Multi-Action Knee Contact Force Prediction by Domain Adaptation
IEEE TRANSACTIONS ON NEURAL SYSTEMS AND REHABILITATION ENGINEERING(2024)
摘要
Most recent musculoskeletal dynamics estimation methods are designed for predefined actions, such as gait, and don't generalize to various tasks. In this work, we address the problem of estimating internal biomechanical forces during more than one actions by introducing unsupervised domain adaptation into a deep learning model. More specifically, we developed a Bidirectional Long Short-Term Memory network for knee contact force prediction, enhanced with correlation alignment layers, in order to minimize the domain shift between kinematic data from different actions. Furthermore, we used the novel Neural State Machine (NSM) as a simulation platform to test and visualize our model predictions in a wide range of trajectories adapted to different 3D scene geometries in real-time. We conducted multiple experiments, including comparison with previous models, model alignment across action classes and real-to-synthetic data alignment. The results showed that the proposed deep learning architecture with domain adaptation performs better than the benchmark in terms of NRMSE and t-test. Overall, our method is capable of predicting knee contact forces for more than one action classes using a single architecture and thereby opens the path for estimating internal forces for intermediate actions, while the knowledge of the hidden state of motion may be used to support personalized rehabilitation. Moreover, our model can be easily integrated into any human motion simulation environment, which shows its potential in enabling biomechanical analysis in an automated and computationally efficient way.
更多查看译文
关键词
Deep learning,domain adaptation,force prediction,BiLSTM,neural state machine,musculoskeletal modeling
AI 理解论文
溯源树
样例
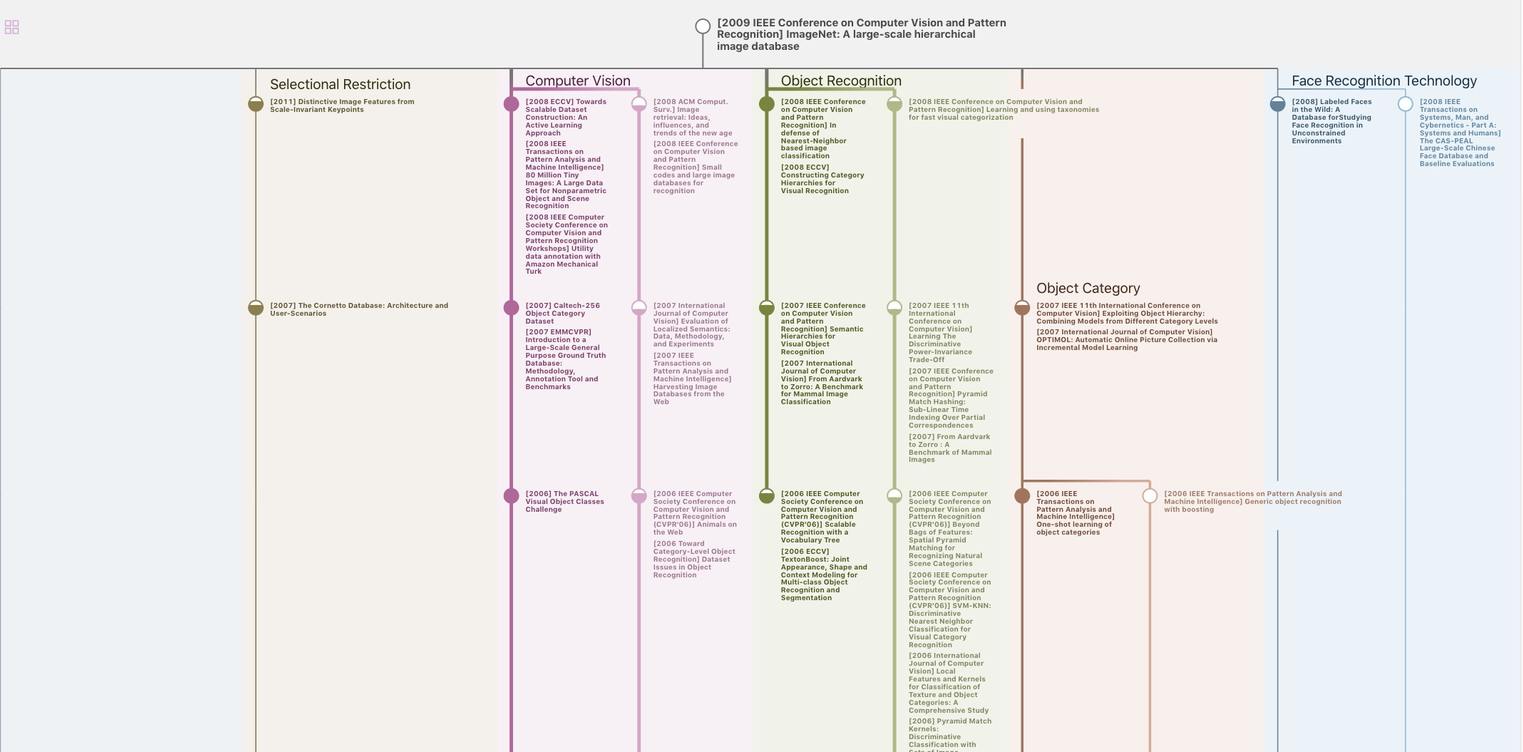
生成溯源树,研究论文发展脉络
Chat Paper
正在生成论文摘要