Global-Group Attention Network With Focal Attention Loss for Aerial Scene Classification
IEEE TRANSACTIONS ON GEOSCIENCE AND REMOTE SENSING(2024)
摘要
Aerial scene classification, aiming at assigning a specific semantic class to each aerial image, is a fundamental task in the remote sensing community. Aerial scene images have more diverse and complex geological features. While some statistics of images can be well fit using convolution, it limits such models to capturing the global context hidden in aerial scenes. Furthermore, to optimize the feature space, many methods add class information to the feature embedding space. However, they seldom combine model structure with class information to obtain more separable feature representations. In this article, we propose to address these limitations in a unified framework (i.e., CGFNet) from two aspects: focusing on the key information of input images and optimizing the feature space. Specifically, we propose a global-group attention module (GGAM) to adaptively learn and selectively focus on important information from input images. GGAM consists of two parallel branches: the adaptive global attention branch (AGAB) and the region-aware attention branch (RAAB). AGAB utilizes an adaptive pooling operation to better model the global context in aerial scenes. As a supplement to AGAB, RAAB combines grouping features with spatial attention to spatially enhance the semantic distribution of features (i.e., selectively focus on effective regions of features and ignore irrelevant semantic regions). In parallel, a focal attention loss (FA-Loss) is exploited to introduce class information into attention vector space, which can improve intraclass consistency and interclass separability. Experimental results on four publicly available and challenging datasets demonstrate the effectiveness of our method.
更多查看译文
关键词
Aerial scene classification,attention,convolutional neural networks (CNNs),loss function,remote sensing
AI 理解论文
溯源树
样例
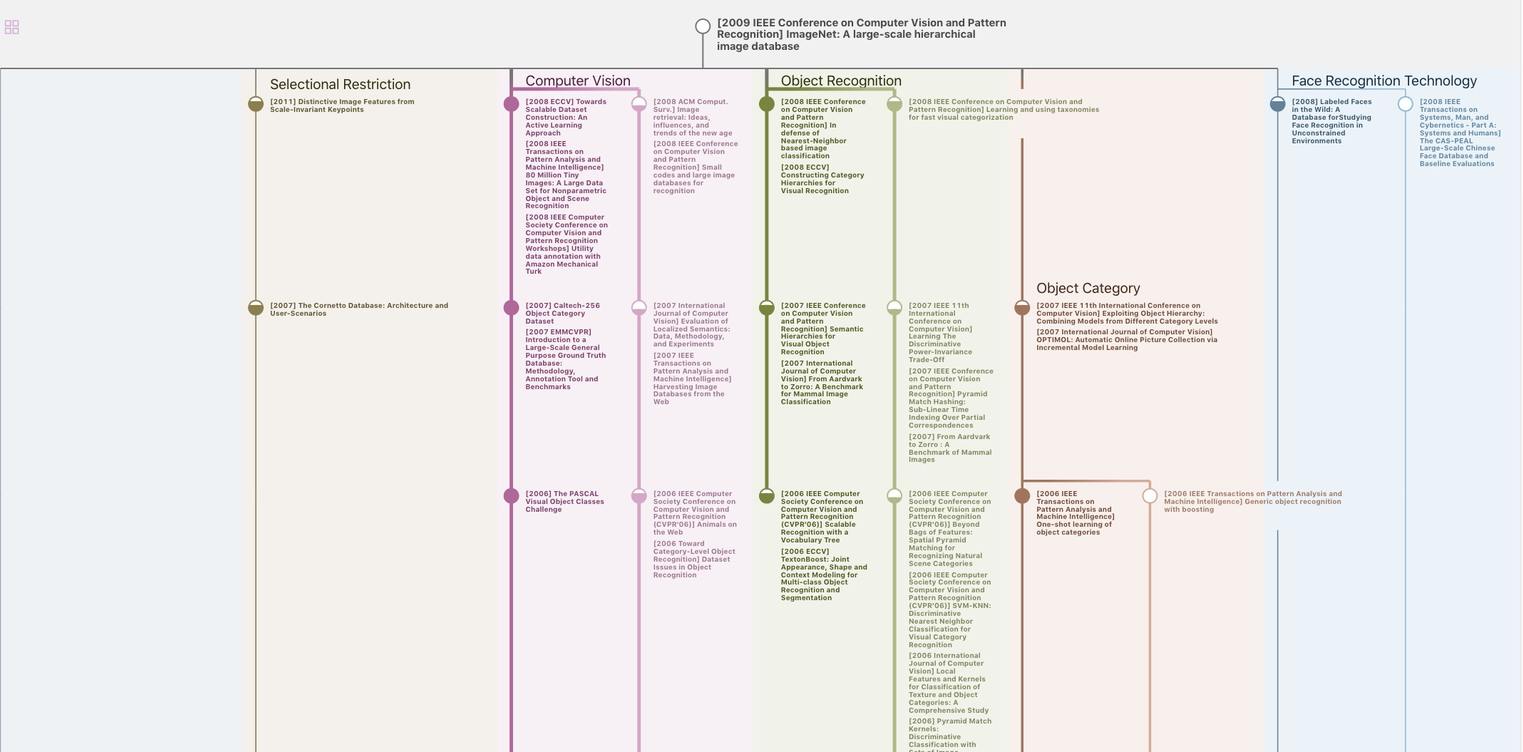
生成溯源树,研究论文发展脉络
Chat Paper
正在生成论文摘要