Robotic Arm Trajectory Planning Method Using Deep Deterministic Policy Gradient With Hierarchical Memory Structure
IEEE ACCESS(2023)
摘要
Traditional robotic arm path planning methods are mainly carried out in the tool center point operation space, and frequently solve inverse kinematics problems, thus consuming a large number of computational resources. In contrast, the use of positive kinematics for planning in joint space not only enhances efficiency, but also provides analytic solutions with higher accuracy. In order to better cover the environmental state space, this paper adopts the full-preserving experience preservation approach. In order to realize fast and efficient sampling of high reward value experience, this study constructs an innovative hierarchical memory structure and eliminates the overfitting phenomenon that may be caused by biased sampling through the Bias-Free strategy. Experimentally validated in the continuous path planning task of a textile robot arm, the proposed hierarchical memory deep deterministic gradient strategy method (HM-DDPG) demonstrates excellent performance and practicality in the textile robot arm path planning problem. This method offers an efficient and robust solution for handling complex tasks with temporal dependencies, paving the way for future innovations and applications in industrial fields such as textiles.
更多查看译文
关键词
Continuous control task,deep deterministic policy gradient,path planning,positive kinematics,hierarchical memory
AI 理解论文
溯源树
样例
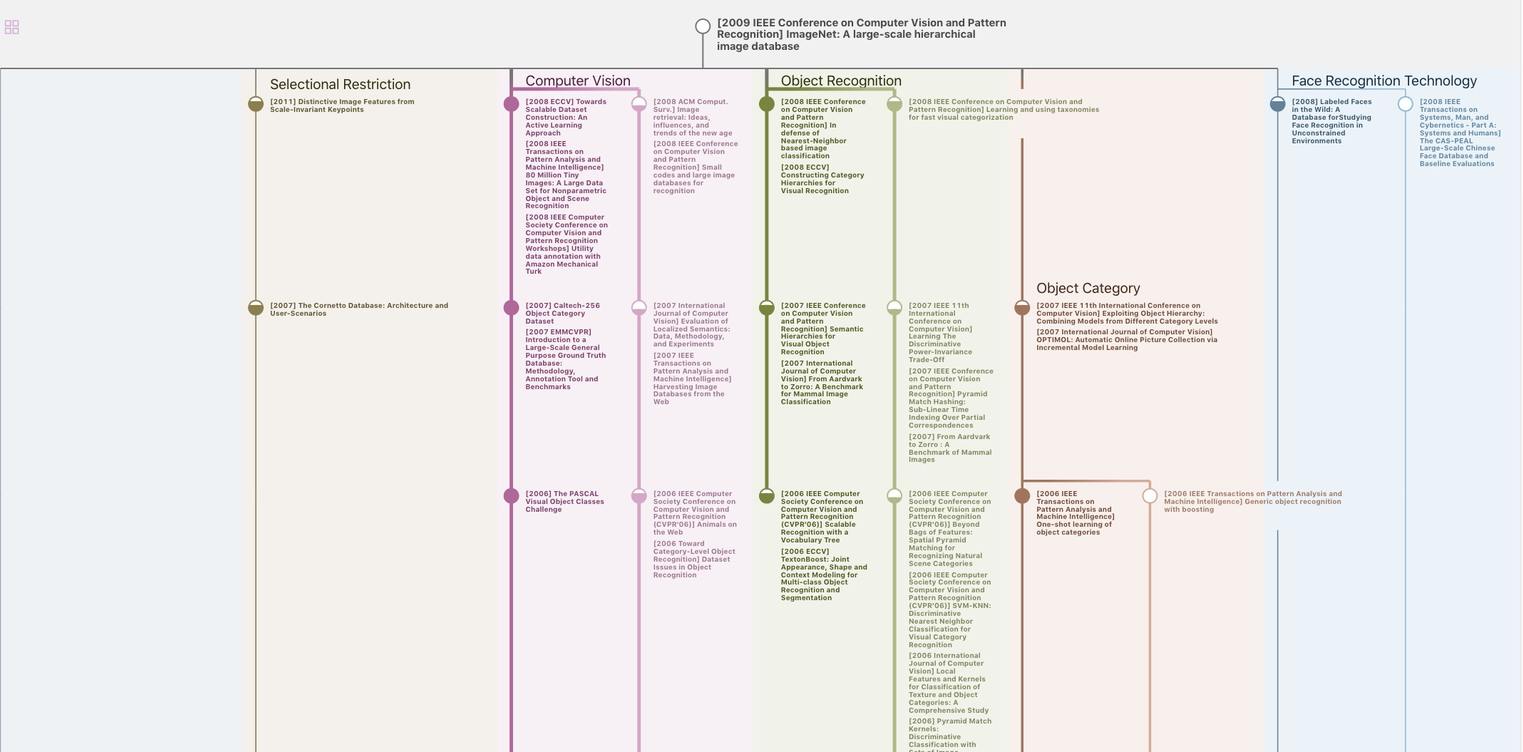
生成溯源树,研究论文发展脉络
Chat Paper
正在生成论文摘要