Predicting the Trend of SARS-CoV-2 Mutation Frequencies Using Historical Data
biorxiv(2023)
摘要
As the SARS-CoV-2 virus rapidly evolves, predicting the trajectory of viral variations has become a critical yet complex task. A deep understanding of future mutation patterns, in particular the mutations that will prevail in the near future, is vital in steering diagnostics, therapeutics, and vaccine strategies in the coming months.
In this study, we developed a model to forecast future SARS-CoV-2 mutation surges in real-time, using historical mutation frequency data from the USA. To improve upon the accuracy of traditional time-series models, we transformed the prediction problem into a supervised learning framework using a sliding window approach. This involved breaking the time series of mutation frequencies into very short segments. Considering the time-dependent nature of the data, we focused on modeling the first-order derivative of the mutation frequency. We predicted the final derivative in each segment based on the preceding derivatives, employing various machine learning methods, including random forest, XGBoost, support vector machine, and neural network models, in this supervised learning setting. Empowered by the novel transformation strategy and the high capacity of machine learning models, we witnessed low prediction error that is confined within 0.1% and 1% when making predictions for future 30 and 80 days respectively. In addition, the method also led to a notable increase in prediction accuracy compared to traditional time-series models, as evidenced by lower MAE, and MSE for predictions made within different time horizons. To further assess the method’s effectiveness and robustness in predicting mutation patterns for unforeseen mutations, we categorized all mutations into three major patterns. The model demonstrated its robustness by accurately predicting unseen mutation patterns when training on data from two pattern categories while testing on the third pattern category, showcasing its potential in forecasting a variety of mutation trajectories.
To enhance accessibility and utility, we built our methodology into an R-shiny app ( ), a tool with potential applicability in studying other infectious diseases, thus extending its relevance beyond the current pandemic.
### Competing Interest Statement
The authors have declared no competing interest.
更多查看译文
AI 理解论文
溯源树
样例
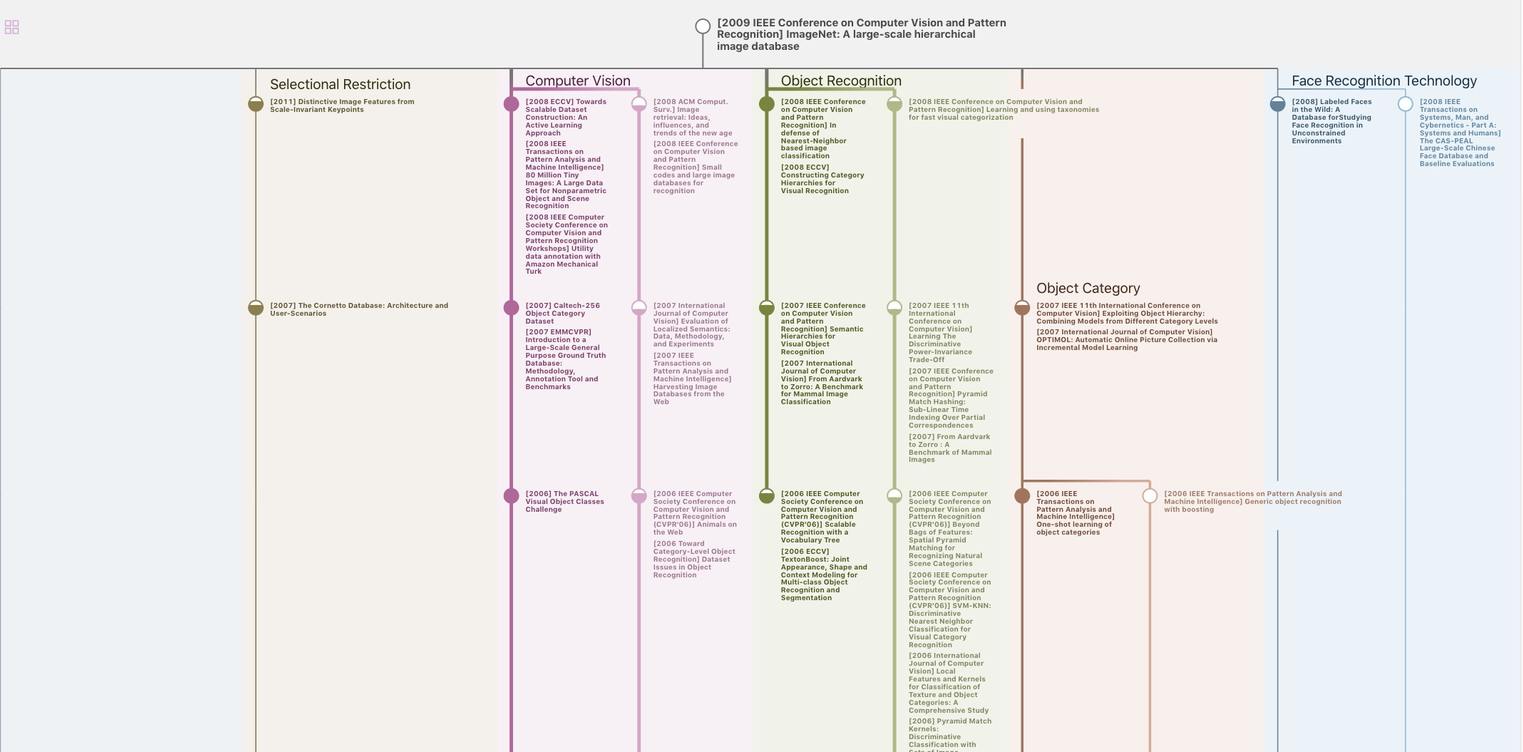
生成溯源树,研究论文发展脉络
Chat Paper
正在生成论文摘要