An efficient feature selection and explainable classification method for EEG-based seizure detection
JOURNAL OF INFORMATION SECURITY AND APPLICATIONS(2024)
摘要
Epilepsy is a prevalent neurological disorder that poses life-threatening emergencies. Early electroencephalogram (EEG) seizure detection can mitigate the risks and aid in the treatment of patients with epilepsy. EEG based epileptic seizure (ES) detection has significant applications in epilepsy treatment and medical diagnosis. Therefore, this paper presents an innovative framework for efficient ES detection, providing coefficient and distance correlation feature selection algorithms, a Bagged Tree-based classifer (BTBC), and Explainable Artificial Intelligence (XAI). Initially, the Butterworth filter is employed to eliminate various artifacts, and the discrete wavelet transform (DWT) is used to decompose the EEG signals and extract various eigenvalue features of the statistical time domain (STD) as linear and Fractal dimension-based non-linear (FD-NL). The optimal features are then identified through correlation coefficients with P-value and distance correlation analysis.These features are subsequently utilized by the Bagged Tree-based classifer (BTBC). The proposed model provides best performance in mitigating overfitting issues and improves the average accuracy by 2% using (CD, E), (AB, CD, E), and (A, B) experimental types as compared to other machine learning (ML) models using well-known Bonn and UCI-EEG benchmark datasets. Finally, SHapley additive exPlanation (SHAP) was used to interpret and explain the decision-making process of the proposed model. The results highlight the framework's capability to accurately classify ES, thereby improving the diagnosis process in patients with brain dysfunctions.
更多查看译文
关键词
Electroencephalogram,Machine learning,Coefficient correlation,Distance correlation,Biomedical signals,Explainable artificial intelligence
AI 理解论文
溯源树
样例
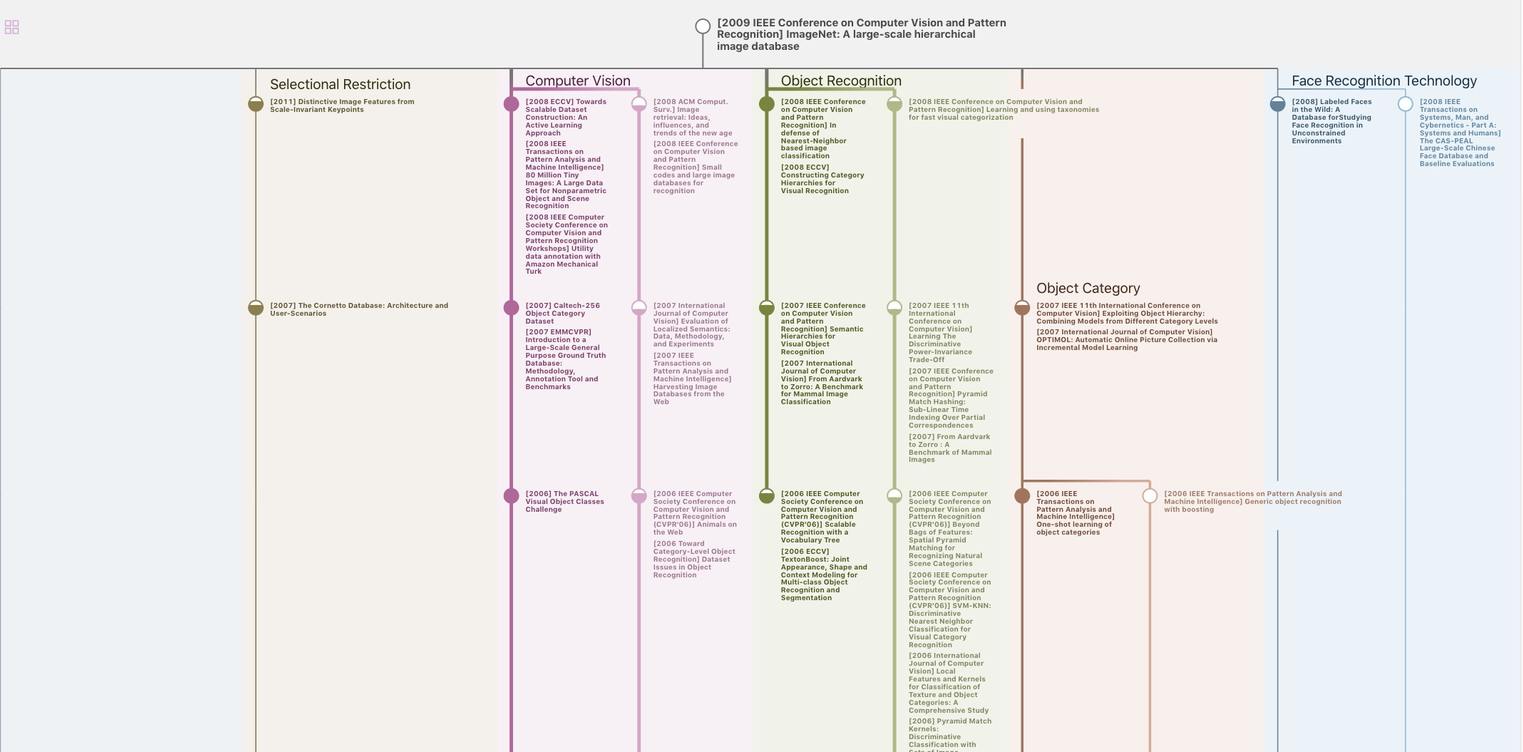
生成溯源树,研究论文发展脉络
Chat Paper
正在生成论文摘要