On the understanding of bubble dynamics through a calibrated textile-like porous medium using a machine learning based algorithm
COMPOSITES PART A-APPLIED SCIENCE AND MANUFACTURING(2024)
摘要
In this work, we present a proof of concept for both 3D-printed media and a machine learning analysis methodology to investigate void formation and transport during liquid filling. Through a series of experiments, we characterized void formation and transport at constant flow rates using two calibrated fabric-like porous geometries created by Stereolithography and Multi Jet Fusion 3D printing techniques. Our findings highlight the significance of porous medium geometry and void size, in addition to the capillary number, in characterizing void formation and mobility during resin flow into a mold containing a fibrous preform. Notably, the paper's strength lies in the presentation of advanced bubble analysis methods, including frame-by-frame high-resolution video analysis, enabling the identification of individual bubbles and the extraction of their statistics, such as count, size, and velocity throughout the experiment. These insights contribute to the design of more efficient processes, resulting in composite parts with reduced void content.
更多查看译文
关键词
E: Liquid Composite Moulding,B: Defects,E: 3-D Printing,B: Porosity,B: Permeability
AI 理解论文
溯源树
样例
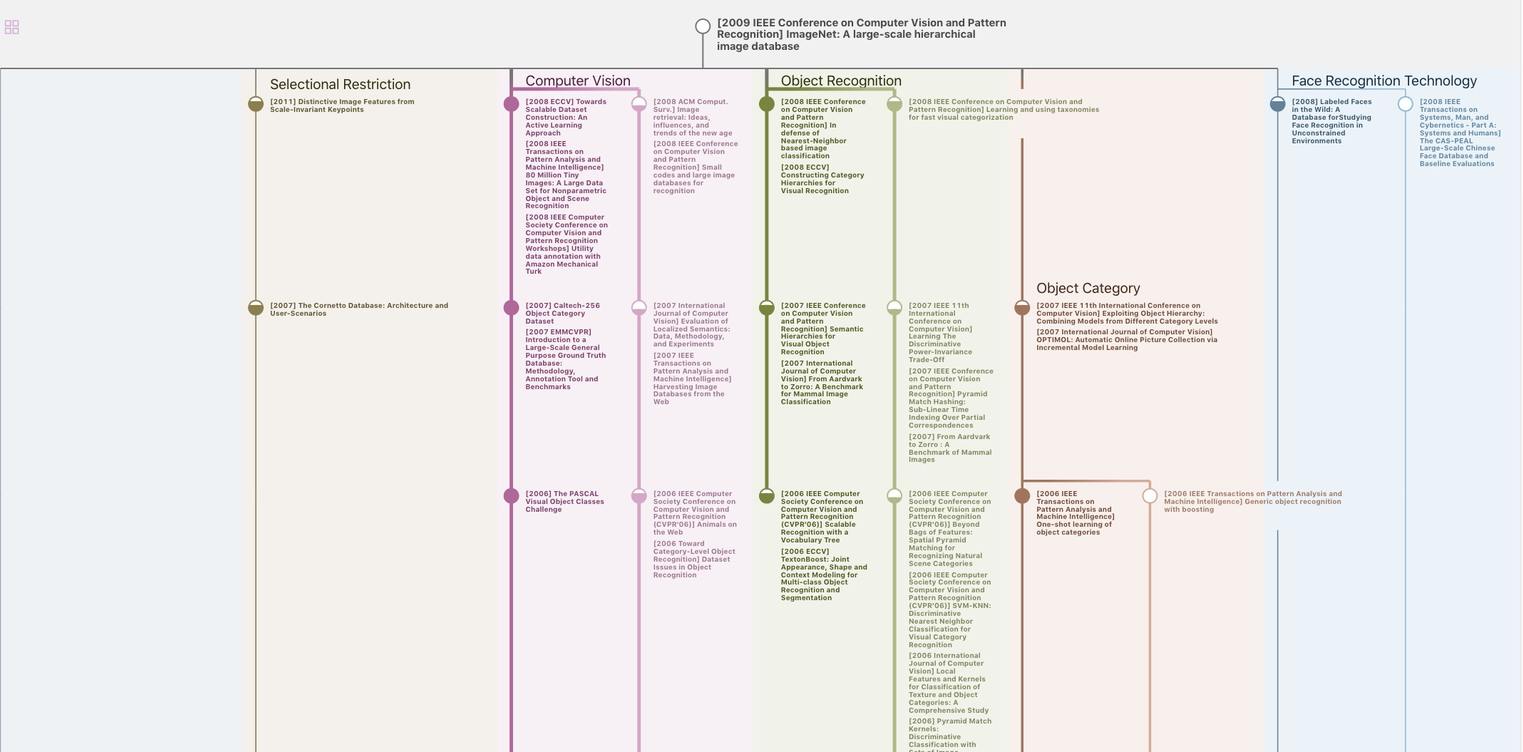
生成溯源树,研究论文发展脉络
Chat Paper
正在生成论文摘要