CausalABSC: Causal Inference for Aspect Debiasing in Aspect-Based Sentiment Classification
IEEE-ACM TRANSACTIONS ON AUDIO SPEECH AND LANGUAGE PROCESSING(2024)
摘要
As the primary subtask of sentiment analysis, aspect-based sentiment classification (ABSC) aims to predict the sentiment polarity for a given aspect. While recent deep neural models for ABSC have shown good performance, their robustness is limited due to their reliance on spurious correlations between aspects and sentiment. Specifically, most existing models tend to assign the most frequent sentiment label to a certain aspect in different sentences, or assume different aspects in the same sentence to have the same sentiment polarity, which is vulnerable due to the aspect biases. In this paper, we propose a causal graph to identify and analyze the causal relationships among treatment variables (e.g., aspect, sentence), intermediate variables (e.g., aspect-aware content), and outcome variables (e.g., sentiment polarity) for ABSC. To address the issue of spurious relationships that mislead sentiment polarity prediction, we introduce a novel causal inference framework called CausalABSC. CausalABSC is model agnostic, allowing integration into existing methods. We conduct extensive experiments on five benchmark datasets, which demonstrate the state-of-the-art performance of CausalABSC and its effectiveness in debiasing.
更多查看译文
关键词
Aspect-based sentiment analysis,causal inference,bias
AI 理解论文
溯源树
样例
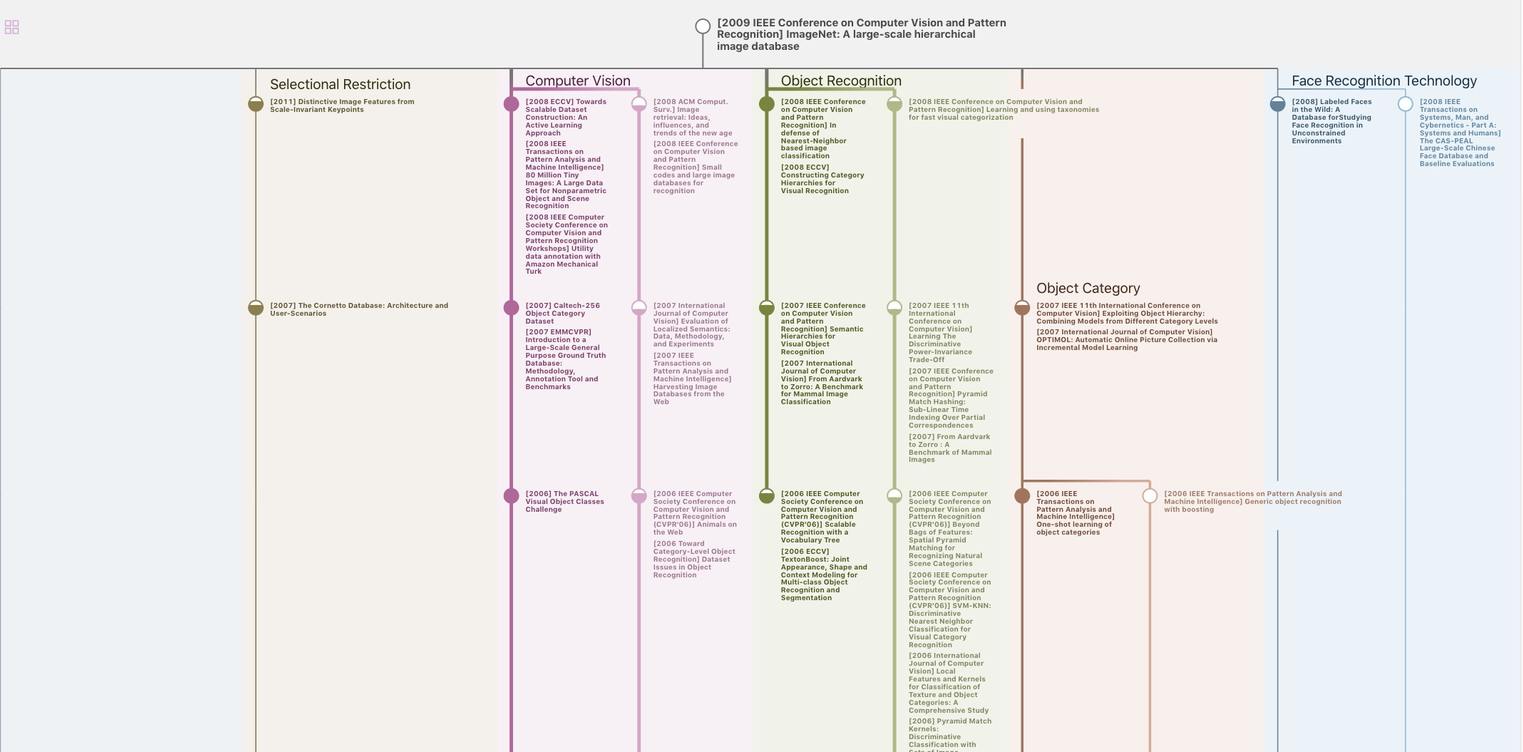
生成溯源树,研究论文发展脉络
Chat Paper
正在生成论文摘要