On Data Selection for the Energy Efficiency of Neural Networks: Towards a New Solution Based on a Dynamic Selectivity Ratio.
2023 IEEE 35TH INTERNATIONAL CONFERENCE ON TOOLS WITH ARTIFICIAL INTELLIGENCE, ICTAI(2023)
Abstract
In this paper, we address the energy efficiency of neural networks training through data selection techniques. We first study the impact of a random data selection approach that renews the selected examples periodically during training. We find that random selection should be considered as a serious option as it allows high energy gains with small accuracy losses. Unexpectedly, it even outperforms a more elaborate approach in some cases.Our study of the random approach conducted us to observe that low selectivity ratios allow important energy savings, but also cause a significant accuracy decrease. To mitigate the effect of such ratios on the prediction quality, we propose to use a dynamic selectivity ratio with a decreasing schedule, that can be integrated to any selection approach. Our first results show that using such a schedule provides around 60% energy gains on the CIFAR-10 dataset with less than 1% accuracy decrease. It also improves the convergence when compared to a fixed ratio.
MoreTranslated text
Key words
Neural networks,random data selection,dynamic selectivity ratio,energy efficiency.
AI Read Science
Must-Reading Tree
Example
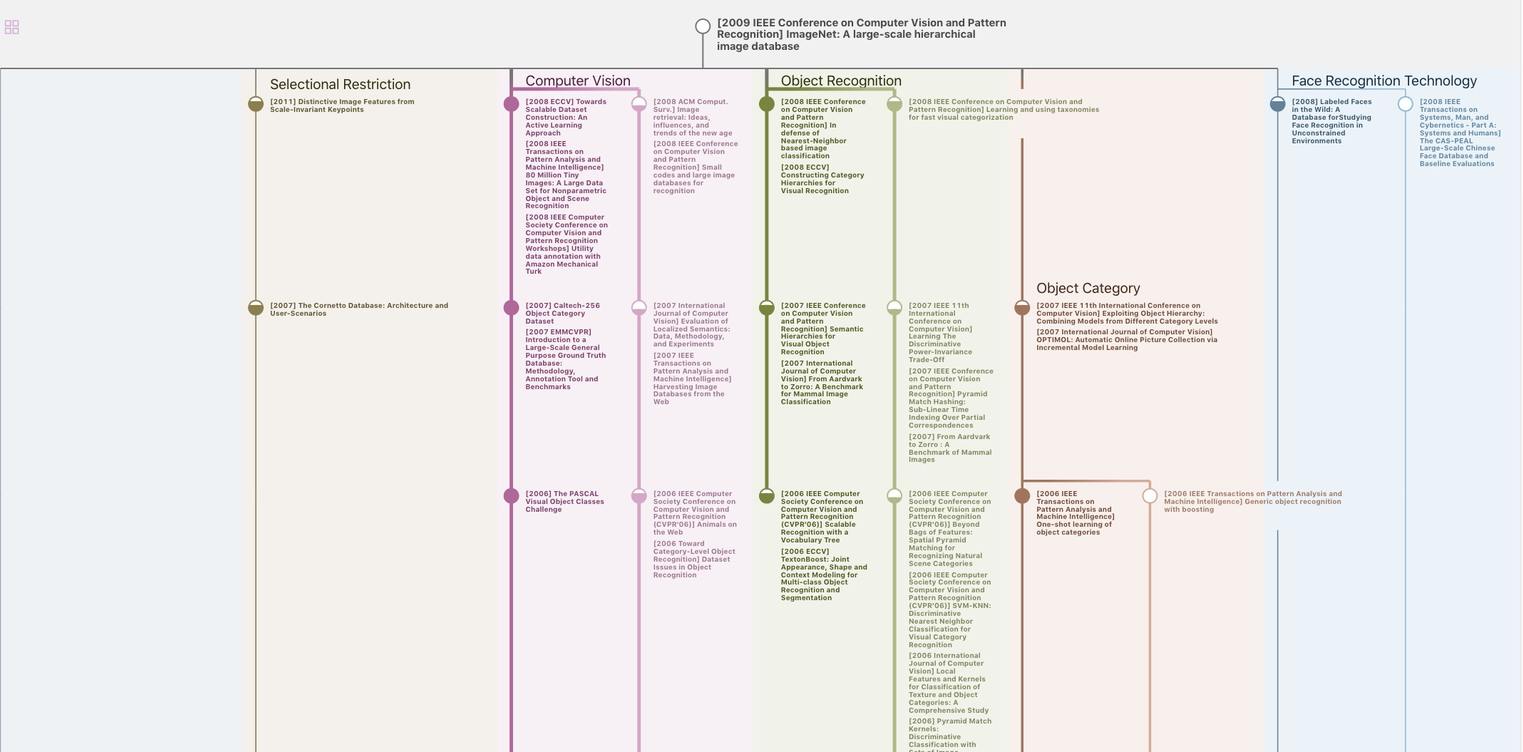
Generate MRT to find the research sequence of this paper
Chat Paper
Summary is being generated by the instructions you defined