Performance and Energy Savings Trade-Off with Uncertainty-Aware Cloud Workload Forecasting
2023 IEEE 31ST INTERNATIONAL CONFERENCE ON NETWORK PROTOCOLS, ICNP(2023)
摘要
Cloud managers typically leverage future workload predictions to make informed decisions on resource allocation, where the ultimate goal of the allocation is to meet customers' demands while reducing the provisioning cost. Among several workload forecasting approaches proposed in the literature, uncertainty-aware time series analysis solutions are desirable in cloud scenarios because they can predict the distribution of future demand and provide bounds associated with a given service level set by the resource manager. The effectiveness of uncertainty based workload predictions is normally assessed in terms of accuracy metrics (e.g. MAE) and service level (e.g. Success Rate), but the effect on the resource provisioning cost is under-investigated. We propose an evaluation framework to assess the impact of uncertainty-aware predictions on the performance vs cost trade-off, where we express the cost in terms of energy savings. We illustrate the framework's effectiveness by simulating two real-world cloud scenarios where an optimizer leverages workload predictions to allocate resources to satisfy a desired service level while minimizing energy waste. Offline experiments compare representative uncertainty-aware models and a new model (HBNN++) that we propose, which predict a cluster trace's GPU demand. We show that more effective uncertainty modelling can save energy without violating desired service level targets and that model performance varies depending on the specific details of the allocation scheme, server and GPU energy costs.
更多查看译文
关键词
Cloud Computing,Energy Saving,Deep Learning,Workload Prediction,Uncertainty,Time Series Forecasting
AI 理解论文
溯源树
样例
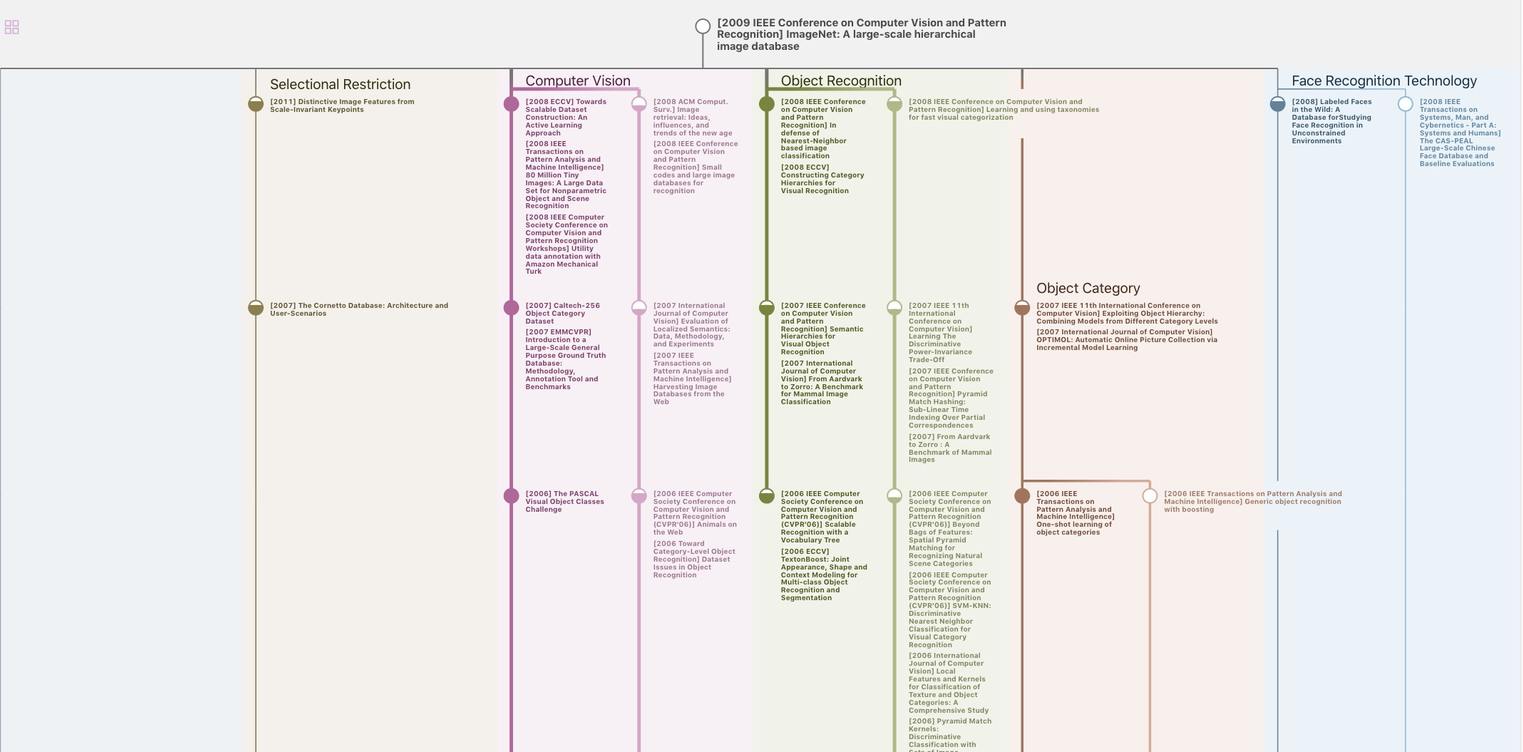
生成溯源树,研究论文发展脉络
Chat Paper
正在生成论文摘要