Enhancing Crop Health Monitoring: A Hybrid CNN-RF Model for Early Detection and Fine-Grained Classification of Cotton Bacterial Blight
2023 4th IEEE Global Conference for Advancement in Technology (GCAT)(2023)
Abstract
The destructive disease known as cotton leaf bacterial blight is a major danger to cotton crops all over the world. To effectively manage crops and maintain yields, early and precise diagnosis of disease severity levels is essential. To identify and categorize cotton bacterial blight illness, we suggest a hybrid strategy in this study that combines a Convolutional Neural Network (CNN) with a Random Forest (RF) model. We used a dataset of 10,000 photos of cotton leaves showing various stages of illness to train our model. The robustness of the model was improved by doing extensive data preparation, which included shrinking, normalizing, class balancing, and augmenting the data. CNN layers were used for feature extraction, while an RF classifier was used for classification in the hybrid model architecture. Our model has an overall accuracy of 94.58% on a different test set. This degree of precision suggests that the hybrid method is capable of properly differentiating between disease severities caused by bacterial blight. Our findings improve upon existing methods for detecting cotton leaf bacterial blight, which is a significant contribution to the area of agricultural disease identification. The combination of a CNN with an RF model provides a powerful tool to aid in crop management practices, allowing for prompt intervention and tailored treatments to lessen the impact of the illness on cotton harvests.
MoreTranslated text
Key words
Bacterial Blight Disease,Convolutional neural network,Random Forest
AI Read Science
Must-Reading Tree
Example
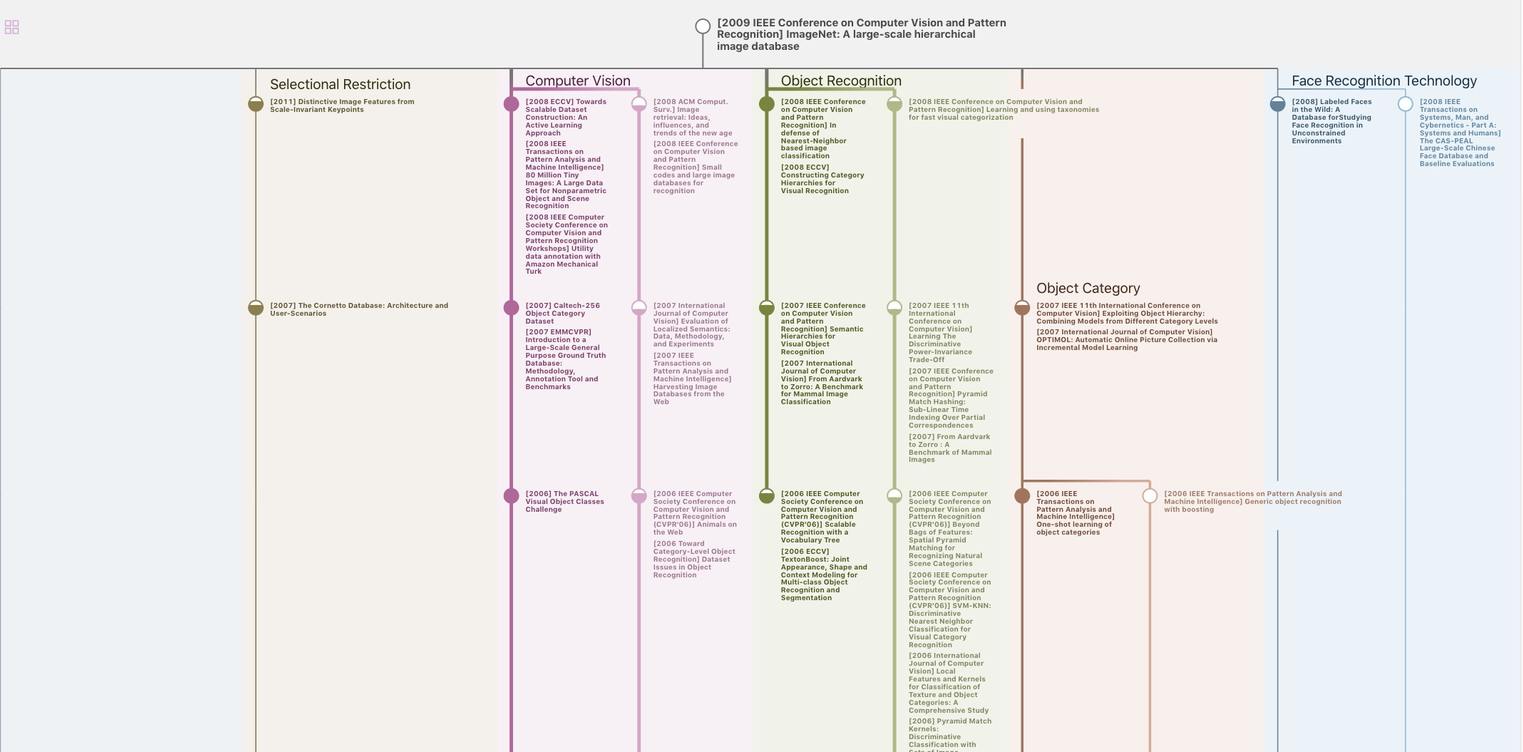
Generate MRT to find the research sequence of this paper
Chat Paper
Summary is being generated by the instructions you defined