Vertical Attention-Based Siamese ConvLSTM Network for Argo Data Error Detection
IEEE TRANSACTIONS ON GEOSCIENCE AND REMOTE SENSING(2024)
关键词
Abnormal data oversampling,array for real-time geostrophic oceanography (Argo) observation data,error detection,siamese convolutional long--short-term memory (ConvLSTM),vertical attention
AI 理解论文
溯源树
样例
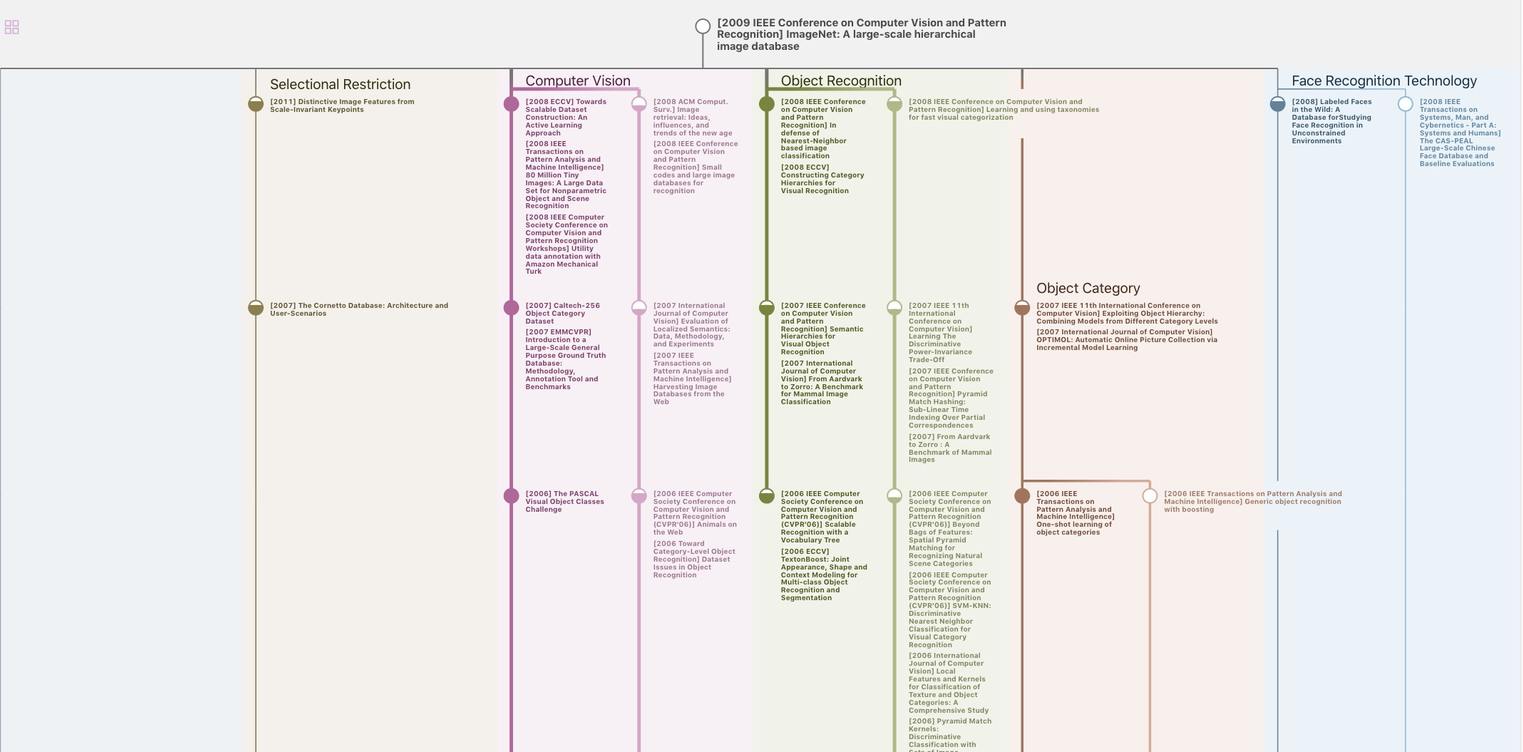
生成溯源树,研究论文发展脉络
Chat Paper
正在生成论文摘要