Enhancing Change Detection in Spectral Images: Integration of UNet and ResNet Classifiers
2023 IEEE 35TH INTERNATIONAL CONFERENCE ON TOOLS WITH ARTIFICIAL INTELLIGENCE, ICTAI(2023)
摘要
Image change detection in remote sensing is crucial for monitoring environmental changes at different temporal and spatial scales. The primary goal is to identify altered pixels in multi-temporal images accurately. However, challenges such as response latency and limited large-scale validation persist. In this study, we propose an accurate automated change detection method called "ResUNet" based on multi-spectral NDVI imagery. ResUNet combines UNet and residual networks, while employing deep learning-based features for precise change detection. We evaluate the proposed method on low-resolution data from three geographical regions: Colombia, California, and Duluth. Each region comprises 145,161 patches, ensuring comprehensive coverage for experimentation. We validate our method in three distinct areas, achieving an accuracy of 99.50% and an F1-score of 99.41%.
更多查看译文
关键词
Change detection,Fusion,Deep learning,CNN,ResNet,Shearlet transform,Multispectral images
AI 理解论文
溯源树
样例
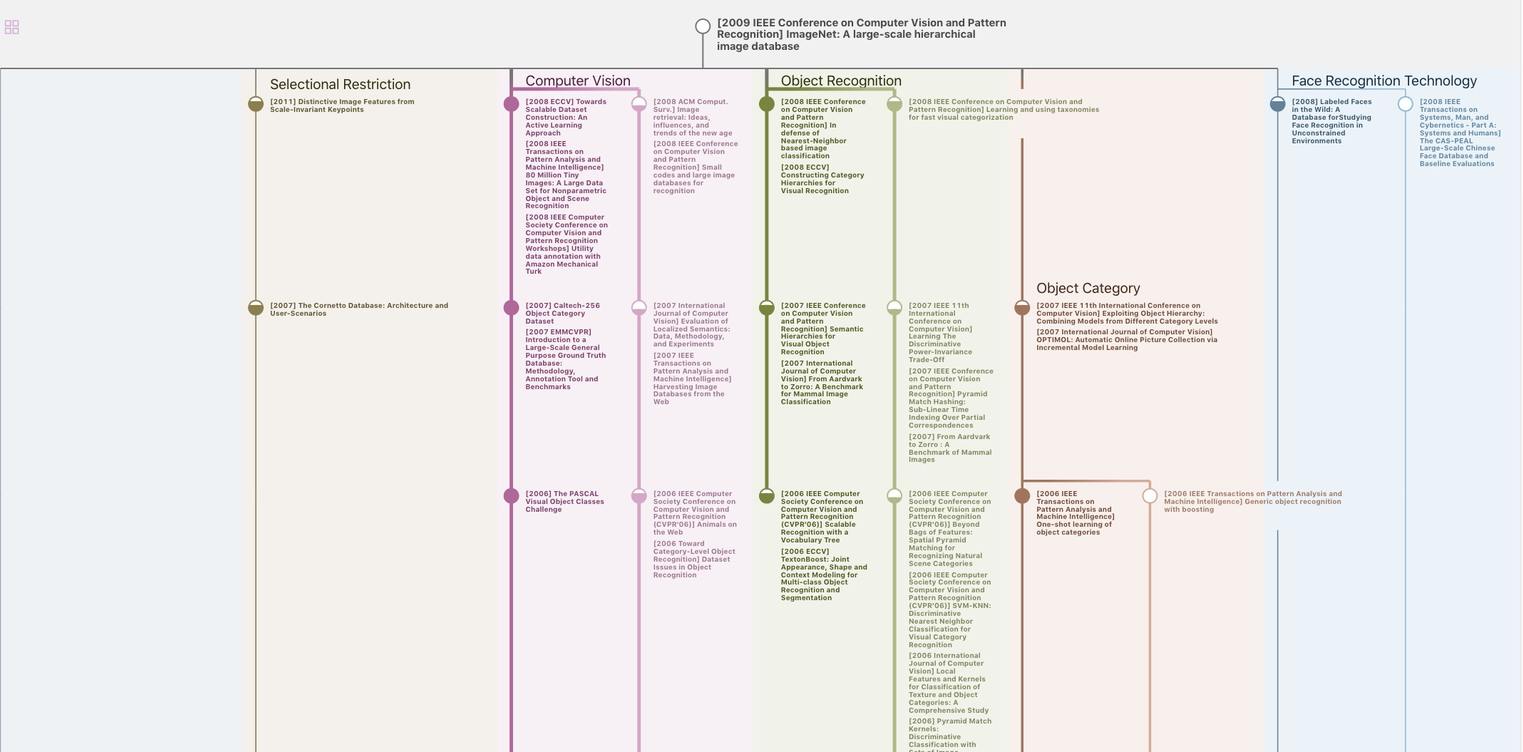
生成溯源树,研究论文发展脉络
Chat Paper
正在生成论文摘要