconstruction. The proposed method can abstract the mapping information from the low-resolution images reconstructed by a conventional reconstruction algorithm to the high-resolution target images, and then recover high-resolution images through an adversarial learning process. In this paper, the low-quality images reconstructed by the Landweber iterative algorithm are used as input for the framework of the proposed method. Numerical results validate the superiority of the DCGAN-based method in the enhancement of the ECT images, i.e., giving more accurate reconstructions of complex-shaped inclusions with lower image errors than Landweber iterative algorithm.
MoreTranslated text
Key words
Electrical capacitance tomography,image enhancement,deep learning,generative adversarial networks
AI Read Science
Must-Reading Tree
Example
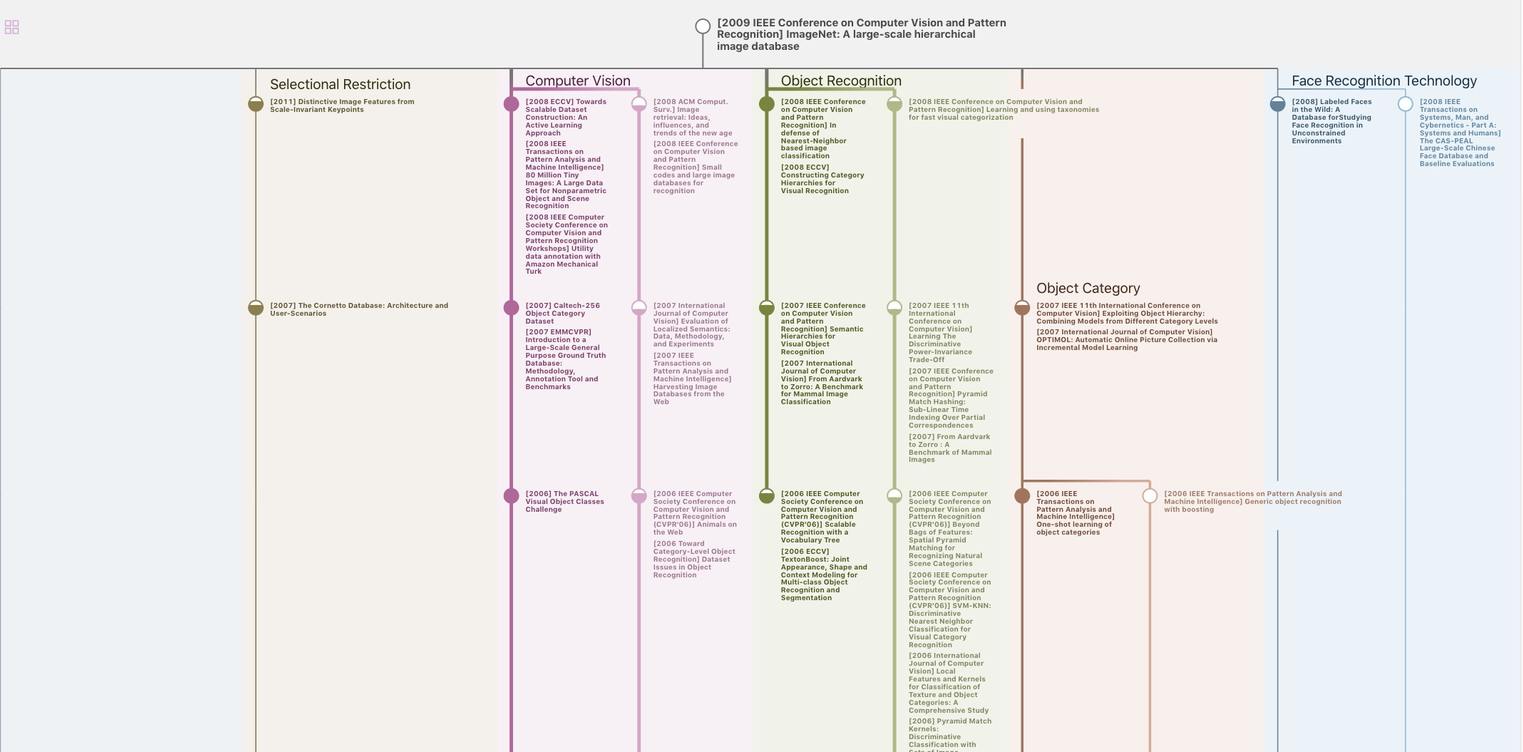
Generate MRT to find the research sequence of this paper
Chat Paper
Summary is being generated by the instructions you defined