Hybrid CNN-LSTM and Domain Modeling in Climate-Energy Analysis for a Smart Environment
2023 IEEE 35TH INTERNATIONAL CONFERENCE ON TOOLS WITH ARTIFICIAL INTELLIGENCE, ICTAI(2023)
摘要
This study aims to model the effect of local climate on energy generation in power plants in the United States. It considers climate change as well as the ever-growing electricity demand, contributing to greenhouse gas emissions. We propose an integration of AI-based and domain-specific paradigms via a hybrid approach of convolutional neural networks (CNN) & long short-term memory (LSTM) coupled with environmental models. Erstwhile studies focus mainly on specific energy sources, e.g. solar/wind power. There is a need for more comprehensive examination, e.g. temperature effects across various energy generation types at the macro level of states and micro level of power plants. This forms the major focus and novelty of our study. Fathoming the factors driving temperature-energy correlations, and assessing power plant vulnerability to changing climate patterns, are both essential to promote energy infrastructure resilience and sustainability. Our study offers novel insights into the complex interplay of temperature, energy generation, sustainability and related aspects to enable enhanced macro & micro level decision-making in energy sector. It therefore makes contributions to smart environment goals for smart cities and a smart planet.
更多查看译文
关键词
CNN,Climate Studies,Electricity,Energy,LSTM,Predictive Modeling,Smart Environment,Smart Cities
AI 理解论文
溯源树
样例
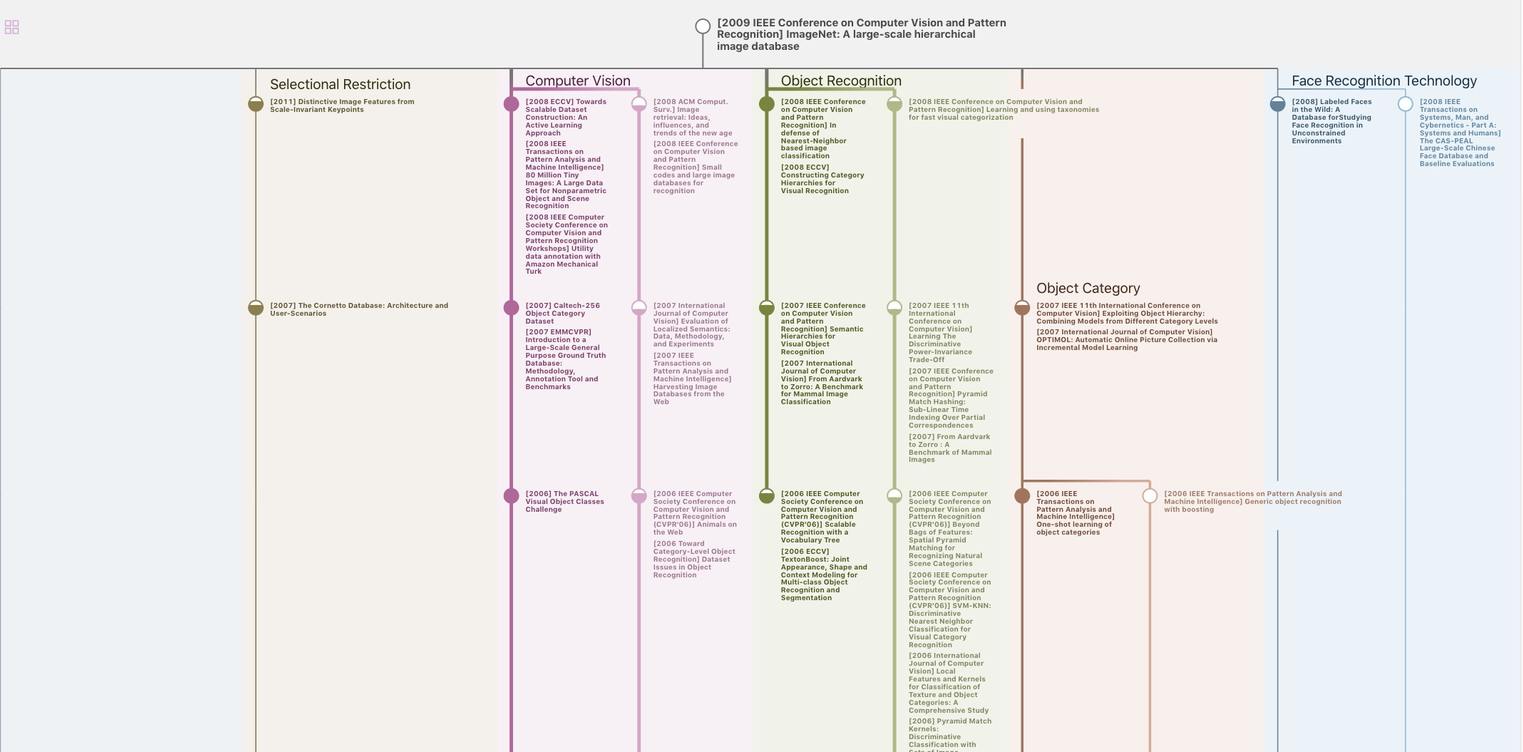
生成溯源树,研究论文发展脉络
Chat Paper
正在生成论文摘要