What Really Matters for Graph Contrastive Learning-Based Recommendations? A Unified Learning Strategy
2023 IEEE 35TH INTERNATIONAL CONFERENCE ON TOOLS WITH ARTIFICIAL INTELLIGENCE, ICTAI(2023)
Abstract
Graph contrastive learning (GCL) has become increasingly popular in recommendation due to its remarkable ability to reduce reliance on labels. Typically, GCL employs data augmentation methods, e.g., structure perturbation and representation perturbation, and CL loss to enhance performance. Recent studies have shown that structural perturbation plays a minor role in GCL, but there is still a lack of exploration on the representation perturbation. Therefore, we compare the two data perturbations in detail and reveal that both of them have a limited impact on performance. Simply combining recommendation loss and CL loss can produce comparable improvements. Besides, we identify a shared principle between the designs of recommendation loss and CL loss: both aim to optimize representation by increasing similarity between a target node and its positive samples while decreasing similarity with negative samples. Based on these findings, we propose Compact Graph Contrastive Learning (CGCL), a streamlined strategy that eliminates the data augmentation and deeply unifies recommendation loss and CL loss by elegantly incorporating their respective contributions. Leveraging the benefits of the unified strategy, we discover that our model can learn a concentrated mode length distribution of representation, which can enhance the ability to debias and thus improve the performance of the recommendation. This is a novel perspective on representation learning, and we also validate its rationality through rigorous experiments. Our comprehensive study on multiple benchmark datasets demonstrates that CGCL outperforms existing GCL methods.
MoreTranslated text
Key words
contrastive learning,recommendation,representation learning
AI Read Science
Must-Reading Tree
Example
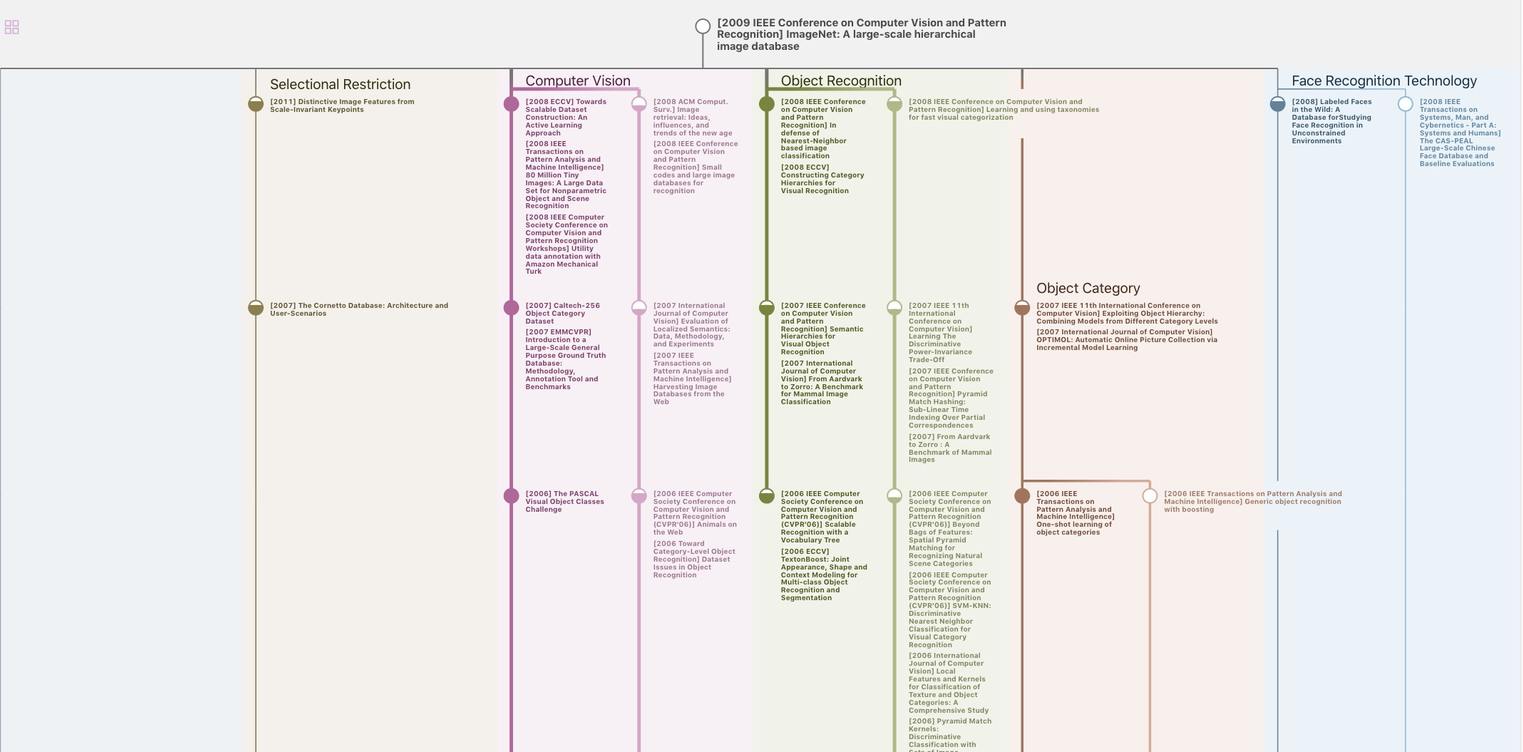
Generate MRT to find the research sequence of this paper
Chat Paper
Summary is being generated by the instructions you defined