RSMS: Robust Semi-supervised Multi-label Feature Selection for Regression
2023 IEEE 35TH INTERNATIONAL CONFERENCE ON TOOLS WITH ARTIFICIAL INTELLIGENCE, ICTAI(2023)
摘要
Feature selection is a very important part of successful data mining applications, because it contributes to the simplification and explainability of the resulting model through dimensionality reduction. Feature selection methods exist for semi-supervised scenarios, in which a small subset of labeled instances is combined with a large batch of unlabeled instances to improve the learning performance. In a multi-label setting, feature selection identifies important features for all labels at once. However, some labels can also be irrelevant and then deteriorate feature selection. In a robust learning framework, removing these noisy labels can decrease the generalization error for the resulting regression model. In this work, we present a novel algorithm performing multi-label semi-supervised feature selection and label seleciton, and show its effectiveness on some publicly available datasets.
更多查看译文
关键词
Feature selection,Semi-supervised learning,Multi-label learning,Regression
AI 理解论文
溯源树
样例
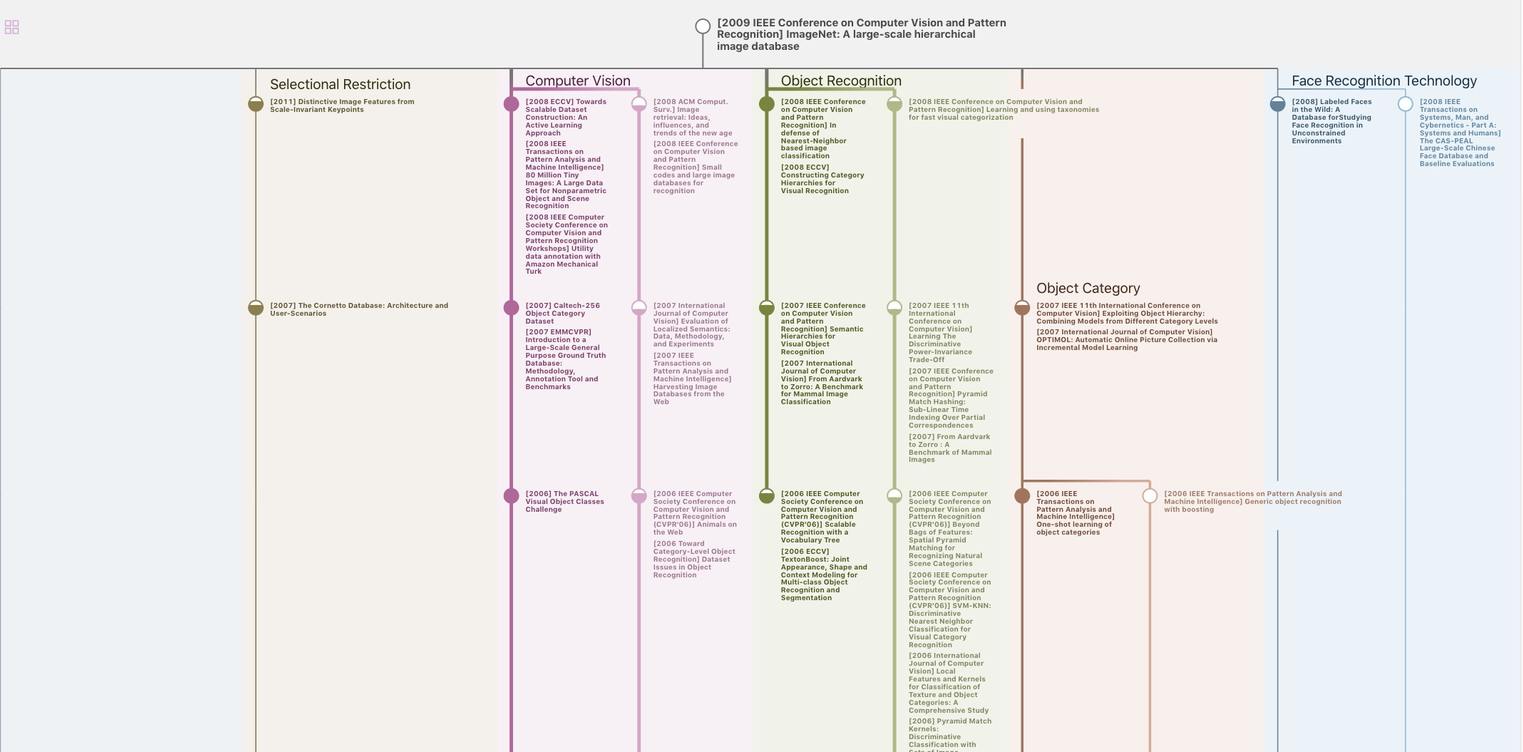
生成溯源树,研究论文发展脉络
Chat Paper
正在生成论文摘要