FireSpot: A Database for Smoke Detection in Early-stage Wildfires
2023 18TH INTERNATIONAL JOINT SYMPOSIUM ON ARTIFICIAL INTELLIGENCE AND NATURAL LANGUAGE PROCESSING, ISAI-NLP(2023)
摘要
During a dry season in northern Thailand, typically between November and May, wildfires, either natural or manmade, annually occur and pose severe air pollution, particularly concerning PM2.5 particles. A no-burning period policy has been deployed to prevent and mitigate the effect. However, relying solely on traditional monitoring methods using forest fire lookout towers operated by human staff is insufficient due to limited human resources. Therefore, this project aims to develop a monitoring system based on visual imagery to detect smoke in early-stage wildfires. Based on our survey, no available database is suitable for practically implementing a smoke detection model for an early-stage forest fire warning system. Thus, we construct it by ourselves and report the detail of its construction in this study. We also developed a smoke detection model based on YOLOv5 to demonstrate its practical use. The model's performance evaluation shows that the accuracy is 93.88%, the precision is 97.40%, the recall is 93.75%, and the F1-score is 95.54%, which are promising results initially.
更多查看译文
关键词
Smoke detection,Forest fire detection,Dataset construction,YOLOv5
AI 理解论文
溯源树
样例
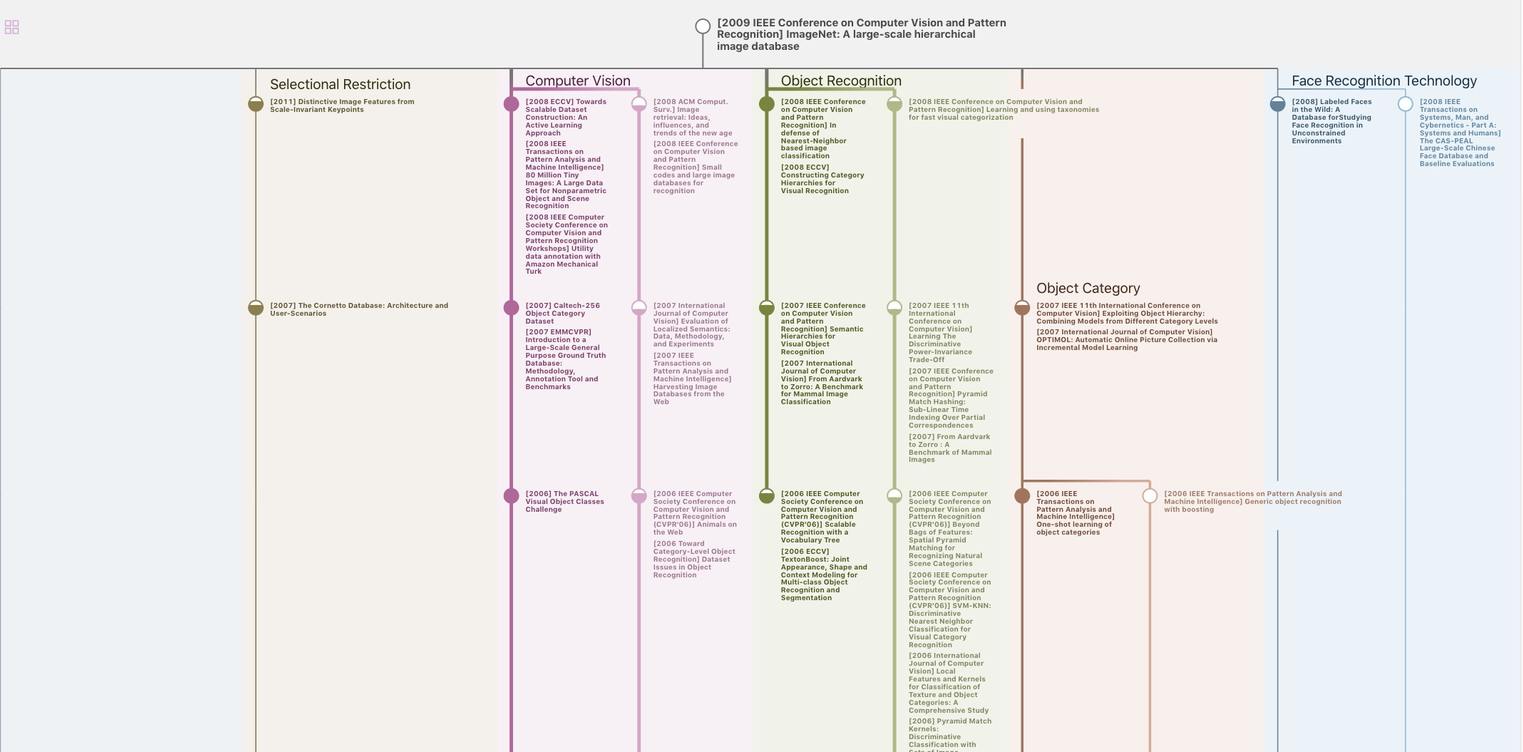
生成溯源树,研究论文发展脉络
Chat Paper
正在生成论文摘要