Enhancing generalized anxiety disorder diagnosis precision: MSTCNN model utilizing high-frequency EEG signals
FRONTIERS IN PSYCHIATRY(2023)
摘要
Generalized Anxiety Disorder (GAD) is a prevalent mental disorder on the rise in modern society. It is crucial to achieve precise diagnosis of GAD for improving the treatments and averting exacerbation. Although a growing number of researchers beginning to explore the deep learning algorithms for detecting mental disorders, there is a dearth of reports concerning precise GAD diagnosis. This study proposes a multi-scale spatial-temporal local sequential and global parallel convolutional model, named MSTCNN, which designed to achieve highly accurate GAD diagnosis using high-frequency electroencephalogram (EEG) signals. To this end, 10-min resting EEG data were collected from 45 GAD patients and 36 healthy controls (HC). Various frequency bands were extracted from the EEG data as the inputs of the MSTCNN. The results demonstrate that the proposed MSTCNN, combined with the attention mechanism of Squeeze-and-Excitation Networks, achieves outstanding classification performance for GAD detection, with an accuracy of 99.48% within the 4-30 Hz EEG data, which is competitively related to state-of-art methods in terms of GAD classification. Furthermore, our research unveils an intriguing revelation regarding the pivotal role of high-frequency band in GAD diagnosis. As the frequency band increases, diagnostic accuracy improves. Notably, high-frequency EEG data ranging from 10-30 Hz exhibited an accuracy rate of 99.47%, paralleling the performance of the broader 4-30 Hz band. In summary, these findings move a step forward towards the practical application of automatic diagnosis of GAD and provide basic theory and technical support for the development of future clinical diagnosis system.
更多查看译文
关键词
generalized anxiety disorder (GAD),electroencephalogram (EEG),convolutional neural network (CNN),attention mechanisms,deep learning
AI 理解论文
溯源树
样例
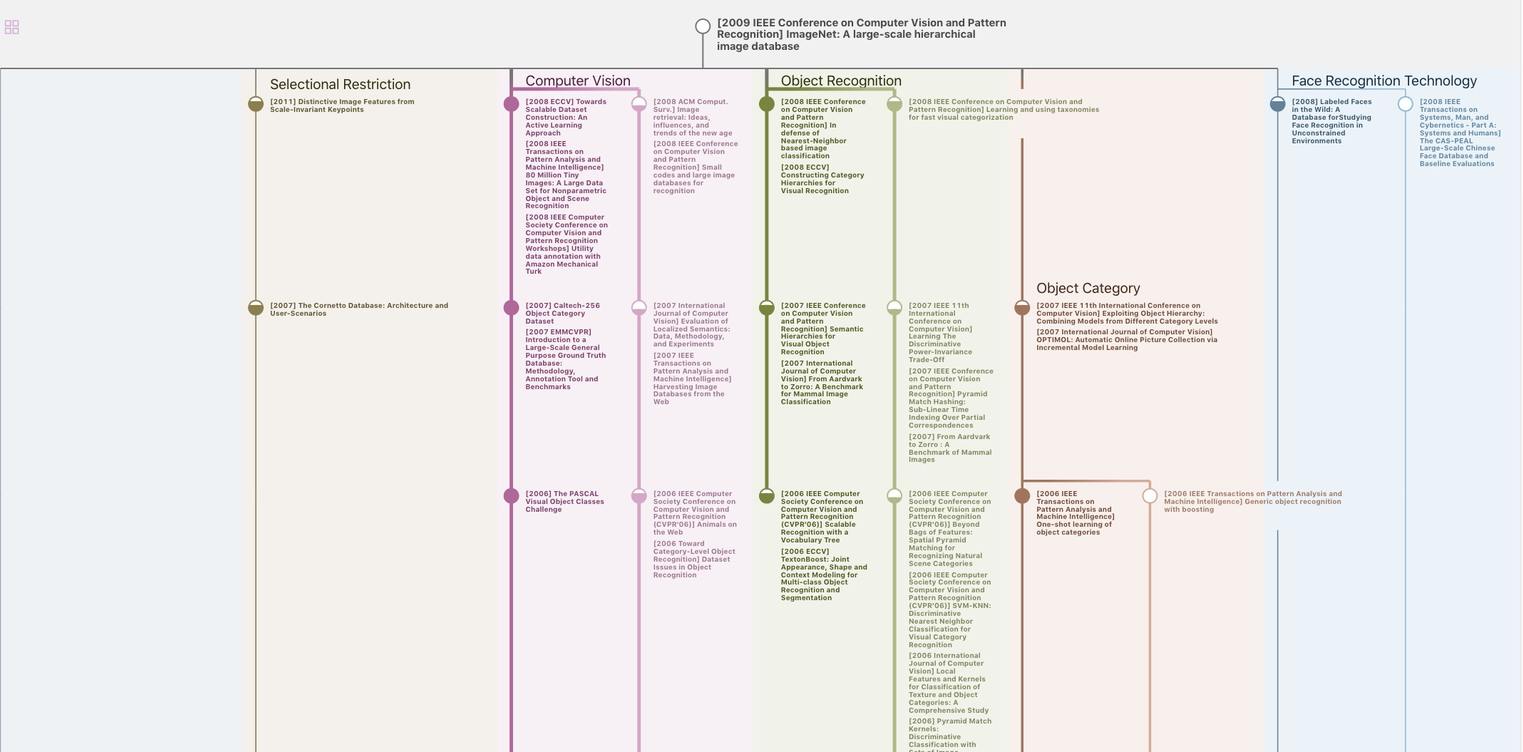
生成溯源树,研究论文发展脉络
Chat Paper
正在生成论文摘要