Enhancing cervical cancer diagnosis: Integrated attention-transformer system with weakly supervised learning
Image and Vision Computing(2024)
Abstract
Cervical cancer screening through cytopathological images poses a significant challenge due to the intricate nature of cancer cells, often resulting in high misdiagnosis rates. This study presents the Integrated Attention-Transformer System (IATS), a pioneering framework designed to enhance the precision and efficiency of cervical cancer cell image analysis, surpassing the capabilities of existing deep learning models. Instead of relying solely on convolutional neural networks (CNNs), IATS leverages the power of transformers, a recently emerged architecture, to holistically capture both global and local features within the images. It employs a multi-pronged approach: Vision Transformer (ViT) module captures the overall spatial context and interactions between cells, providing a crucial understanding of potential cancer patterns. Token-to-token module zooms in on individual cells, meticulously examining subtle malignant features that might be missed by CNNs. SeNet integration with ResNet101 and DenseNet169 refines feature extraction by dynamically analyzing the importance of different features captured by these popular deep learning architectures. SeNet acts like a skilled analyst, prioritizing the most informative features for accurate cancer cell identification. Weighted voting combines the insights from each module, leading to robust and accurate identification, minimizing misdiagnosis risk. The proposed framework achieves an impressive accuracy of 98.44% on Mendeley dataset and 95.88% on SIPaKMeD dataset, outperforming 25 deep learning models, which included Convolutional Neural Network (CNN) and Vision Transformer (VT) models. These results reveal a 2.5% accuracy improvement compared to the best-performing CNN model on the Mendeley dataset. This significant advancement holds the potential to revolutionize cervical cancer screening by substantially reducing misdiagnosis rates and improving patient outcomes. While this study focuses on model performance, future work will explore its computational efficiency and real-world clinical integration to ensure its broader impact on patient care.
MoreTranslated text
Key words
Cervical cancer,ViT,Weakly supervised learning,Vision transformer
AI Read Science
Must-Reading Tree
Example
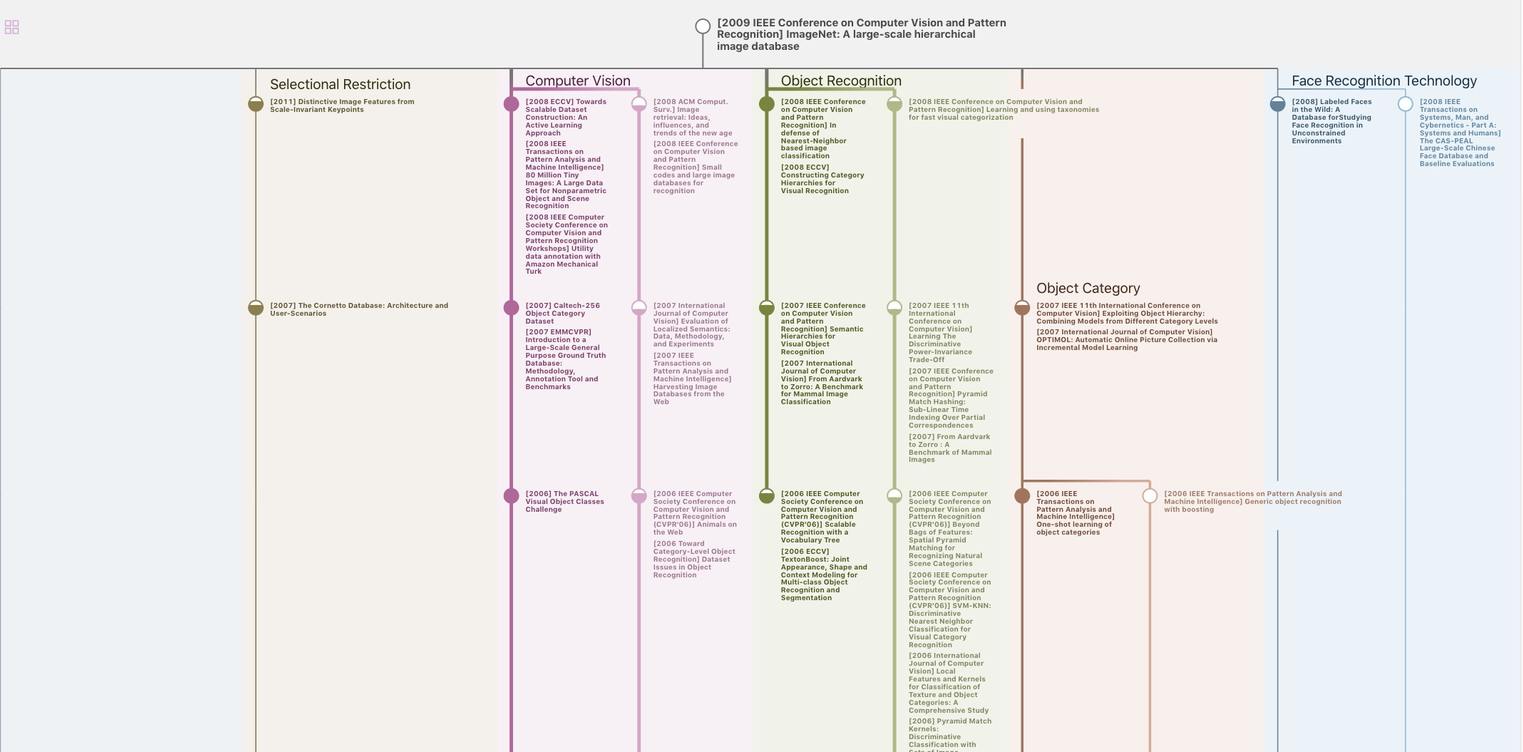
Generate MRT to find the research sequence of this paper
Chat Paper
Summary is being generated by the instructions you defined